Unsupervised Active Learning of CRF Model for Cross-Lingual Named Entity Recognition
ANNPR(2014)
摘要
Manual annotation of the training data of information extraction models is a time consuming and expensive process but necessary for the building of information extraction systems. Active learning has been proven to be effective in reducing manual annotation efforts for supervised learning tasks where a human judge is asked to annotate the most informative examples with respect to a given model. However, in most cases reliable human judges are not available for all languages. In this paper, we propose a cross-lingual unsupervised active learning paradigm (XLADA) that generates high-quality automatically annotated training data from a word-aligned parallel corpus. To evaluate our paradigm, we applied XLADA on English-French and English-Chinese bilingual corpora then we trained French and Chinese information extraction models. The experimental results show that XLADA can produce effective models without manually-annotated training data.
更多查看译文
关键词
cross- lingual domain adaptation,information extraction,named entity recognition,unsupervised active learning
AI 理解论文
溯源树
样例
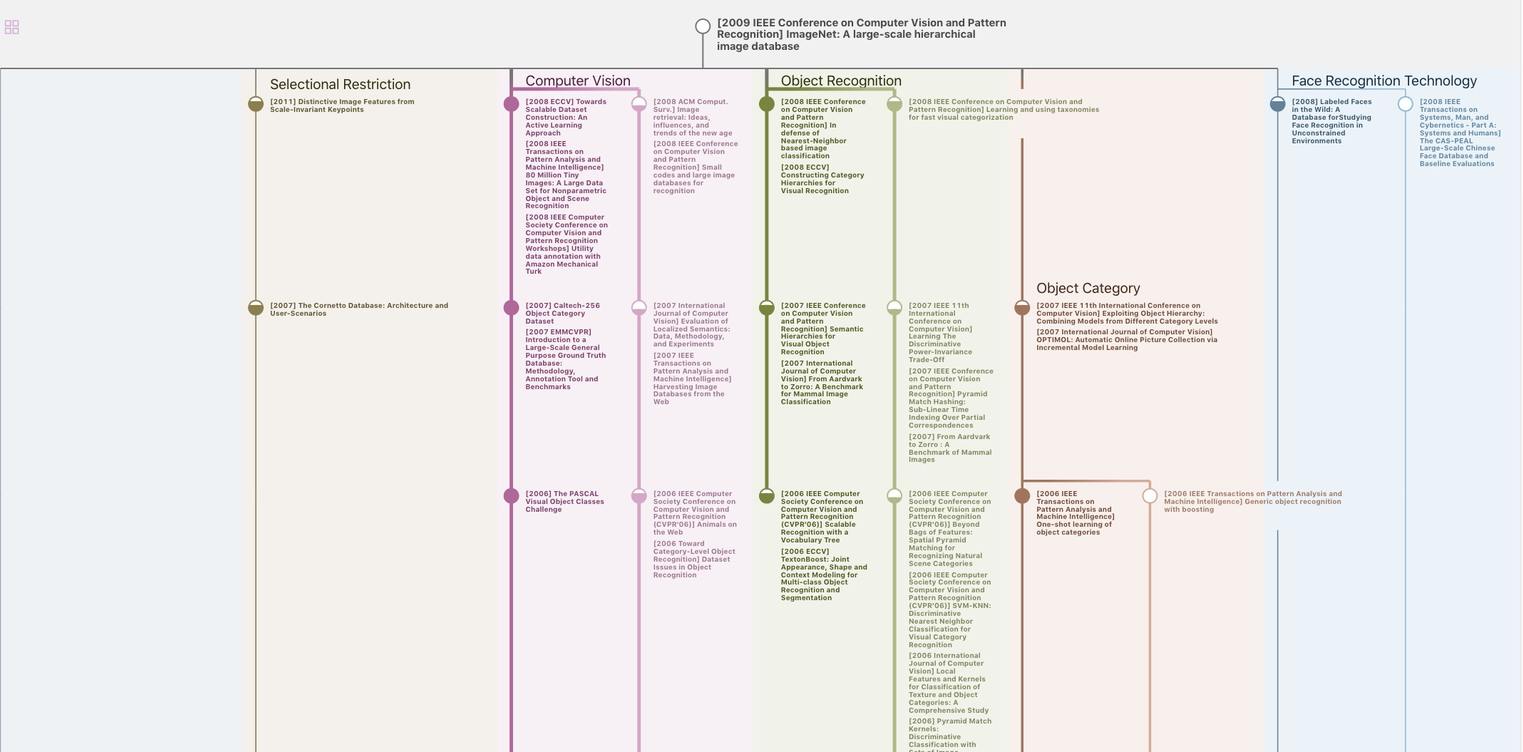
生成溯源树,研究论文发展脉络
Chat Paper
正在生成论文摘要