A Patch Aware Multiple Dictionary Framework For Demosaicing
COMPUTER VISION - ACCV 2014, PT III(2014)
摘要
Most digital cameras rely on demosaicing algorithms to restore true color images. Data captured by these cameras is reduced by two thirds because a Color Filter Array (CFA) allows only one particular channel go through at each pixel. This paper proposes a Patch Aware Multiple Dictionary (PAMD) framework for demosaicing. Instead of using a common dictionary, multiple dictionaries are trained for different classes of signals. These class-specific dictionaries comprise a super overcomplete dictionary. The most suitable dictionary would be adaptively selected based on the patch class, determined by the Energy Exclusiveness Feature (EEF) which measures the degree of energy domination in the representation code. In this way, candidate atoms are constrained in a set of atoms with low correlations; and meanwhile, the signal would have sparser representation over this adapted dictionary than over the common one, making the fixed sample rate relatively high and thus, accomplishing satisfying restoration according to the compressive sensing theory. Extensive experiments demonstrated that PAMD outperforms traditional Single Dictionary (SD) based approach as well as leading algorithms in diffusion-based family significantly, with respect to both PSNR and visual quality. Especially, the general artifact by Bayer CFA, Moire Pattern, is dramatically reduced. Furthermore, on several images, it also significantly outperforms the state-of-the-art algorithms which are also sparse coding based but very complicated. PAMD is a general framework which can cooperate with existing demosaicing algorithms based on sparse coding.
更多查看译文
关键词
Sparse Representation, Compressive Sensing, Sparse Code, Sparsity Level, Color Filter Array
AI 理解论文
溯源树
样例
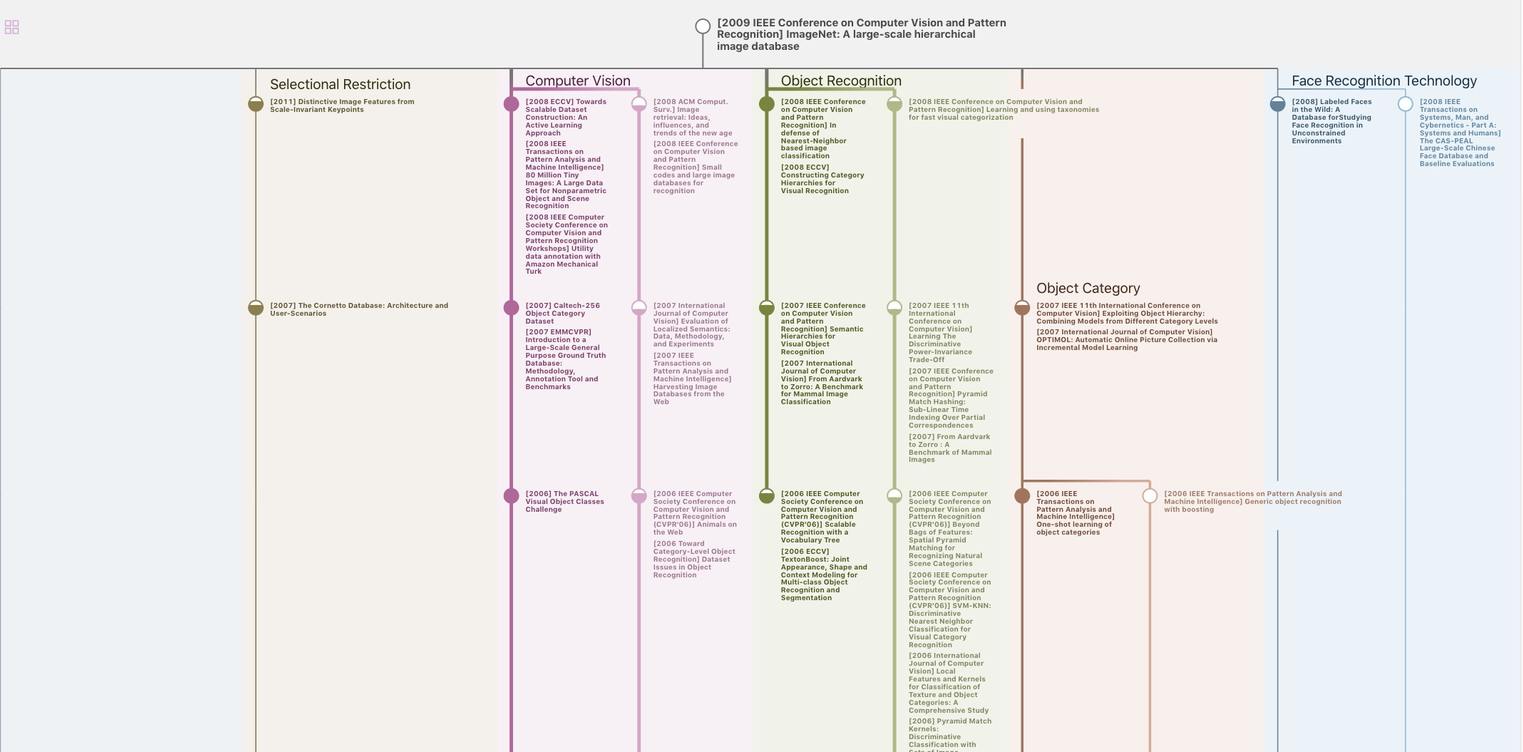
生成溯源树,研究论文发展脉络
Chat Paper
正在生成论文摘要