Significant Route Discovery: A Summary Of Results
GEOGRAPHIC INFORMATION SCIENCE (GISCIENCE 2014)(2014)
摘要
Given a spatial network and a collection of activities (e.g., pedestrian fatality reports, crime reports), Significant Route Discovery (SRD) finds all shortest paths in the spatial network where the concentration of activities is unusually high (i.e., statistically significant). SRD is important for societal applications in transportation safety, public safety, or public health such as finding routes with significant concentrations of accidents, crimes, or diseases. SRD is challenging because 1) there are a potentially large number of candidate routes (similar to 10(16)) in a given dataset with millions of activities or road network nodes and 2) significance testing does not obey the monotonicity property. Previous work focused on finding circular areas of concentration, limiting its usefulness for finding significant linear routes on a network. SaTScan may miss many significant routes since a large fraction of the area bounded by circles for activities on a path will be empty. This paper proposes a novel algorithm for discovering statistically significant routes. To improve performance, the proposed algorithm features algorithmic refinements that prune unlikely paths and speeds up Monte Carlo simulation. We present a case study comparing the proposed statistically significant network-based analysis (i.e., shortest paths) to a statistically significant geometry-based analysis (e.g., circles) on pedestrian fatality data. Experimental results on real data show that the proposed algorithm, with our algorithmic refinements, yields substantial computational savings without reducing result quality.
更多查看译文
关键词
Likelihood Ratio, Short Path, Road Network, Active Node, Route Discovery
AI 理解论文
溯源树
样例
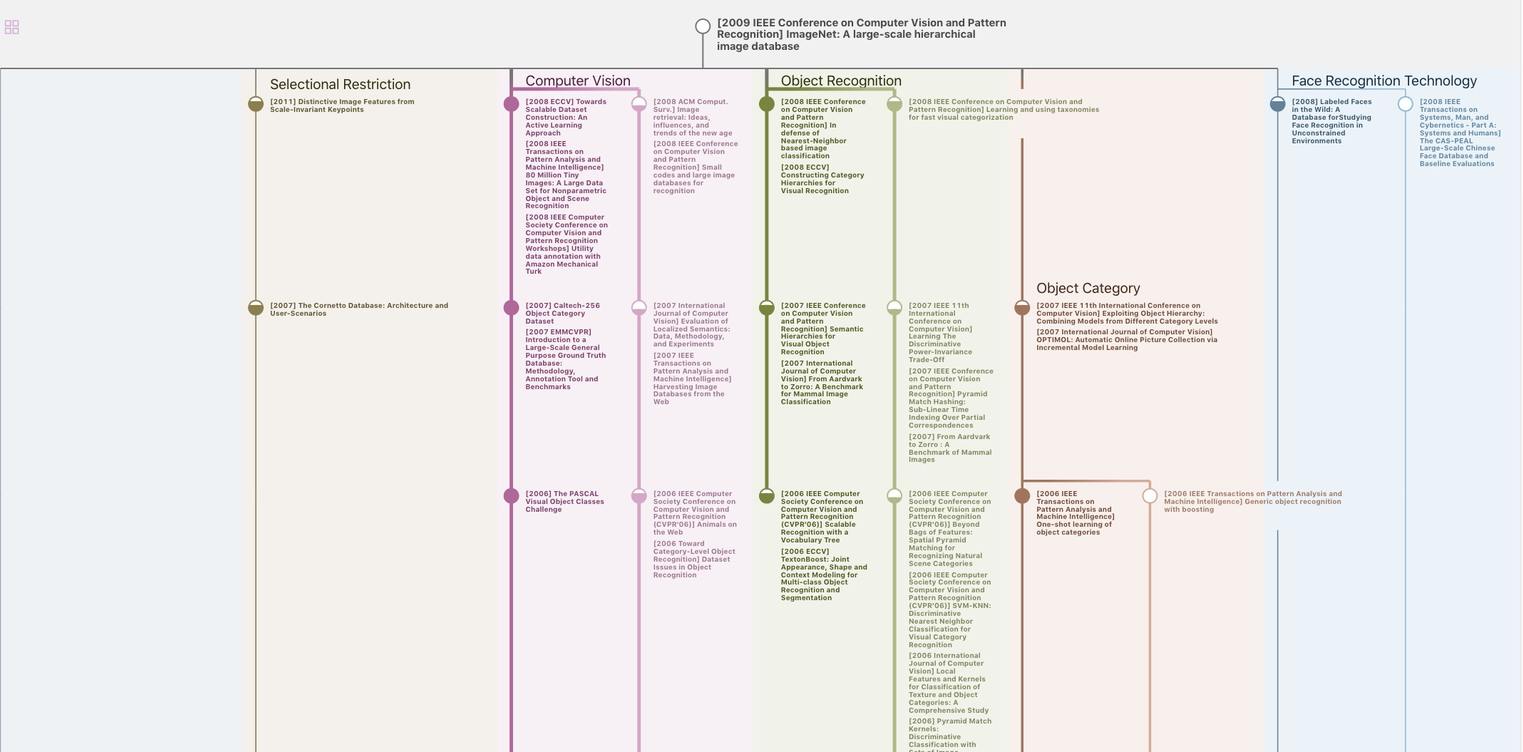
生成溯源树,研究论文发展脉络
Chat Paper
正在生成论文摘要