Autonomously Generating Hints by Inferring Problem Solving Policies
L@S(2015)
摘要
Exploring the whole sequence of steps a student takes to produce work, and the patterns that emerge from thousands of such sequences is fertile ground for a richer understanding of learning. In this paper we autonomously generate hints for the Code.org `Hour of Code,' (which is to the best of our knowledge the largest online course to date) using historical student data. We first develop a family of algorithms that can predict the way an expert teacher would encourage a student to make forward progress. Such predictions can form the basis for effective hint generation systems. The algorithms are more accurate than current state-of-the-art methods at recreating expert suggestions, are easy to implement and scale well. We then show that the same framework which motivated the hint generating algorithms suggests a sequence-based statistic that can be measured for each learner. We discover that this statistic is highly predictive of a student's future success.
更多查看译文
关键词
computer-assisted instruction,educational datamining.,hint generation,problem solving policy
AI 理解论文
溯源树
样例
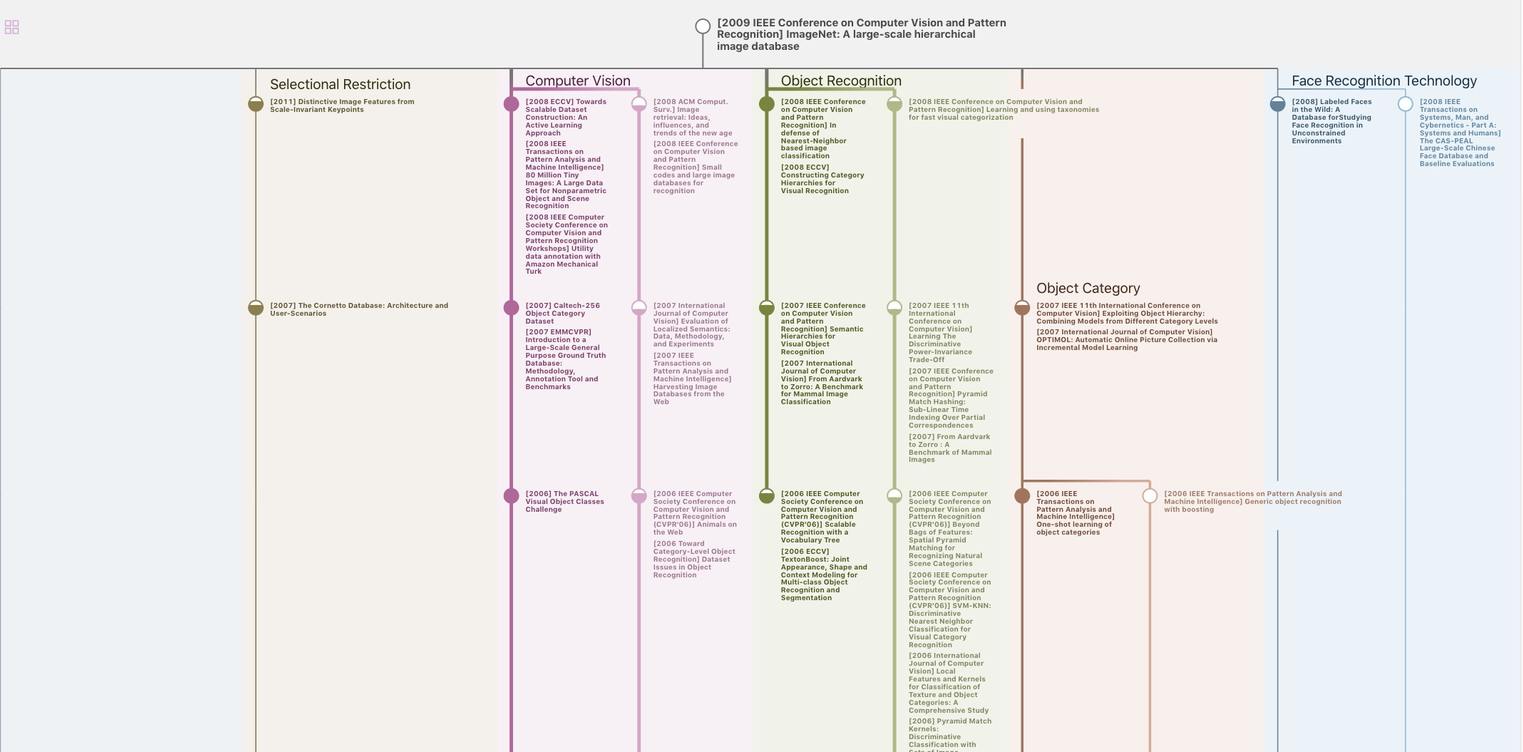
生成溯源树,研究论文发展脉络
Chat Paper
正在生成论文摘要