Limited Labels For Unlimited Data: Active Learning For Speaker Recognition
15TH ANNUAL CONFERENCE OF THE INTERNATIONAL SPEECH COMMUNICATION ASSOCIATION (INTERSPEECH 2014), VOLS 1-4(2014)
摘要
In this paper, we attempt to quantify the amount of labeled data necessary to build a state-of-the-art speaker recognition system. We begin by using i-vectors and the cosine similarity metric to represent an unlabeled set of utterances, then obtain labels from a noiseless oracle in the form of pairwise queries. Finally, we use the resulting speaker clusters to train a PLDA scoring function, which is assessed on the 2010 NIST Speaker Recognition Evaluation. After presenting the initial results of an algorithm that sorts queries based on nearest-neighbor pairs, we develop techniques that further minimize the number of queries needed to obtain state-of-the-art performance. We show the generalizability of our methods in anecdotal fashion by applying our methods to two different distributions of utterances-per-speaker and, ultimately, find that the actual number of pairwise labels needed to obtain state-of-the-art results may be a mere fraction of the queries required to fully label the entire set of utterances.
更多查看译文
关键词
speaker recognition,i-vectors,active learning
AI 理解论文
溯源树
样例
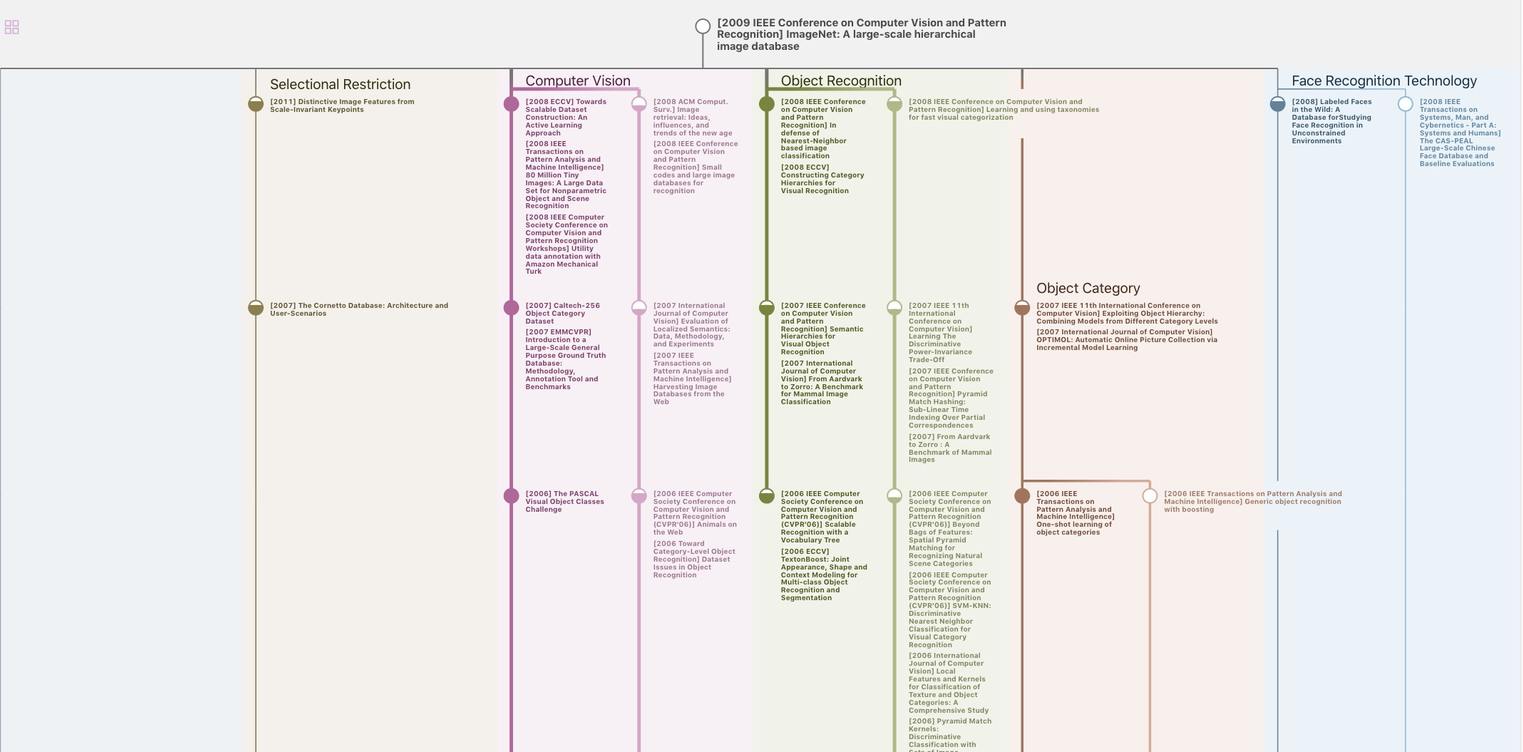
生成溯源树,研究论文发展脉络
Chat Paper
正在生成论文摘要