A Unifying View of Representer Theorems.
ICML'14: Proceedings of the 31st International Conference on International Conference on Machine Learning - Volume 32(2014)
摘要
It is known that the solution of regularization and interpolation problems with Hilbertian penalties can be expressed as a linear combination of the data. This very useful property, called the representer theorem , has been widely studied and applied to machine learning problems. Analogous optimality conditions have appeared in other contexts, notably in matrix regularization. In this paper we propose a unified view , which generalizes the concept of representer theorems and extends necessary and sufficient conditions for such theorems to hold. Our main result shows a close connection between representer theorems and certain classes of regularization penalties, which we call orthomonotone functions . This result not only subsumes previous representer theorems as special cases but also yields a new class of optimality conditions, which goes beyond the classical linear combination of the data. Moreover, orthomonotonicity provides a useful criterion for testing whether a representer theorem holds for a specific regularization problem.
更多查看译文
AI 理解论文
溯源树
样例
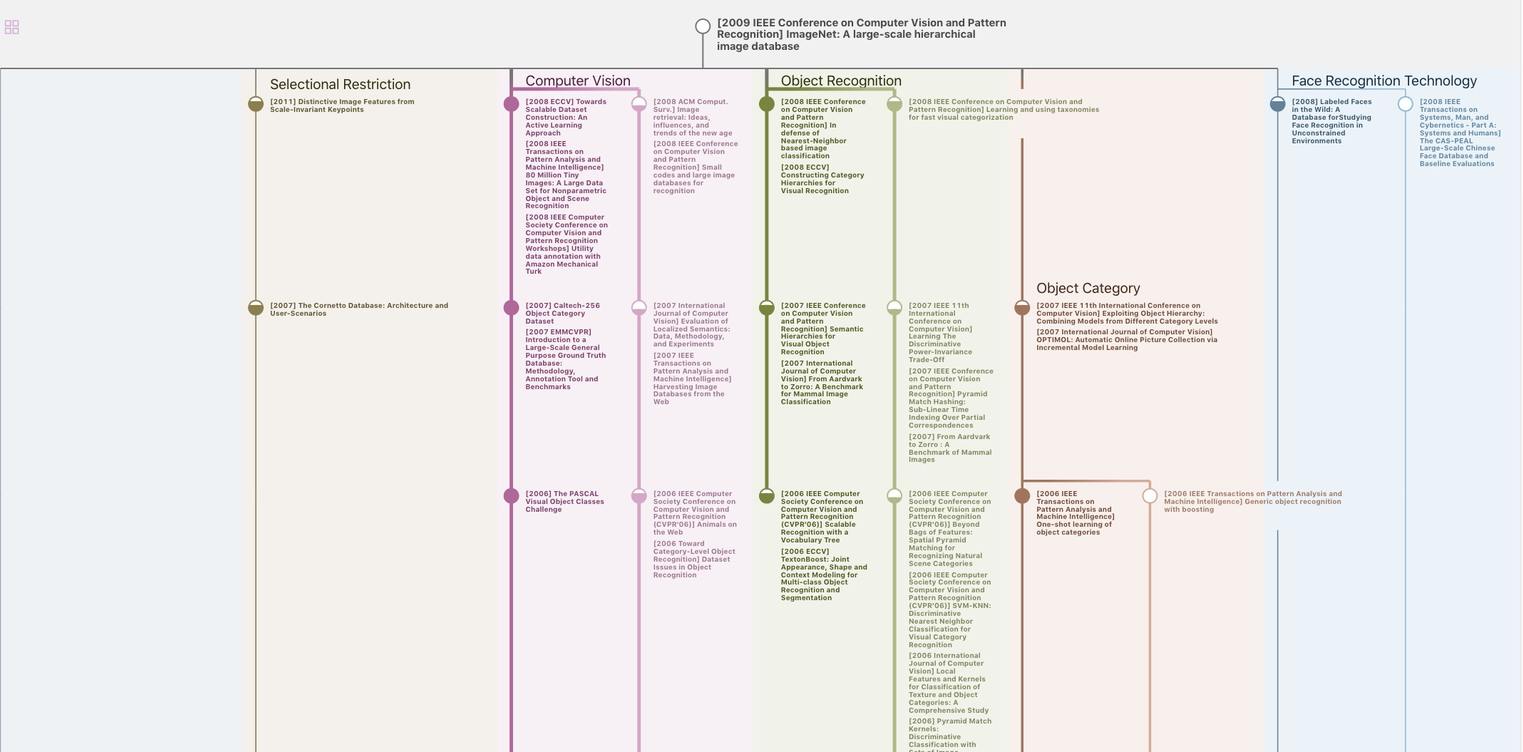
生成溯源树,研究论文发展脉络
Chat Paper
正在生成论文摘要