GeoF: A geometric Bayesian filter for indoor position tracking in mixed LOS/NLOS conditions
WPNC(2014)
摘要
A large number of indoor positioning systems are based on sensor networks or WLAN ranging techniques with a filter to remove the positioning uncertainty coming from the ranging errors. Bayesian filter has emerged as a useful approach for sequential position estimation, which generally resorts to a numerical solution due to the nonlinearity and the non-Gaussian nature of mobile positioning. The accuracy of numerical Bayesian approaches depends mostly on two factors: the sample density of the state approximation and how closely the state transition model mimics the true motion of each iteration. However, dense samples typically cause high computation and memory complexity; worse, an improper transition model can lead to the problem of filter divergence. We hold that in the presence of at least one line-of-sight (LOS) range, the state space can be effectively confined by the geometries of ranging measurements. Therefore, this paper proposes a geometric filter (GeoF) learning the transition model by the geometry of the most recent TOA ranges. The key idea of GeoF is to adaptively generate the sample set of the state based on the intersections of every pair-wise range circles. Therefore, our approach employs a very small number of samples, causing much smaller implementation and computation overhead compared to general numerical Bayesian approaches. The experiment results of mobile robot localization in typical LOS/NLOS mixed scenarios show that GeoF yields better performance over extended Kalman filter, generic particle filter and grid-based filter.
更多查看译文
关键词
nonline-of-sight,los/nlos toa ranging,indoor position tracking,particle filtering (numerical methods),mobile positioning,positioning uncertainty,kalman filters,tracking filters,bayes methods,toa ranges,geof,mobile robots,mobile robot localization,numerical bayesian methods,state transition model,state approximation,geometric positioning,sensor networks,sequential position estimation,indoor positioning systems,grid-based filter,generic particle filter,geometric bayesian filter,extended kalman filter,time-of-arrival estimation,mixed los-nlos conditions,iterative methods,nonlinear optics,estimation,geometry
AI 理解论文
溯源树
样例
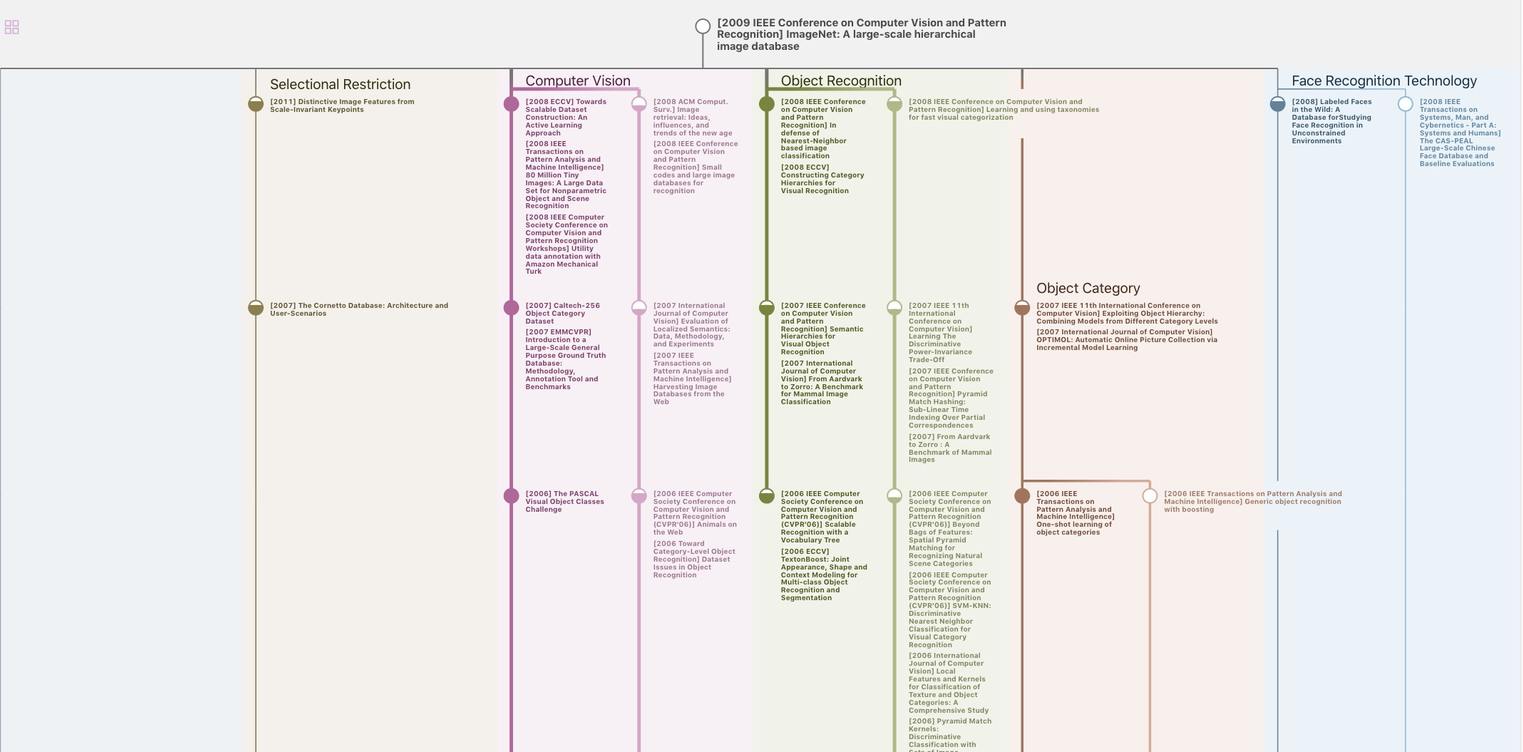
生成溯源树,研究论文发展脉络
Chat Paper
正在生成论文摘要