Application of principal component pursuit to process fault detection and diagnosis
ACC(2013)
摘要
Data-driven process monitoring has been extensively discussed in both academia and industry because of its applicability and effectiveness. One of the most applied techniques is the principal component analysis (PCA). Recently a new technique called principal component pursuit (PCP) is introduced. Compared to PCA, PCP is more robust to outliers. In this paper, the application of the PCP technique to process monitoring is thoroughly discussed from training data preprocessing to residual signal post-filtering. A new scaling preprocessing step is proposed to improve quality of data matrices in the sense of low coherence. A residual generator and a post-filter suitable for PCP generated process models are also provided. The post-filtered residual represents the fault signal, which makes the fault detection, isolation and reconstruction procedures simple and straightforward. A numerical example is provided to describe and illustrate the PCP-based process modeling and monitoring procedures.
更多查看译文
关键词
process monitoring,data-driven process monitoring,fault detection,training data preprocessing,pcp-based process monitoring,signal processing,residual generator,scaling preprocessing step,pcp generated process model,computerised monitoring,principal component pursuit,pcp-based process modeling,data matrix quality,fault signal,fault diagnosis,data handling,filtering theory,fault isolation,fault reconstruction,pcp technique,principal component analysis,post-filtered residual,residual signal post-filtering,vectors,coherence,sparse matrices,optimization
AI 理解论文
溯源树
样例
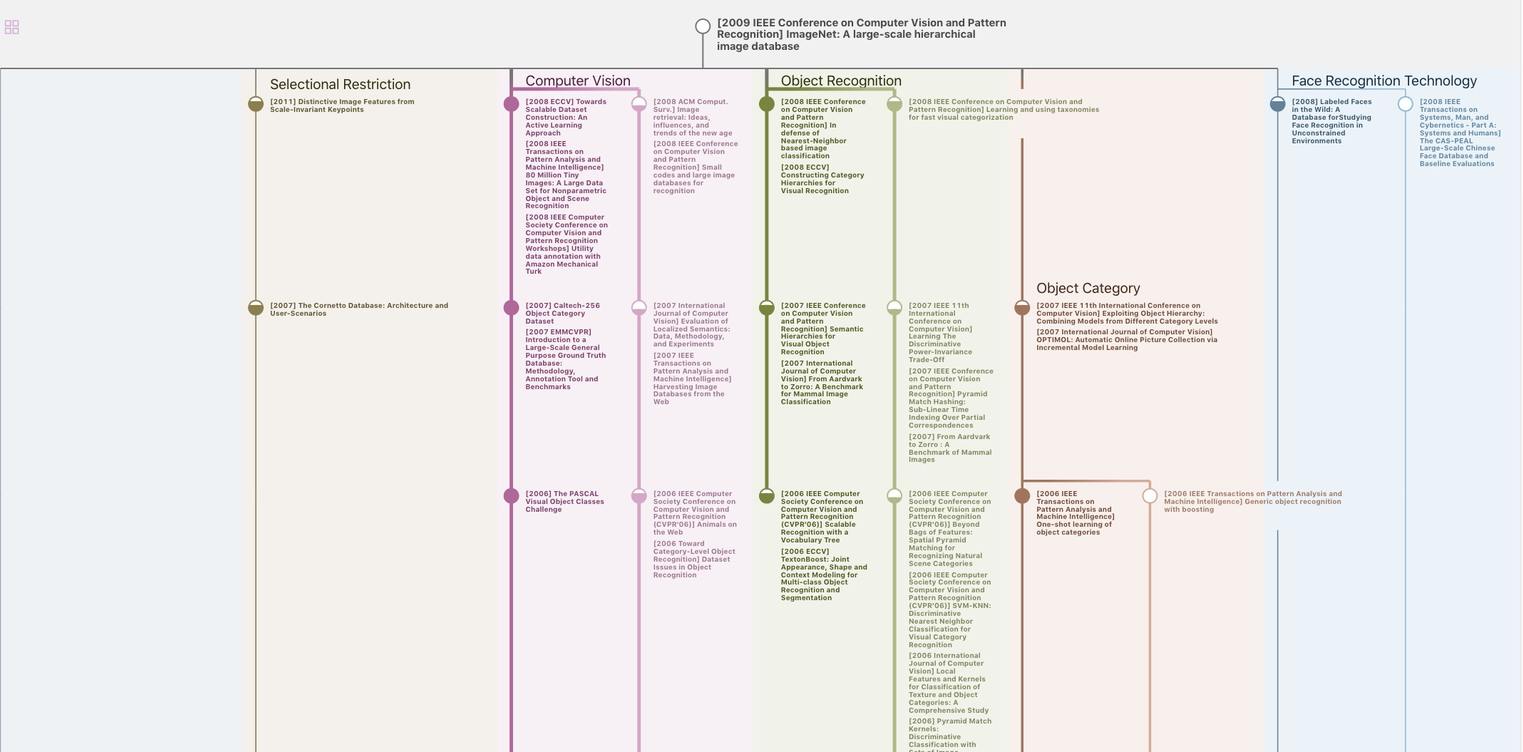
生成溯源树,研究论文发展脉络
Chat Paper
正在生成论文摘要