Group Latent Factor Model For Recommendation With Multiple User Behaviors
IR(2014)
摘要
Recently, some recommendation methods try to relieve the data sparsity problem of Collaborative Filtering by exploiting data from users' multiple types of behaviors. However, most of the exist methods mainly consider to model the correlation between different behaviors and ignore the heterogeneity of them, which may make improper information transferred and harm the recommendation results. To address this problem, we propose a novel recommendation model, named Group Latent Factor Model (GLFM), which attempts to learn a factorization of latent factor space into subspaces that are shared across multiple behaviors and subspaces that are specific to each type of behaviors. Thus, the correlation and heterogeneity of multiple behaviors can be modeled by these shared and specific latent factors. Experiments on the real-world dataset demonstrate that our model can integrate users' multiple types of behaviors into recommendation better.
更多查看译文
关键词
Recommender Systems,Matrix Factorization
AI 理解论文
溯源树
样例
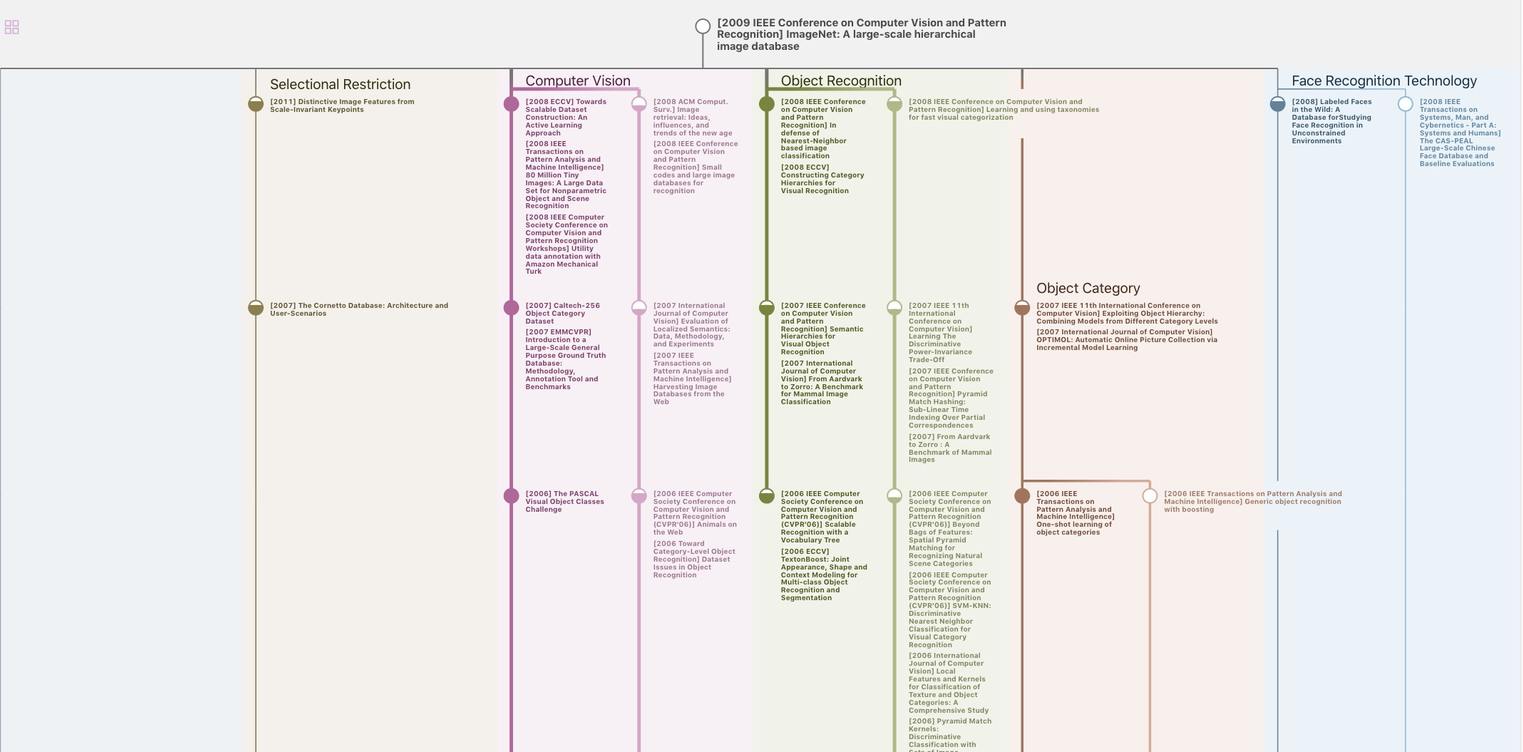
生成溯源树,研究论文发展脉络
Chat Paper
正在生成论文摘要