A Novel Contextual Topic Model for Query-Focused Multi-document Summarization
ICTAI(2014)
摘要
The problem of the oft-decried information overload negatively impacts comprehension of useful information. How to solve this problem has given rise to increase of interest in research on multi-document summarization. With the aim of seeking a new method to help justify the importance and similarity of sentences in multi-document summarization, this study proposes a novel approach based on well-known hierarchical Bayesian topic models. By investigating hierarchical topics and their correlations with respect to the lexical co-occurrences of words, the proposed contextual topic model can determine the relevance of sentences more effectively, and recognize latent topics and arrange them hierarchically as well. The quantitative evaluation results show that this model has outperformed hLDA and LDA in document modeling. In addition, a practical application demonstrates that a summarization system implementing this model can significantly improve the performance of summarization and make it comparable to state-of-the-art summarization systems.
更多查看译文
关键词
probabilistic inference,sentence similarity,machine learning, hierarchical topic model, text summarization,hierarchical bayesian topic models,hierarchical topic model,bayes methods,query-focused multidocument summarization,machine learning,document handling,unsupervised learning,text summarization,query processing
AI 理解论文
溯源树
样例
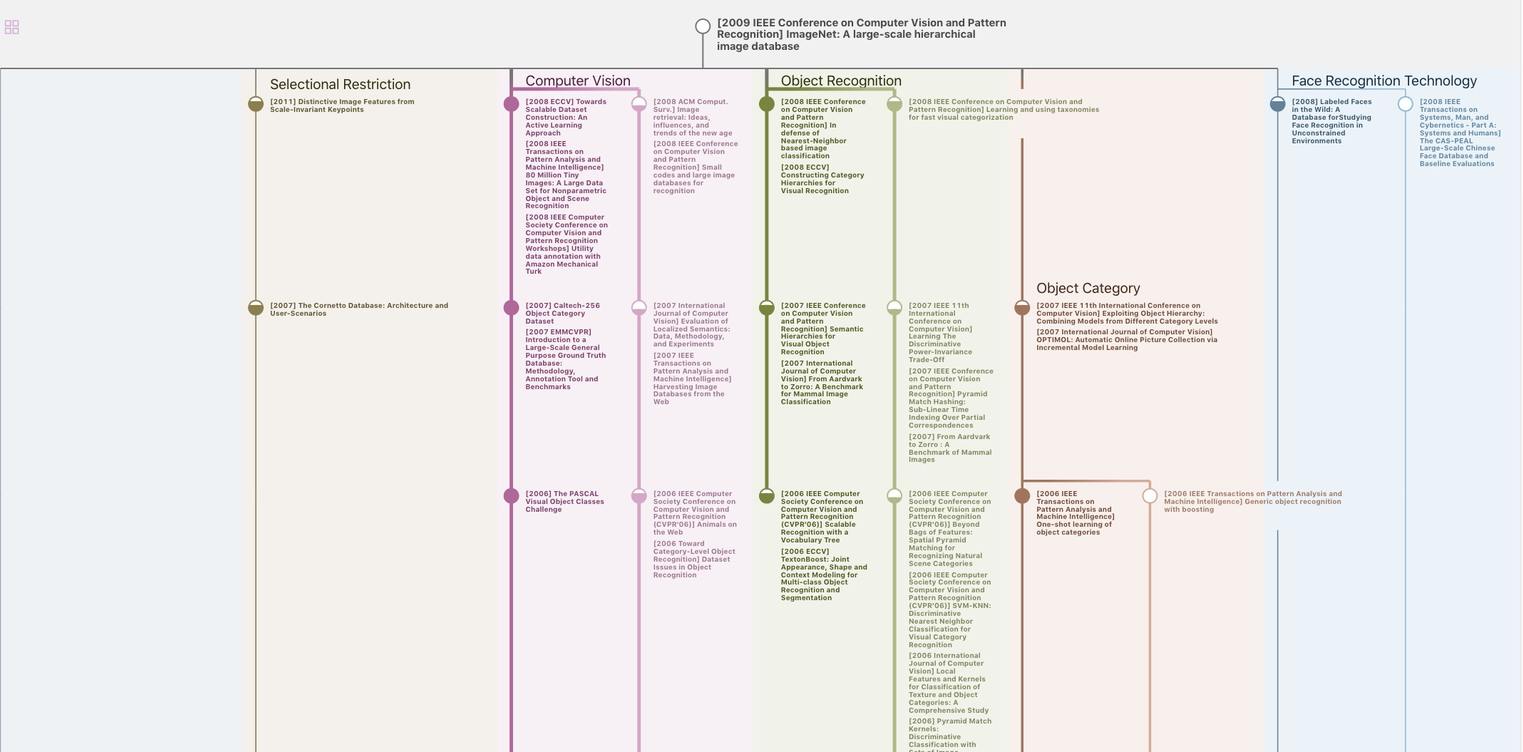
生成溯源树,研究论文发展脉络
Chat Paper
正在生成论文摘要