PROT: Productive regions oriented task space path planning for hyper-redundant manipulators
ICRA(2014)
摘要
In this paper we propose a novel efficient sampling bias technique to improve the performance of a task space trajectory planner for hyper-redundant manipulators. We defines productive regions in the task space as a set of states that can lead effectively to a goal state. We first compute a maximum reachable area (MRA) where a robot can reach from the node by an employed local planner for a node in the task space. When the MRA of a node contains the goal state, we call it promising and bias our sampling to cover promising MRAs. When the MRA does not contain the goal state, we call it unpromising and construct a detour sampling domain for detouring operations from obstacles constraining the manipulator. The union of promising MRAs and detour sampling domains approximates our productive regions, and we bias our sampling to cover these domains more. We have applied our Productive Regions Oriented Task space planner (PROT) to various types of robots in R2 task space and achieved up to 3.54 times improvement over the state-of-the-art task space planner. We have additionally verified the benefits of our method by applying it to cabled mobile robot planning.
更多查看译文
关键词
task space trajectory planner,R2 task space,maximum reachable area,MRA,sampling bias technique,hyperredundant manipulators,mobile robots,PROT,redundant manipulators,productive region oriented task space path planning,path planning,cabled mobile robot planning,sampling methods,detour sampling domain
AI 理解论文
溯源树
样例
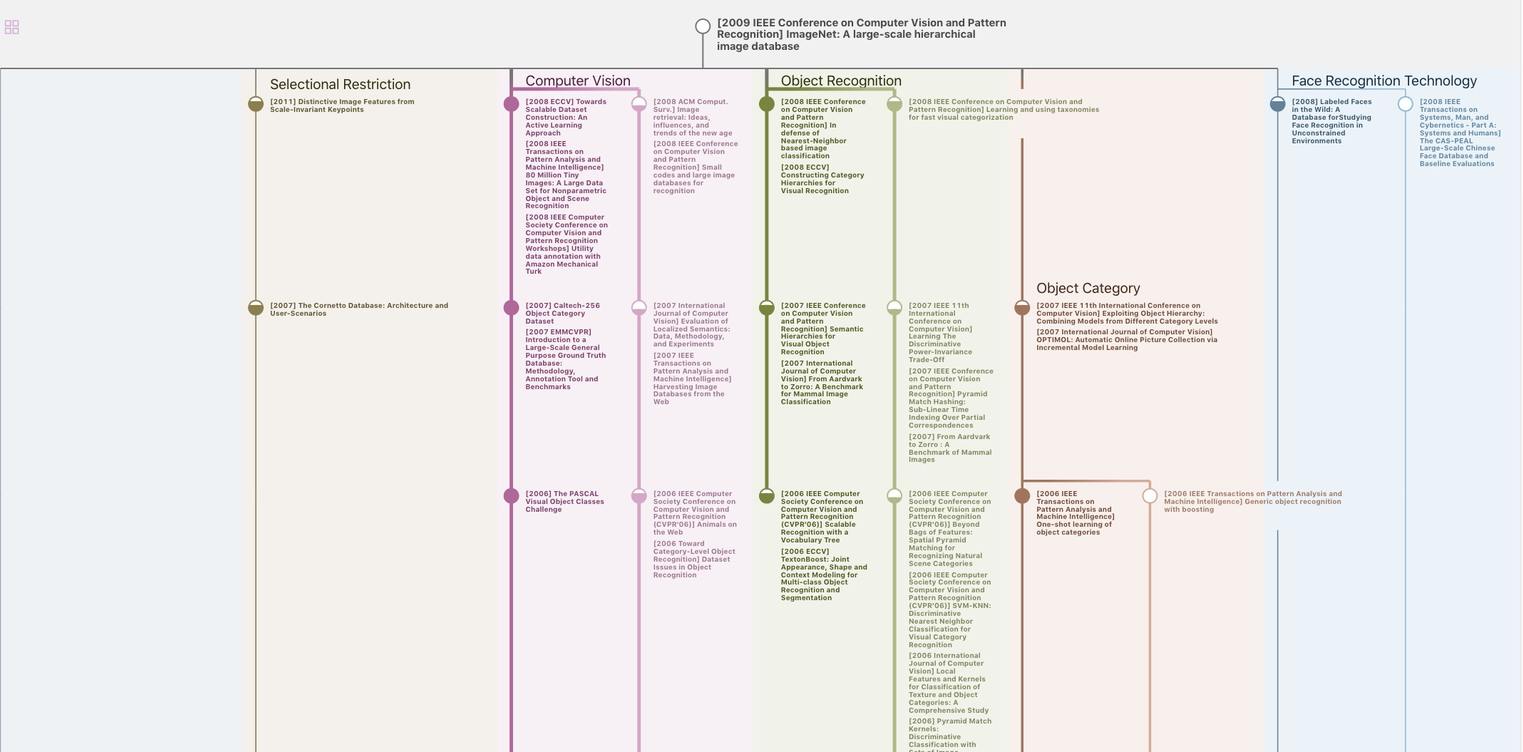
生成溯源树,研究论文发展脉络
Chat Paper
正在生成论文摘要