A classifier based approach to real-time fall detection using low-cost wearable sensors
SoCPaR(2014)
摘要
In this paper, we present a novel fall detection method using wearable sensors that are inexpensive and easy to deploy. A new, simple, yet effective feature extraction scheme is proposed, in which features are extracted from slices or quanta of sliding windows on the sensor's continuously acceleration data stream. Extracted features are used with a support vector machine model, which is trained to classify frames of data streams into containing falls or not. The proposed method is rigorously evaluated on a dataset containing 144 falls and other activities of daily living (which produces significant noise for fall detection). Results shows that falls could be detected with 91.9% precision and 94.4% recall. The experiments also demonstrate the superior performance of the proposed methods over three other fall detection methods.
更多查看译文
关键词
feature extraction scheme,pattern classification,classifier based approach,svm,low-cost wearable sensors,real-time fall detection,feature extraction,daily living,wireless sensor networks,support vector machine model,continuously acceleration data stream,fall detection,handicapped aids,wearable sensors,support vector machines,quantum computing,vectors,acceleration,accuracy,sensors
AI 理解论文
溯源树
样例
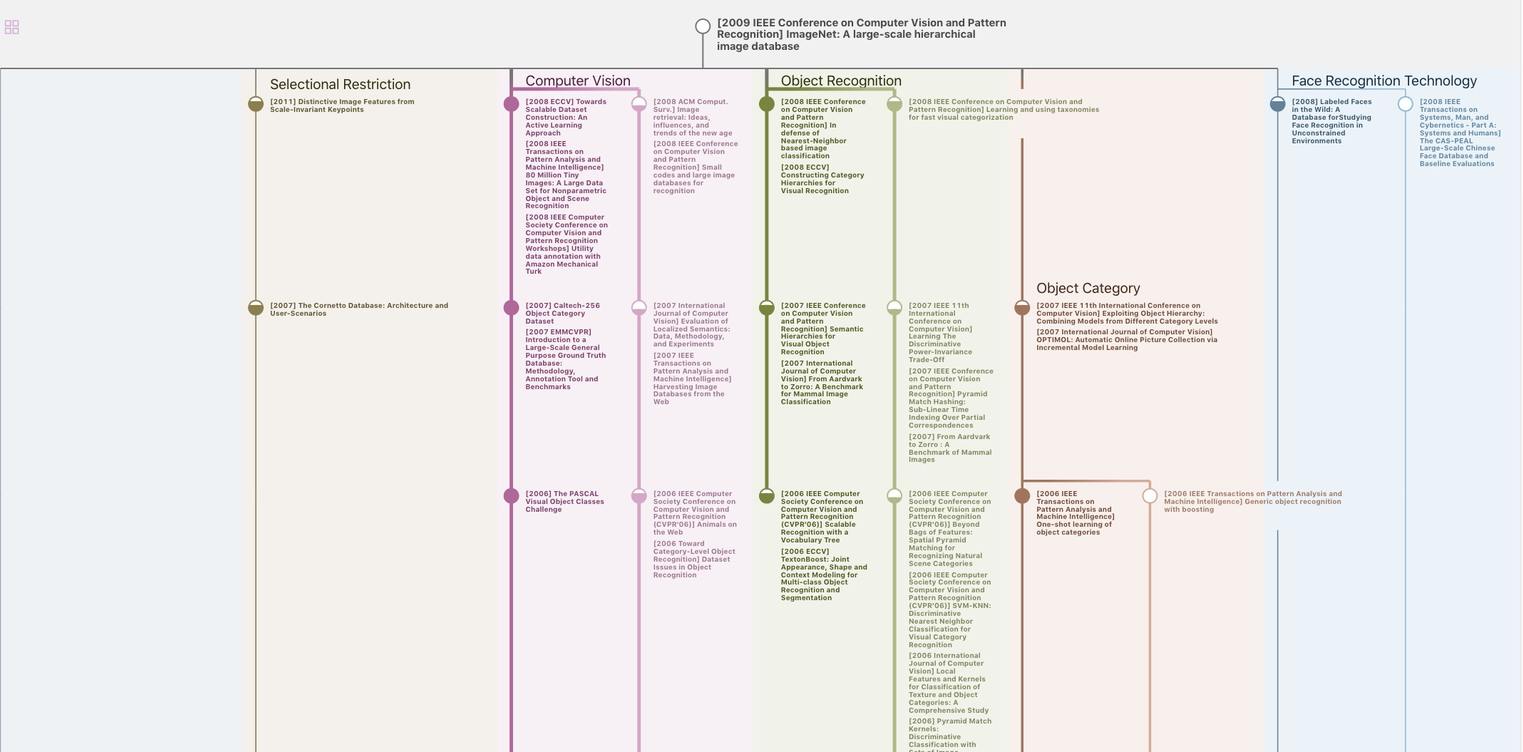
生成溯源树,研究论文发展脉络
Chat Paper
正在生成论文摘要