Structural Data De-Anonymization: Quantification, Practice, And Implications
CCS(2014)
摘要
In this paper, we study the quantification, practice, and implications of structural data (e.g., social data, mobility traces) De-Anonymization (DA). First, we address several open problems in structural data DA by quantifying perfect and (1 - epsilon)-perfect structural data DA, where epsilon is the error tolerated by a DA scheme. To the best of our knowledge, this is the first work on quantifying structural data DA under a general data model, which closes the gap between structural data DA practice and theory. Second, we conduct the first large-scale study on the de-anonymizability of 26 real world structural datasets, including Social Networks (SNs), Collaborations Networks, Communication Networks, Autonomous Systems, and Peer-to-Peer networks. We also quantitatively show the conditions for perfect and (1 - epsilon)-perfect DA of the 26 datasets. Third, following our quantification, we design a practical and novel single-phase cold start Optimization based DA (ODA) algorithm. Experimental analysis of ODA shows that about 77.7%-83.3% of the users in Gowalla (.2M users and 1M edges) and 86.9% - 95.5% of the users in Google+ (4.7M users and 90.8M edges) are de-anonymizable in different scenarios, which implies optimization based DA is implementable and powerful in practice. Finally, we discuss the implications of our DA quantification and ODA and provide some general suggestions for future secure data publishing.
更多查看译文
关键词
De-anonymization,structural data,quantification,evaluation,social networks,mobility traces
AI 理解论文
溯源树
样例
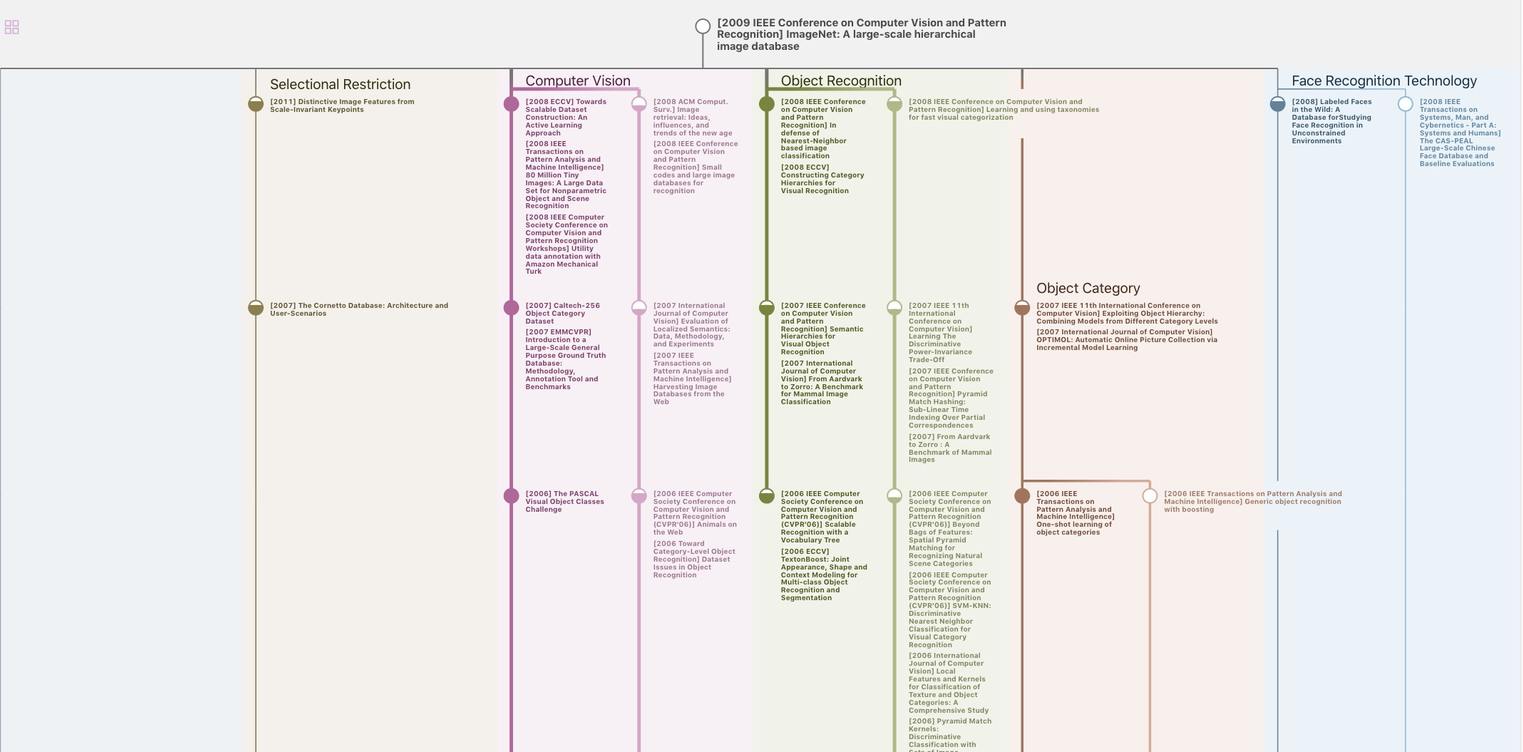
生成溯源树,研究论文发展脉络
Chat Paper
正在生成论文摘要