Finito: A Faster, Permutable Incremental Gradient Method for Big Data Problems
ICML'14: Proceedings of the 31st International Conference on International Conference on Machine Learning - Volume 32(2014)
摘要
Recent advances in optimization theory have shown that smooth strongly convex finite sums can be minimized faster than by treating them as a black box "batch" problem. In this work we introduce a new method in this class with a theoretical convergence rate four times faster than existing methods, for sums with sufficiently many terms. This method is also amendable to a sampling without replacement scheme that in practice gives further speed-ups. We give empirical results showing state of the art performance.
更多查看译文
AI 理解论文
溯源树
样例
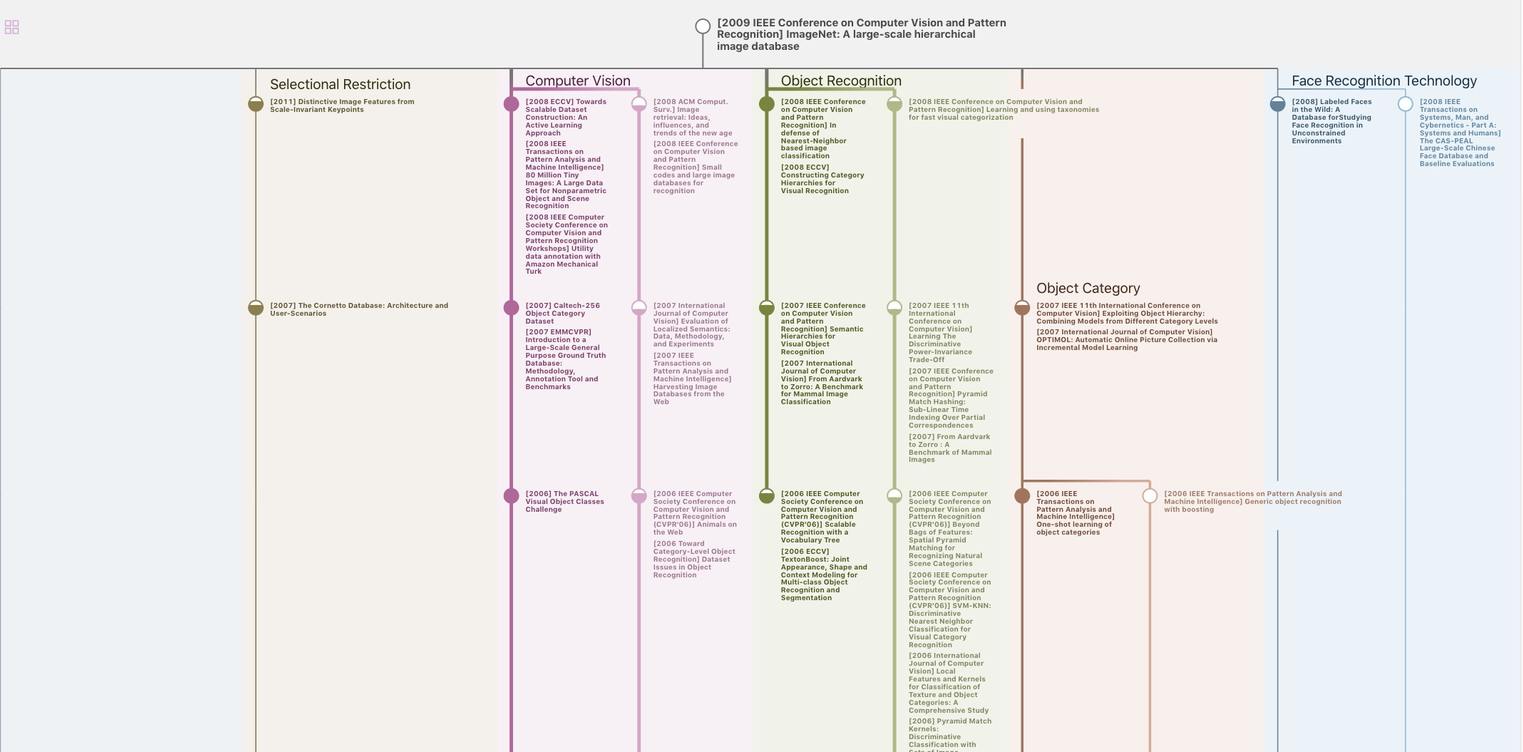
生成溯源树,研究论文发展脉络
Chat Paper
正在生成论文摘要