Reasoning Over Relations Based On Chinese Knowledge Bases
CHINESE COMPUTATIONAL LINGUISTICS AND NATURAL LANGUAGE PROCESSING BASED ON NATURALLY ANNOTATED BIG DATA, CCL 2014(2014)
摘要
Knowledge bases are useful resource for many applications, but reasoning new relationships between new entities based on them is difficult because they often lack the knowledge of new relations and entities. In this paper, we introduce the novel Neural Tensor Network (NTN)[1] model to reason new facts based on Chinese knowledge bases. We represent entities as an average of their constituting word or character vectors, which share the statistical strength between entities, such as and. The NTN model uses a tensor network to replace a standard neural layer, which strengthen the interaction of two entity vectors in a simple and efficient way. In experiments, we compare the NTN and several other models, the results show that all models' performance can be improved when word vectors are pre-trained from an unsupervised large corpora and character vectors don't have this advantage. The NTN model outperforms others and reachs high classification accuracy 91.1% and 89.6% when using pre-trained word vectors and random character vectors, respectively. Therefore, when Chinese word segmentation is a difficult task, initialization with random character vectors is a feasible choice.
更多查看译文
关键词
knowledge bases,reason,Neural Tensor Network,word representations,character representations
AI 理解论文
溯源树
样例
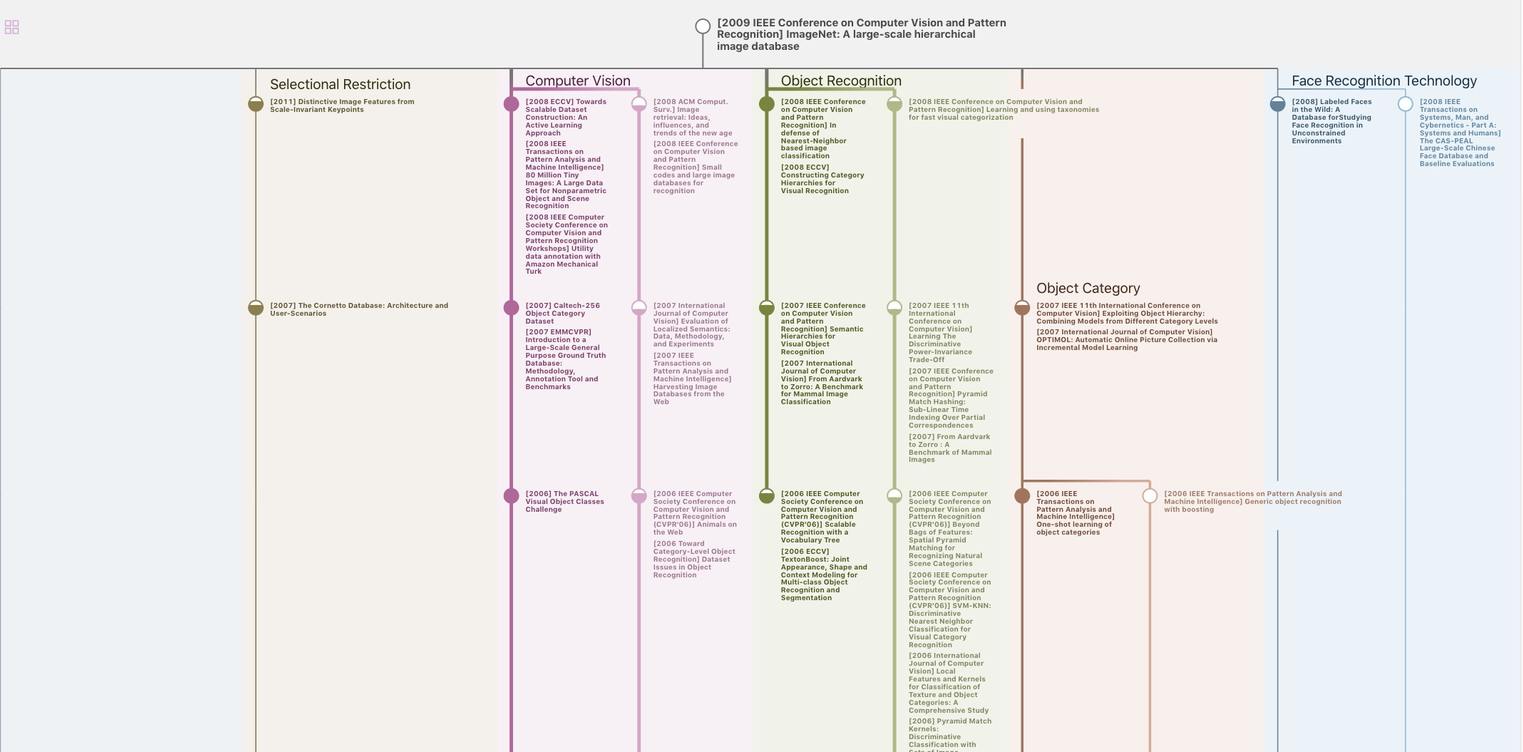
生成溯源树,研究论文发展脉络
Chat Paper
正在生成论文摘要