Aggregation of Markov chains: an analysis of deterministic annealing based methods
CDC(2014)
摘要
We develop a method for aggregating large Markov chains into smaller representative Markov chains, where Markov chains are viewed as weighted directed graphs, and similar nodes (and edges) are aggregated using a deterministic annealing approach. The notions of representativeness of the aggregated graphs and similarity between nodes in graphs are based on a newly proposed metric that quantifies connectivity in the underlying graph. Namely, we develop notions of distance between subchains in Markov chains, and provide easily verifiable conditions that determine if a given Markov chain is nearly decomposable, that is, conditions for which the deterministic annealing approach can be used to identify subchains with high probability. We show that the aggregated Markov chain preserves certain dynamics of the original chain. In particular we provide explicit bounds on the ℓ1 norm of the error between the aggregated stationary distribution of the original Markov chain and the stationary distribution of the aggregated Markov chain, which extends on longstanding foundational results (Simon and Ando, 1961).
更多查看译文
关键词
optimisation,statistical distributions,original markov chain stationary distribution aggregation,similarity,deterministic annealing based methods,ℓ1 norm,aggregated markov chain stationary distribution aggregation,aggregated graphs,similar node aggregation,weighted directed graphs,distance notion development,directed graphs,deterministic algorithms,markov processes,subchain identification
AI 理解论文
溯源树
样例
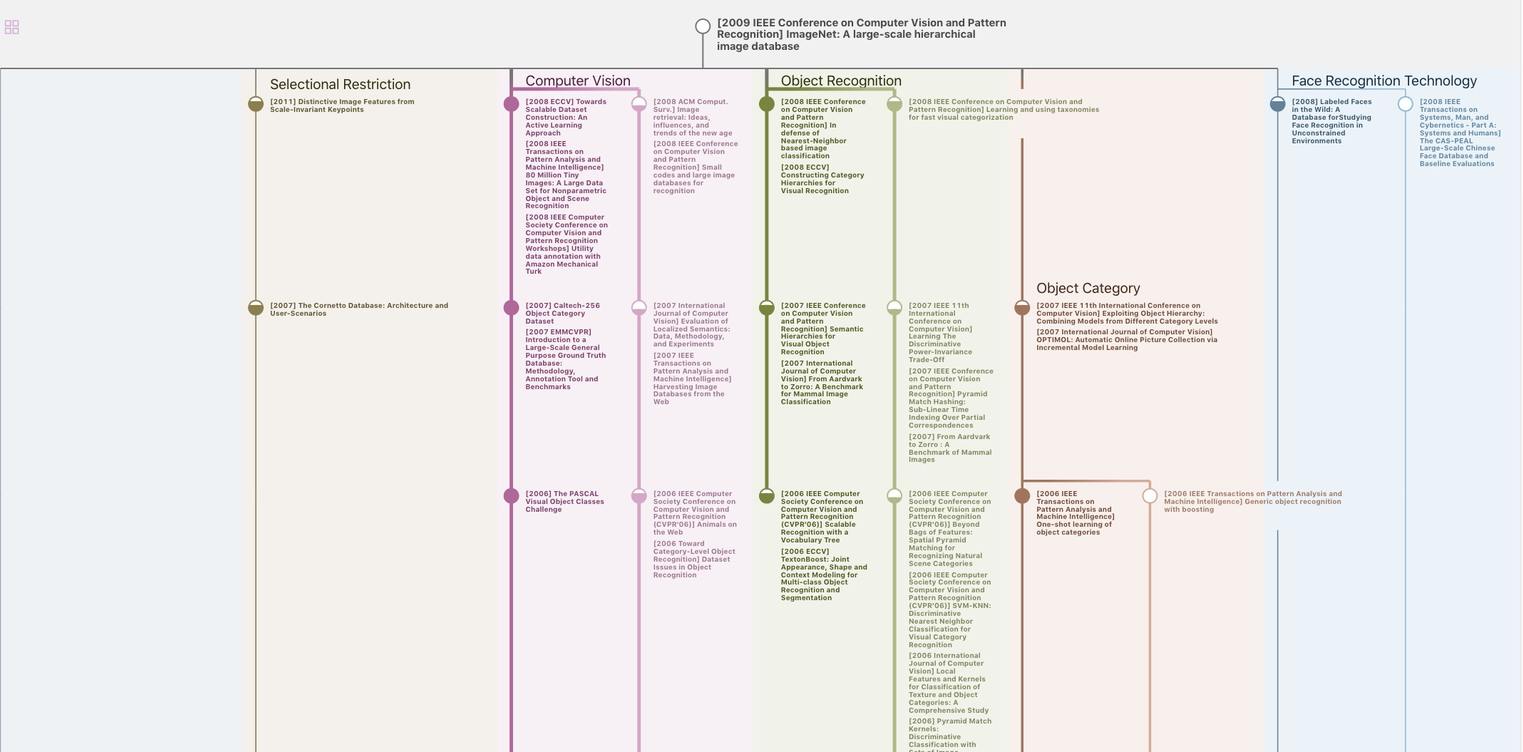
生成溯源树,研究论文发展脉络
Chat Paper
正在生成论文摘要