Cramér-Rao lower bound analysis for wireless localization systems using priori information
WPNC(2014)
摘要
Bayesian estimation methods are widely used for wireless localization systems. They employ priori information and current measurement error distribution models to derive the state of a mobile target. Cramér-Rao lower bound (CRLB) is a fundamental tool to analyze the performance of Bayesian estimators. Although CRLB is derived based on the measurement error distribution, only a few works have investigated the performance using priori information. In this paper, we derive the CRLB formulation in three cases by using the priori information: (1) fundamental Bayesian process; (2) recursive process; (3) adaptive process. These three processes represent the common Bayesian tracking algorithms for wireless system. Simulations are constructed to compare the localization performance according to the different processes. The results indicate how the priori information influences the location estimation and how to improve the performance according to different scenarios.
更多查看译文
关键词
recursive process,mobile target,indoor localization,bayes methods,bayesian estimation,cramér-rao lower bound,fundamental bayesian process,current measurement error distribution model,radio networks,priori information,wireless localization systems,bayesian estimation methods,priori information model,recursive estimation,adaptive process,crlb,mobile computing,bayesian tracking algorithms,cramέr-rao lower bound analysis,cramer rao lower bound,wireless communication,wireless sensor networks,estimation,noise measurement,measurement errors,noise
AI 理解论文
溯源树
样例
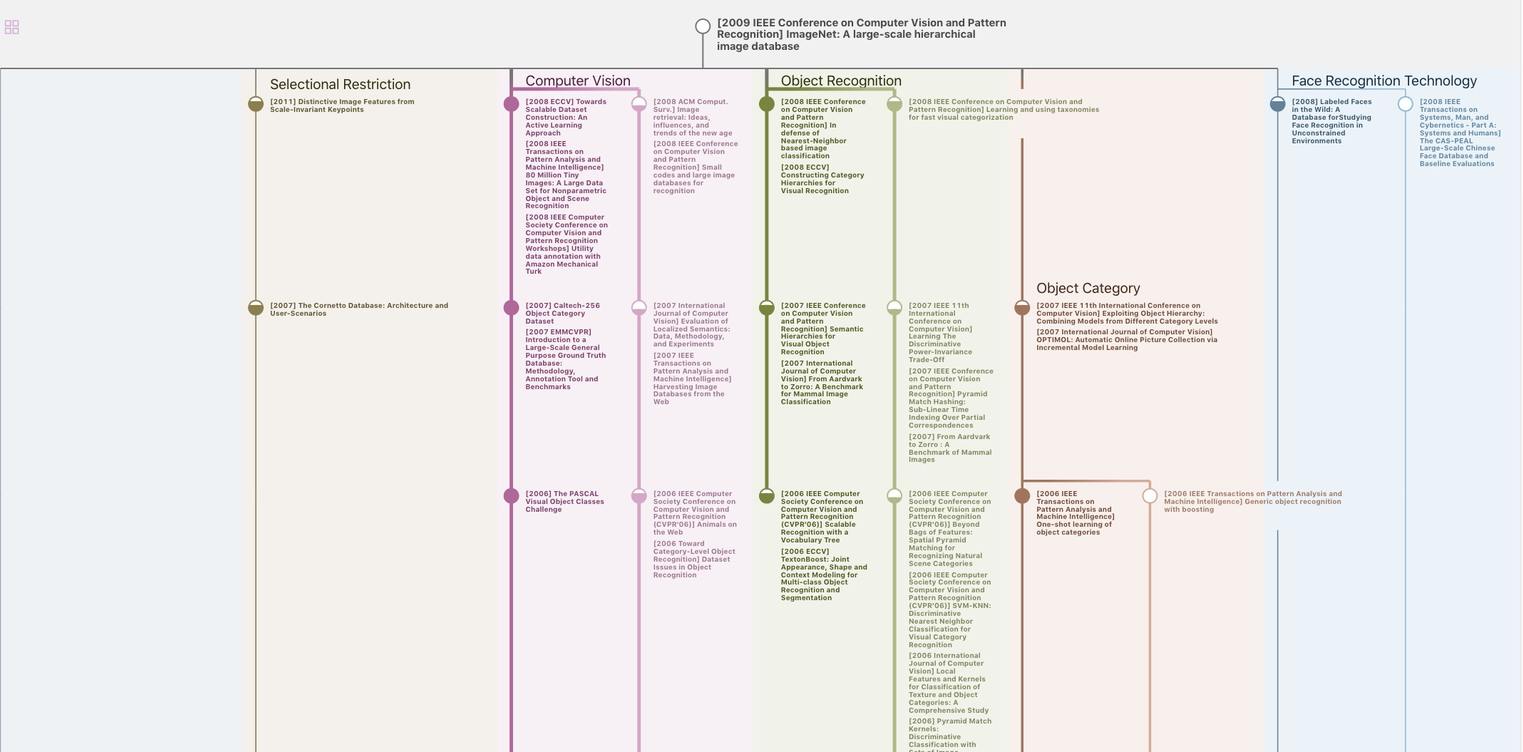
生成溯源树,研究论文发展脉络
Chat Paper
正在生成论文摘要