Sampling From Dense Streams Without Penalty: Improved Bounds For Frequency Moments And Heavy Hitters
COMPUTING AND COMBINATORICS, COCOON 2014(2014)
摘要
We investigate the ability to sample relatively small amounts of data from a stream and approximately calculate statistics on the original stream. Mc-Gregor et al. [29] provide worst case theoretical bounds that show space costs for sampling that are inversely correlated with the sampling rate. Indeed, while the lower bound of McGregor et al. cannot be improved in the general case, we show it is possible to improve the space bound for stream D of domain n, when the average positive frequency mu = F-1/F-0 is sufficiently large. We consider the following range of parameters: mu >= log (n) and sample rate p >= C-k mu(-1) log (n), where C-k is a constant. On these streams we improve the bound from (O) over tilde (1/pn(1-2/k)) to (O) over tilde (1/pn(1-2/k)) 0) thus giving polynomial improvement in space for sufficiently large /1 and p(-1).
更多查看译文
关键词
Streaming Algorithms,Sampling,Frequency Moments,Heavy Hitters
AI 理解论文
溯源树
样例
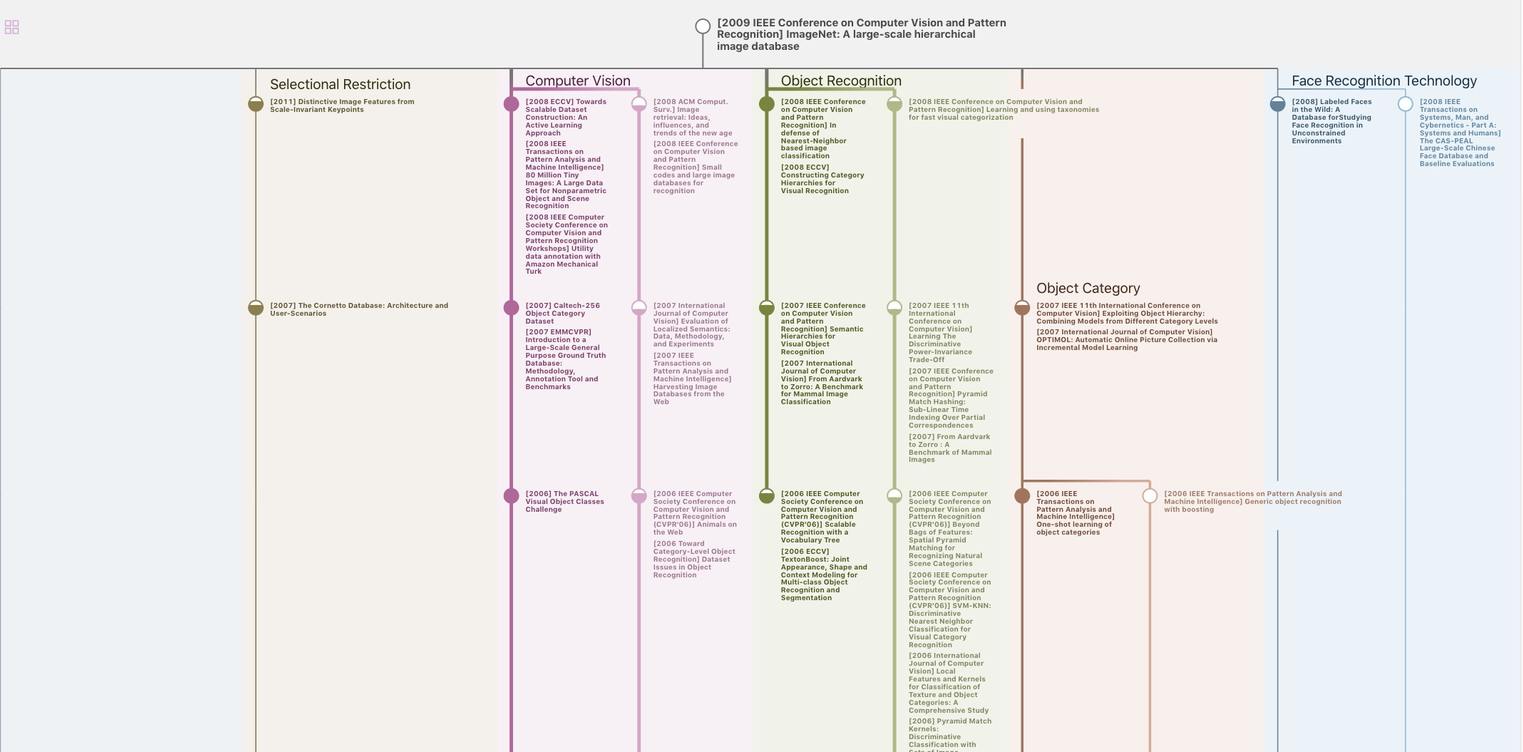
生成溯源树,研究论文发展脉络
Chat Paper
正在生成论文摘要