Similarity-Aware Patchwork Assembly for Depth Image Super-resolution
CVPR(2014)
摘要
This paper describes a patchwork assembly algorithm for depth image super-resolution. An input low resolution depth image is disassembled into parts by matching similar regions on a set of high resolution training images, and a super-resolution image is then assembled using these corresponding matched counterparts. We convert the super resolution problem into a Markov Random Field (MRF) labeling problem, and propose a unified formulation embedding (1) the consistency between the resolution enhanced image and the original input, (2) the similarity of disassembled parts with the corresponding regions on training images, (3) the depth smoothness in local neighborhoods, (4) the additional geometric constraints from self-similar structures in the scene, and (5) the boundary coincidence between the resolution enhanced depth image and an optional aligned high resolution intensity image. Experimental results on both synthetic and real-world data demonstrate that the proposed algorithm is capable of recovering high quality depth images with X4 resolution enhancement along each coordinate direction, and that it outperforms state-of-the-arts [14] in both qualitative and quantitative evaluations.
更多查看译文
关键词
mrf labeling problem,image matching,random processes,quantitative evaluation,assembly,image resolution,self-similarity,input low resolution depth image,similarity-aware patchwork assembly,markov processes,dpeth map super resolution,disassemble,resolution enhancement,qualitative evaluation,depth image super-resolution,similar region matching,self-similar structures,dpeth map super resolution, assembly, disassemble, self-similarity,image enhancement,geometric constraints,markov random field labeling problem,boundary coincidence,self similarity,databases
AI 理解论文
溯源树
样例
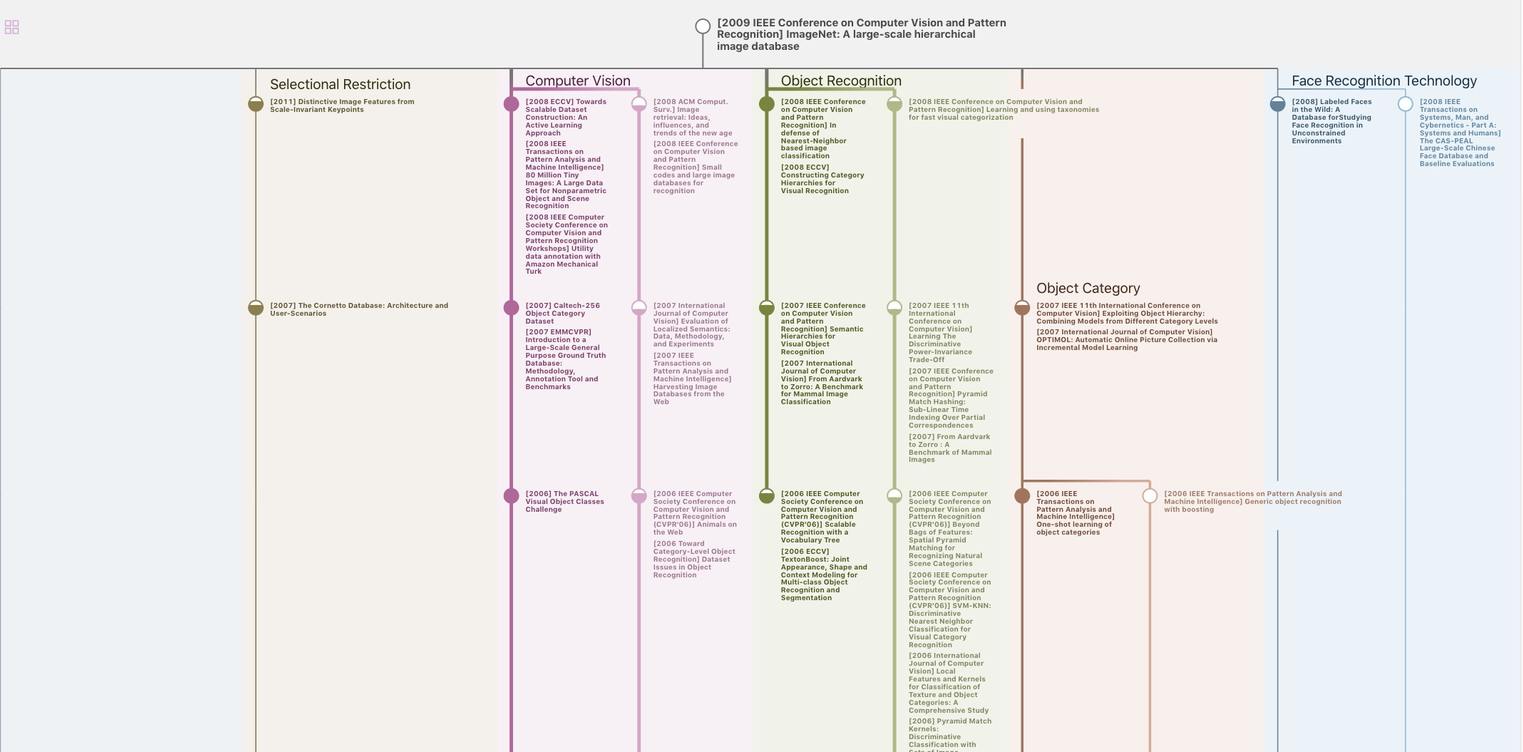
生成溯源树,研究论文发展脉络
Chat Paper
正在生成论文摘要