Action recognition using ensemble weighted multi-instance learning
ICRA(2014)
摘要
This paper deals with recognizing human actions in depth video data. Current state-of-the-art action recognition methods use hand-designed features, which are difficult to produce and time-consuming to extend to new modalities. In this paper, we propose a novel, 3.5D representation of a depth video for action recognition. A 3.5D graph of the depth video consists of a set of nodes that are the joints of the human body. Each joint is represented by a set of spatio-temporal features, which are computed by an unsupervised learning approach. However, if occlusions occur, the 3D positions of the joints are noisy which increases the intra-class variations in action classes. To address this problem, we propose the Ensemble Weighted Multi-Instance Learning approach (EnwMi) for the action recognition task. It considers the class imbalance and intra-class variations. We formulate the action recognition task with depth videos as a weighted multi-instance problem. We further integrate an ensemble learning method into the weighted multi-instance learning framework. Our approach is evaluated on Microsoft Research Action3D dataset, and the results show that it outperforms state-of-the-art methods.
更多查看译文
关键词
enwmi,image representation,ensemble weighted multiinstance learning,3.5d representation,human action recognition,image recognition,depth video,unsupervised learning,image motion analysis
AI 理解论文
溯源树
样例
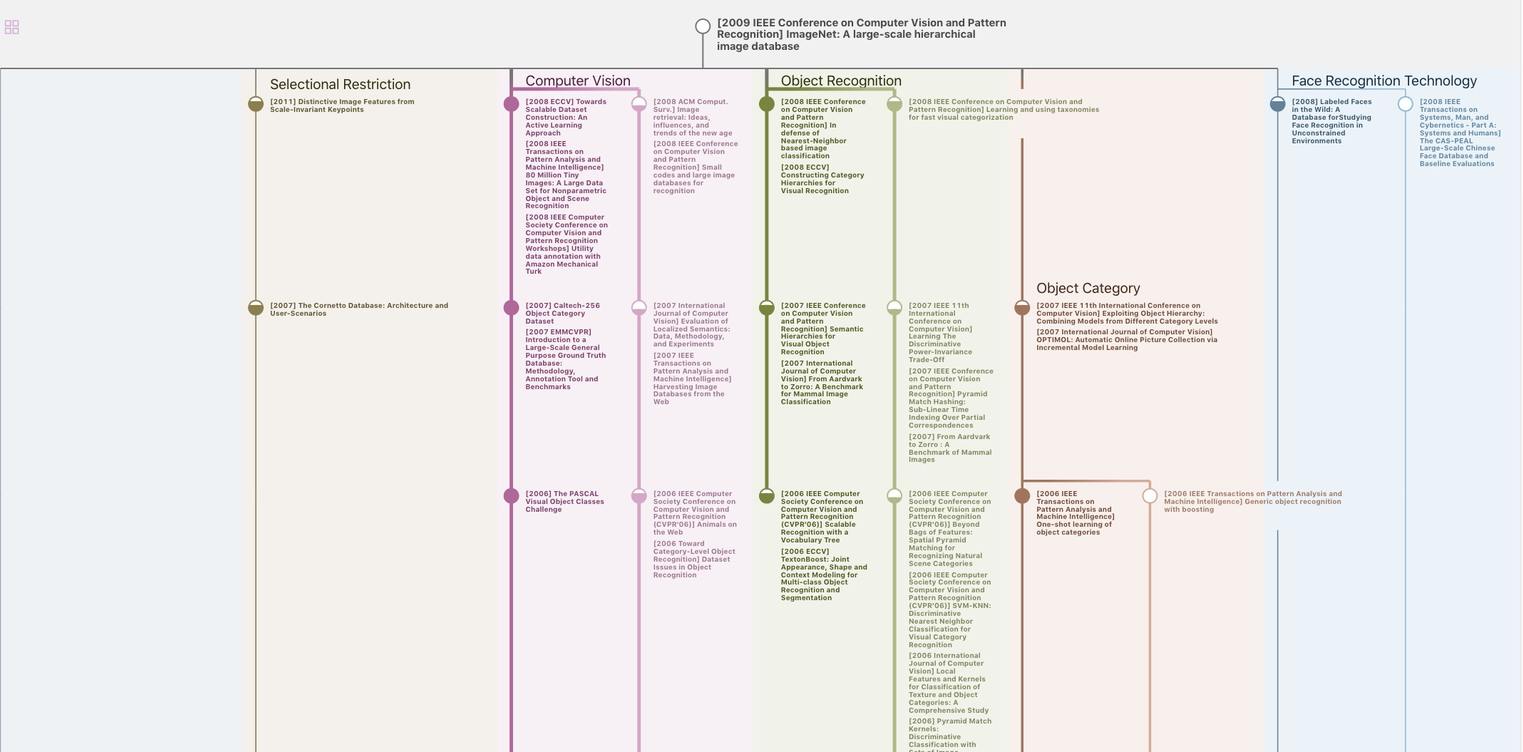
生成溯源树,研究论文发展脉络
Chat Paper
正在生成论文摘要