Rumor source detection with multiple observations: fundamental limits and algorithms.
SIGMETRICS '14: ACM SIGMETRICS / International Conference on Measurement and Modeling of Computer Systems Austin Texas USA June, 2014(2014)
摘要
This paper addresses the problem of a single rumor source detection with multiple observations, from a statistical point of view of a spreading over a network, based on the susceptible-infectious model. For tree networks, multiple sequential observations for one single instance of rumor spreading cannot improve over the initial snapshot observation. The situation dramatically improves for multiple independent observations. We propose a unified inference framework based on the union rumor centrality, and provide explicit detection performance for degree-regular tree networks. Surprisingly, even with merely two observations, the detection probability at least doubles that of a single observation, and further approaches one, i.e., reliable detection, with increasing degree. This indicates that a richer diversity enhances detectability. For general graphs, a detection algorithm using a breadth-first search strategy is also proposed and evaluated. Besides rumor source detection, our results can be used in network forensics to combat recurring epidemic-like information spreading such as online anomaly and fraudulent email spams.
更多查看译文
AI 理解论文
溯源树
样例
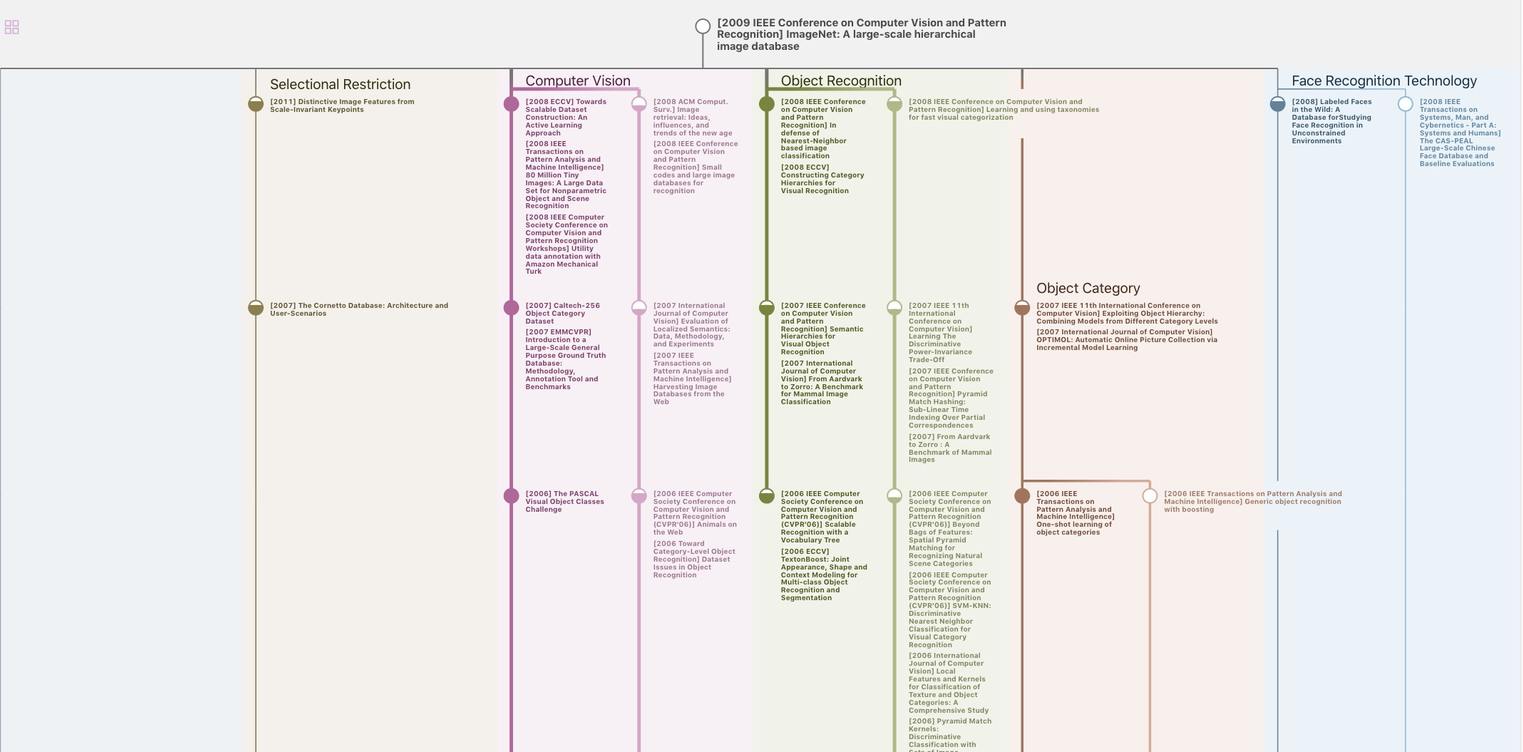
生成溯源树,研究论文发展脉络
Chat Paper
正在生成论文摘要