Clarisense: Clarifying Sensor Anomalies Using Social Network Feeds
2014 IEEE INTERNATIONAL CONFERENCE ON PERVASIVE COMPUTING AND COMMUNICATIONS WORKSHOPS (PERCOM WORKSHOPS)(2014)
摘要
The explosive growth in social networks that publish real-time content begs the question of whether their feeds can complement traditional sensors to achieve augmented sensing capabilities. One such capability is to explain anomalous sensor readings. Towards that end, in this paper, we build an automated anomaly clarification service, called ClariSense. It explains sensor anomalies using social network feeds. Explanation goes beyond detection. When a sensor network detects anomalous conditions, our system automatically suggests hypotheses that explain the likely causes of the anomaly to a human by identifying unusual social network feeds that seem to be correlated with the sensor anomaly in time and in space. To evaluate this service, we use real-time data feeds from the California traffic system that shares vehicle count and traffic speed on major California highways at 5 minute intervals. When anomalies are detected, our system automatically diagnoses their root cause by correlating the anomaly with feeds on Twitter. The identified cause is then compared to official traffic and incident reports, showing a great correspondence with ground truth.
更多查看译文
关键词
anomaly detection,entropy
AI 理解论文
溯源树
样例
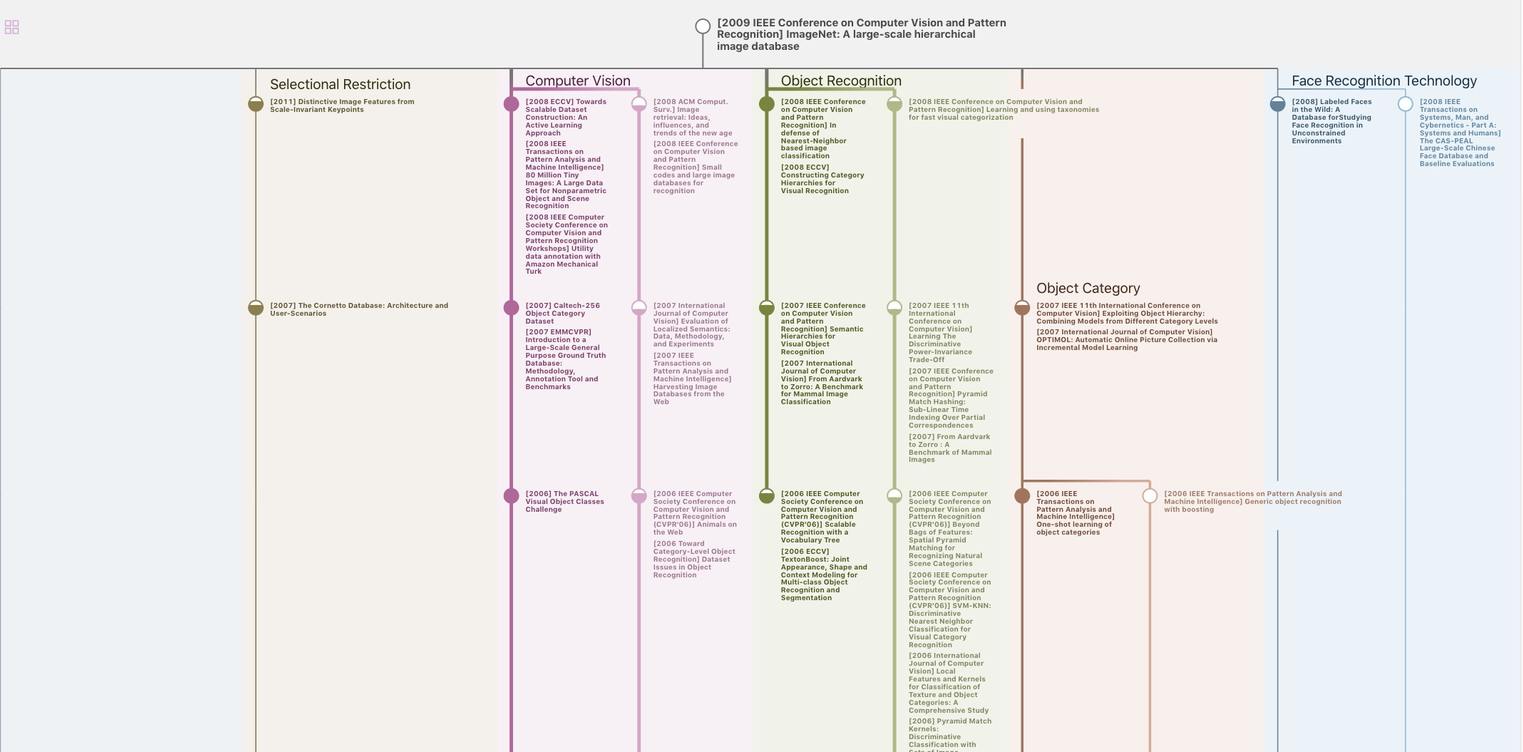
生成溯源树,研究论文发展脉络
Chat Paper
正在生成论文摘要