Clinical Risk Prediction With Multilinear Sparse Logistic Regression
KDD(2014)
摘要
Logistic regression is one core predictive modeling technique that has been used extensively in health and biomedical problems. Recently a lot of research has been focusing on enforcing sparsity on the learned model to enhance its effectiveness and interpretability, which results in sparse logistic regression model. However, no matter the original or sparse logistic regression, they require the inputs to be in vector form. This limits the applicability of logistic regression in the problems when the data cannot be naturally represented vectors (e.g., functional magnetic resonance imaging and electroencephalography signals). To handle the cases when the data are in the form of multi-dimensional arrays, we propose MulSLR: Multilinear Sparse Logistic Regression. MulSLR can be viewed as a high order extension of sparse logistic regression. Instead of solving one classification vector as in conventional logistic regression, we solve for K classification vectors in MulSLR (K is the number of modes in the data). We propose a block proximal descent approach to solve the problem and prove its convergence. The convergence rate of the proposed algorithm is also analyzed. Finally we validate the efficiency and effectiveness of MulSLR on predicting the onset risk of patients with Alzheimer's disease and heart failure.
更多查看译文
关键词
Logistic Regression,Multilinear,Proximal Gradient,Healthcare
AI 理解论文
溯源树
样例
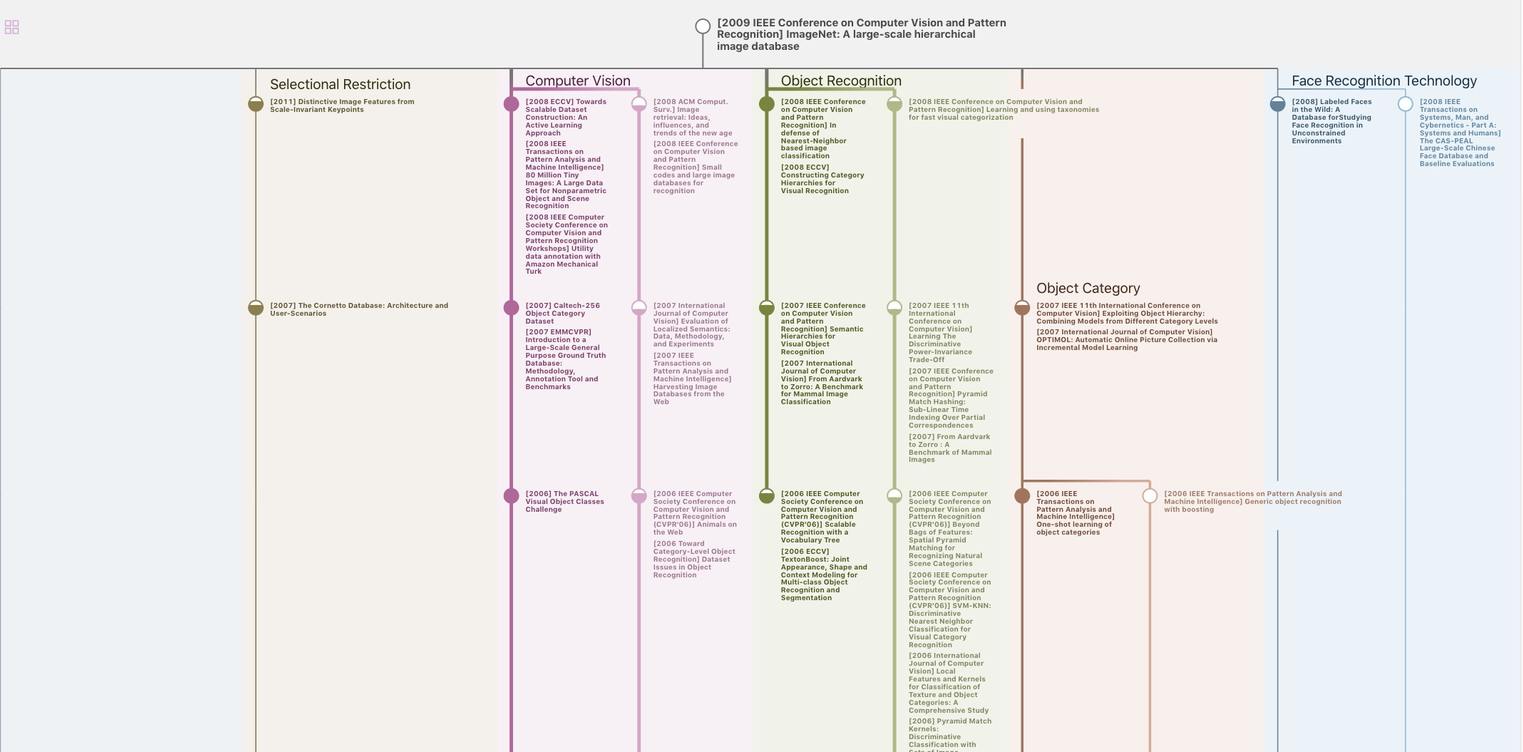
生成溯源树,研究论文发展脉络
Chat Paper
正在生成论文摘要