Community Detection-Based Feature Construction for Protein Sequence Classification.
BIOINFORMATICS RESEARCH AND APPLICATIONS (ISBRA 2015)(2015)
摘要
Machine learning algorithms are widely used to annotate biological sequences. Low-dimensional informative feature vectors can be crucial for the performance of the algorithms. In prior work, we have proposed the use of a community detection approach to construct low dimensional feature sets for nucleotide sequence classification. Our approach uses the Hamming distance between short nucleotide subsequences, called k-mers, to construct a network, and subsequently uses community detection to identify groups of k-mers that appear frequently in a set of sequences. While this approach worked well for nucleotide sequence classification, it could not be directly used for protein sequences, as the Hamming distance is not a good measure for comparing short protein k-mers. To address this limitation, we extend our prior approach by replacing the Hamming distance with substitution scores. Experimental results in different learning scenarios show that the features generated with the new approach are more informative than k-mers.
更多查看译文
关键词
Community detection,Feature construction,Feature selection,Dimensionality reduction,Protein sequence classification,Supervised learning,Semi-supervised learning,Domain adaptation
AI 理解论文
溯源树
样例
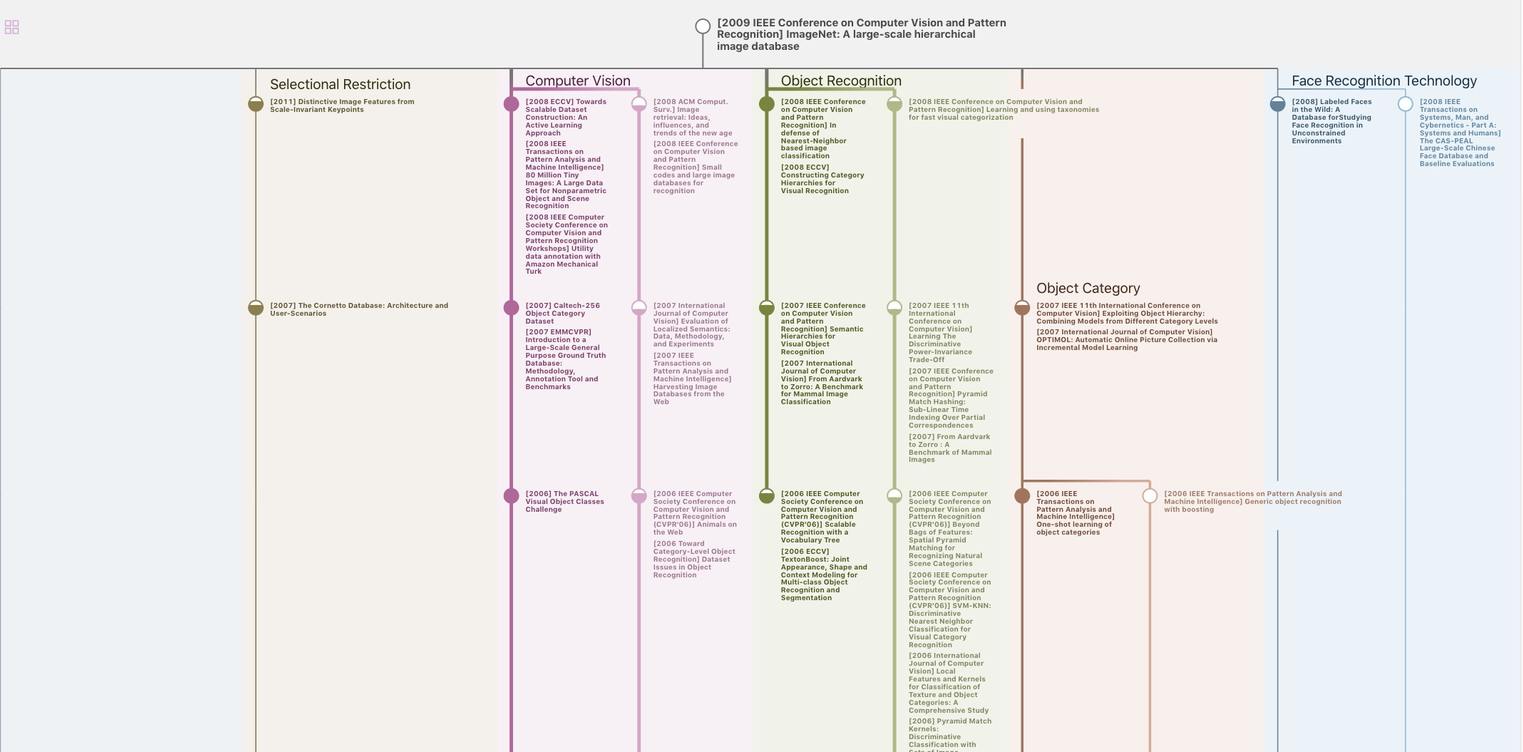
生成溯源树,研究论文发展脉络
Chat Paper
正在生成论文摘要