Speech feature denoising and dereverberation via deep autoencoders for noisy reverberant speech recognition
ICASSP(2014)
摘要
Denoising autoencoders (DAs) have shown success in generating robust features for images, but there has been limited work in applying DAs for speech. In this paper we present a deep denoising autoencoder (DDA) framework that can produce robust speech features for noisy reverberant speech recognition. The DDA is first pre-trained as restricted Boltzmann machines (RBMs) in an unsupervised fashion. Then it is unrolled to autoencoders, and fine-tuned by corresponding clean speech features to learn a nonlinear mapping from noisy to clean features. Acoustic models are re-trained using the reconstructed features from the DDA, and speech recognition is performed. The proposed approach is evaluated on the CHiME-WSJ0 corpus, and shows a 16-25% absolute improvement on the recognition accuracy under various SNRs.
更多查看译文
关键词
recognition accuracy,signal denoising,deep neural network,boltzmann machines,speech recognition,denoising autoencoder,learning (artificial intelligence),acoustic models,reverberation,restricted boltzmann machines,speech feature denoising,noisy reverberant speech recognition,chime-wsj0 corpus,speech coding,feature denoising,robust speech recognition,deep denoising autoencoders,speech feature dereverberation,unsupervised learning,noise measurement,decoding,robustness,noise reduction,learning artificial intelligence,hidden markov models,speech
AI 理解论文
溯源树
样例
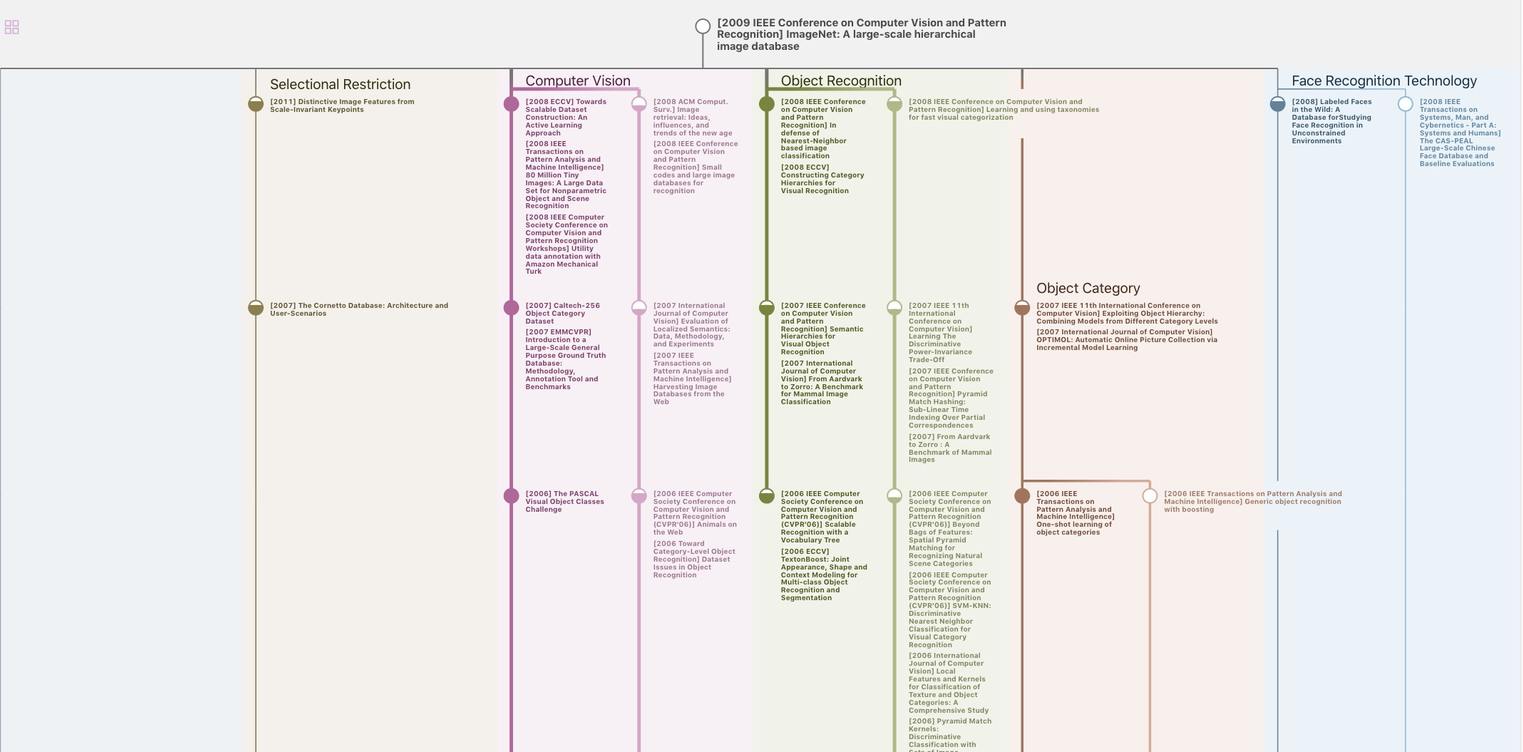
生成溯源树,研究论文发展脉络
Chat Paper
正在生成论文摘要