Utilization of Exercise Difficulty Rating by Students for Recommendation.
Lecture Notes in Computer Science(2014)
摘要
Recommendation plays a vital role in adaptive educational systems. Learners often face large body of educational materials including not only texts (explanations), but also interactive content such as exercises and questions. These require various knowledge levels of multiple topics. For effective learning, personalized recommendation of the most appropriate items according to the learner's current knowledge level and preferences is an essential feature. In this paper, we describe a learning object recommendation method based on students' explicit difficulty ratings during and after exercise/question solving. It is based on comparing the learner's state when the recommendation is to be made against his peers with similar knowledge in the moment when they rated the difficulty. To deal with sparsity of ratings that are even further filtered, we also propose two solutions to either adaptively elicit ratings in appropriate moments during learners work, or to predict ratings from implicit user actions. We evaluate the method in ALEF - adaptive web-based educational system.
更多查看译文
关键词
Learning object difficulty,Exercise difficulty rating,Personalized recommendation,Rating prediction,Learning network
AI 理解论文
溯源树
样例
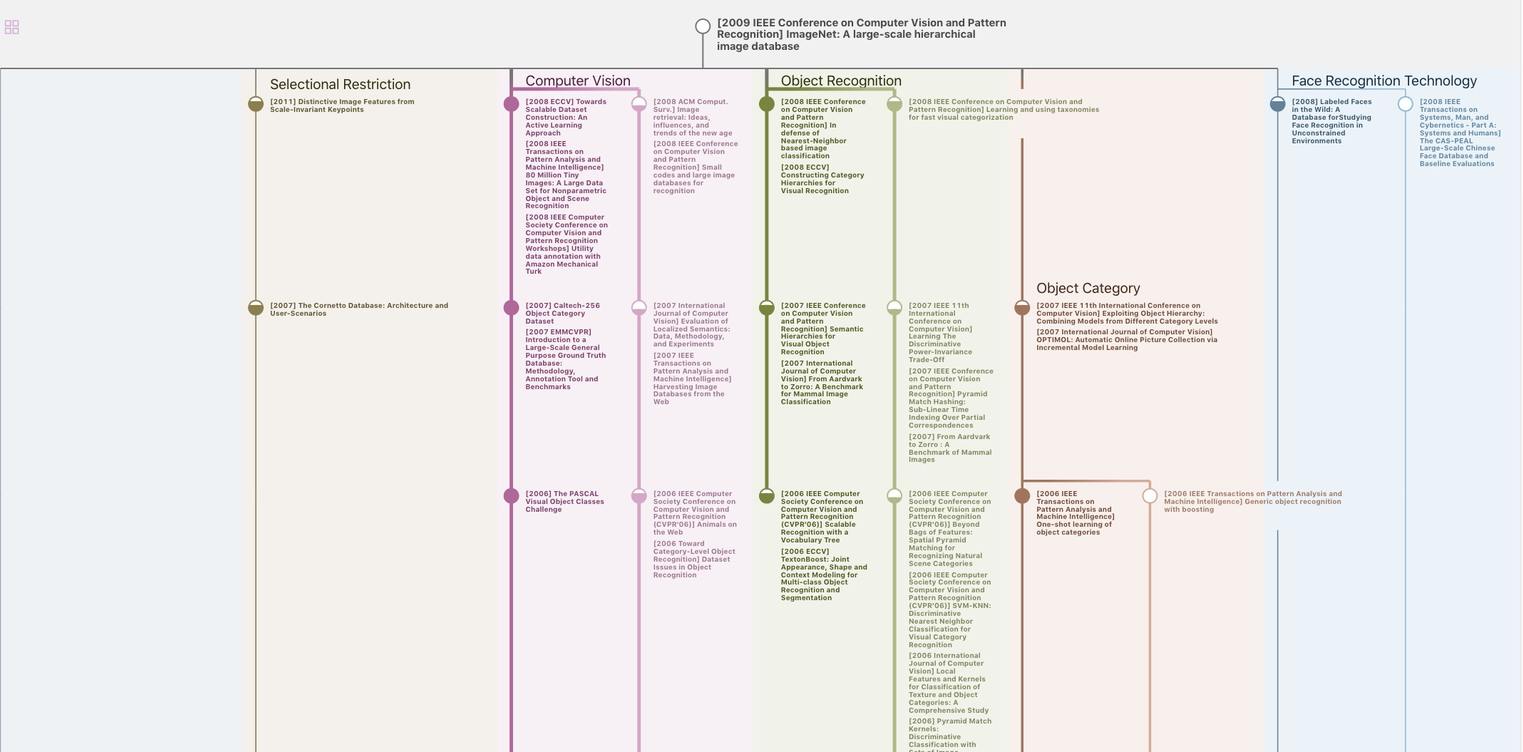
生成溯源树,研究论文发展脉络
Chat Paper
正在生成论文摘要