Mean shrinkage improves the classification of ERP signals by exploiting additional label information
Tubingen(2014)
摘要
Linear discriminant analysis (LDA) is the most commonly used classification method for single trial data in a brain-computer interface (BCI) framework. The popularity of LDA arises from its robustness, simplicity and high accuracy. However, the standard LDA approach is not capable to exploit sublabel information (such as stimulus identity), which is accessible in data from event related potentials (ERPs): it assumes that the evoked potentials are independent of the stimulus identity and dependent only on the users' attentional state. We question this assumption and investigate several methods which extract subclass-specific features from ERP data. Moreover, we propose a novel classification approach which exploits subclass-specific features using mean shrinkage. Based on a reanalysis of two BCI data sets, we show that our novel approach outperforms the standard LDA approach, while being computationally highly efficient.
更多查看译文
关键词
bioelectric potentials,brain-computer interfaces,electroencephalography,feature extraction,medical signal processing,signal classification,BCI data sets,ERP signal classification,additional label information,brain-computer interface,electroencephalography,event related potentials,evoked potentials,linear discriminant analysis,mean shrinkage,novel classification approach,single trial data,standard LDA approach,stimulus identity,subclass-specific feature extraction,subclass-specific features,sublabel information,users attentional state
AI 理解论文
溯源树
样例
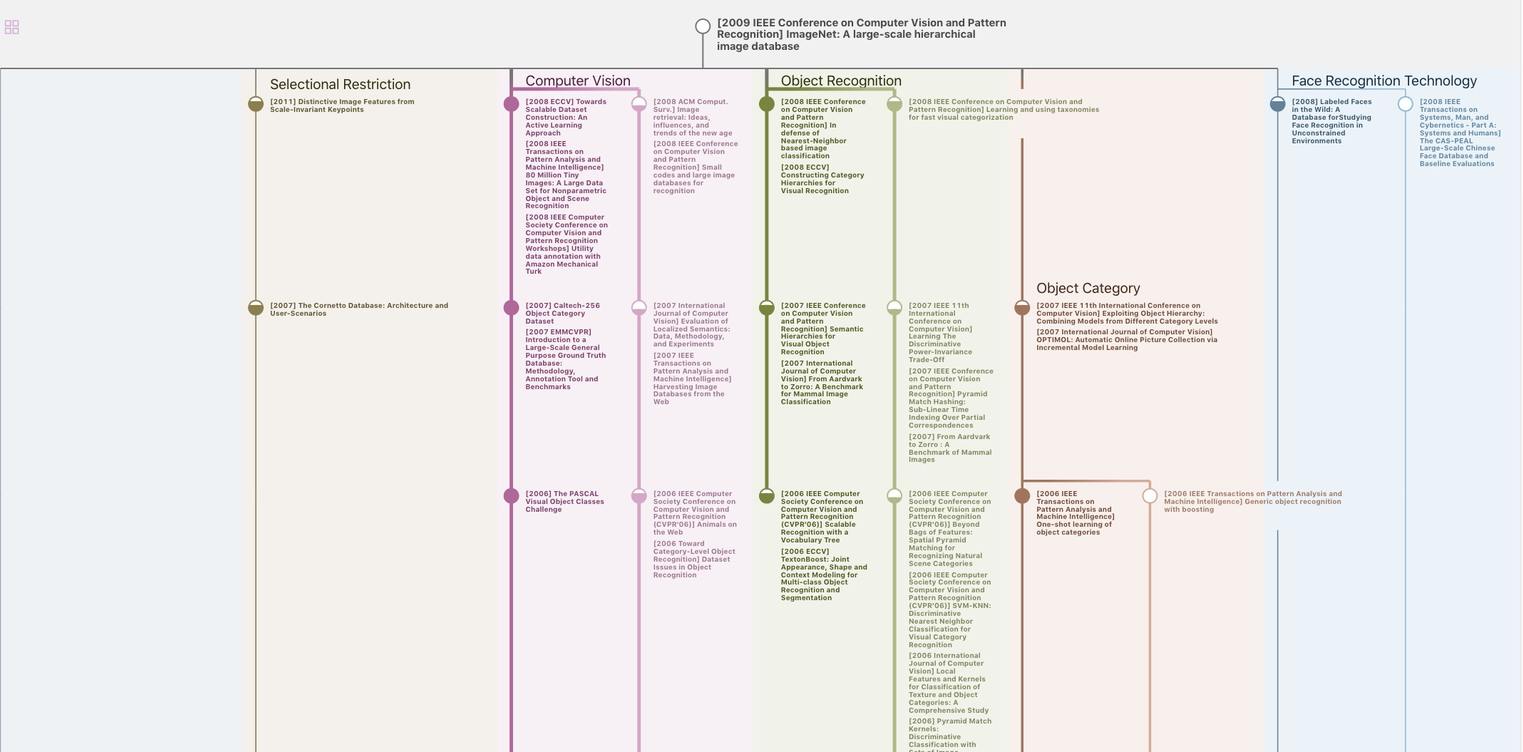
生成溯源树,研究论文发展脉络
Chat Paper
正在生成论文摘要