Enriching Visual Knowledge Bases via Object Discovery and Segmentation
CVPR(2014)
摘要
There have been some recent efforts to build visual knowledge bases from Internet images. But most of these approaches have focused on bounding box representation of objects. In this paper, we propose to enrich these knowledge bases by automatically discovering objects and their segmentations from noisy Internet images. Specifically, our approach combines the power of generative modeling for segmentation with the effectiveness of discriminative models for detection. The key idea behind our approach is to learn and exploit top-down segmentation priors based on visual subcategories. The strong priors learned from these visual subcategories are then combined with discriminatively trained detectors and bottom up cues to produce clean object segmentations. Our experimental results indicate state-of-the-art performance on the difficult dataset introduced by [29] Rubinstein et al. We have integrated our algorithm in NEIL for enriching its knowledge base [5]. As of 14th April 2014, NEIL has automatically generated approximately 500K segmentations using web data.
更多查看译文
关键词
bounding box representation,image representation,knowledge based systems,web data,image segmentation,object segmentation,neil,generative modeling,internet,internet images,visual subcategories,visual knowledge bases,top-down segmentation,discriminatively trained detectors,object discovery,detectors,semantics,visualization,noise measurement
AI 理解论文
溯源树
样例
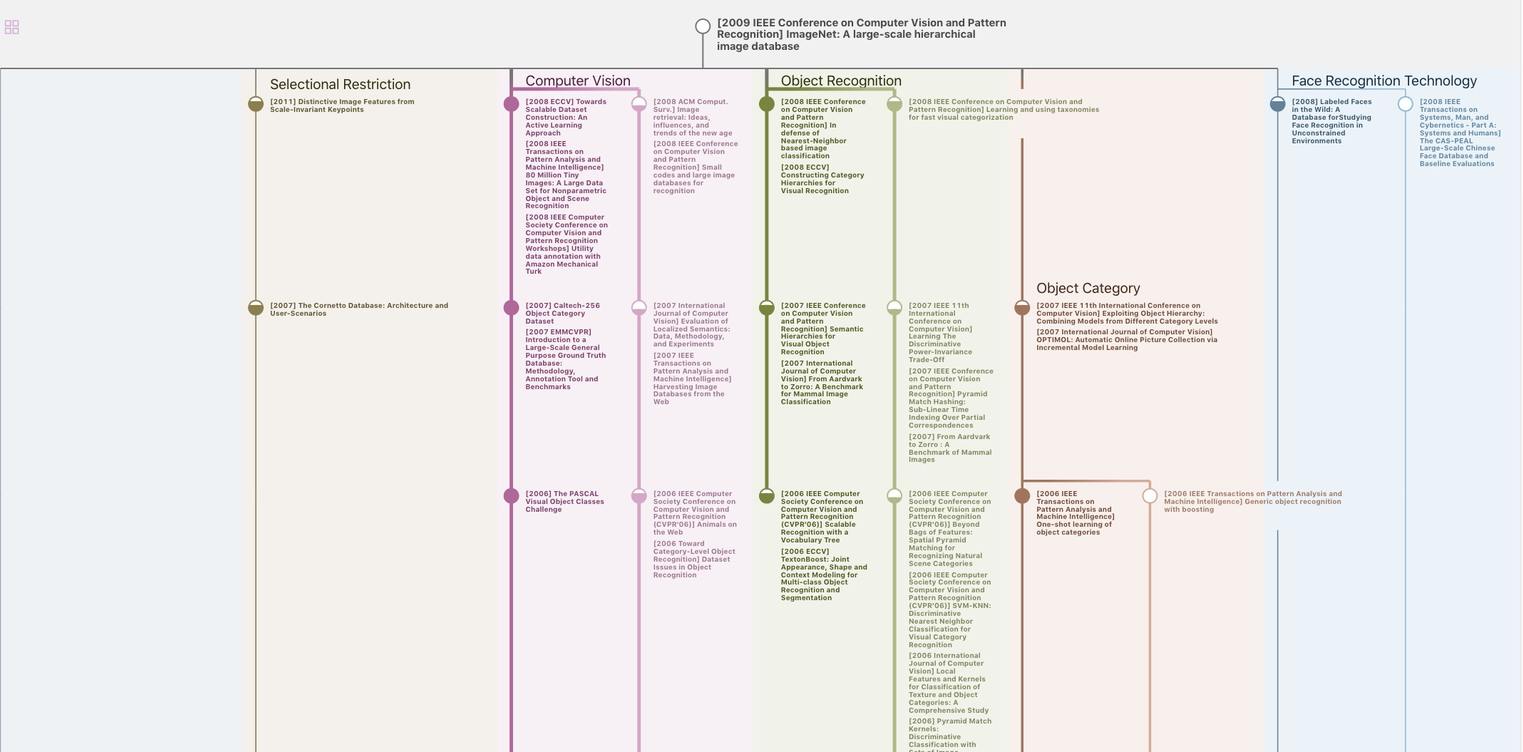
生成溯源树,研究论文发展脉络
Chat Paper
正在生成论文摘要