Coarse-To-Fine Review Selection Via Supervised Joint Aspect And Sentiment Model
IR(2014)
摘要
Online reviews are immensely valuable for customers to make informed purchase decisions and for businesses to improve the quality of their products and services. However, customer reviews grow exponentially while varying greatly in quality. It is generally very tedious and difficult, if not impossible, for users to read though the huge amount of review data for decision-making. Fortunately, review quality evaluation enables a system to recommend automatically the most helpful reviews to users. Previous studies predict only the overall review utility about a product, and often focus on developing different data features to learn a quality function for the problem. In this paper, we aim to select the most helpful reviews not only at the product level, but also at a fine-grained product aspect level. We propose a novel supervised joint aspect and sentiment model (SJASM), which is a probabilistic topic modeling framework that jointly discovers aspects and sentiments guided by a review helpfulness metric. One key advantage of SJASM is its ability to infer the underlying aspects and sentiments, which are indicative of the helpfulness of a review. We validate SJASM using publicly available review data, and our experimental results demonstrate the superiority of SJASM over several competing models.
更多查看译文
关键词
review selection,review helpfulness,supervised joint topic model,sentiment analysis
AI 理解论文
溯源树
样例
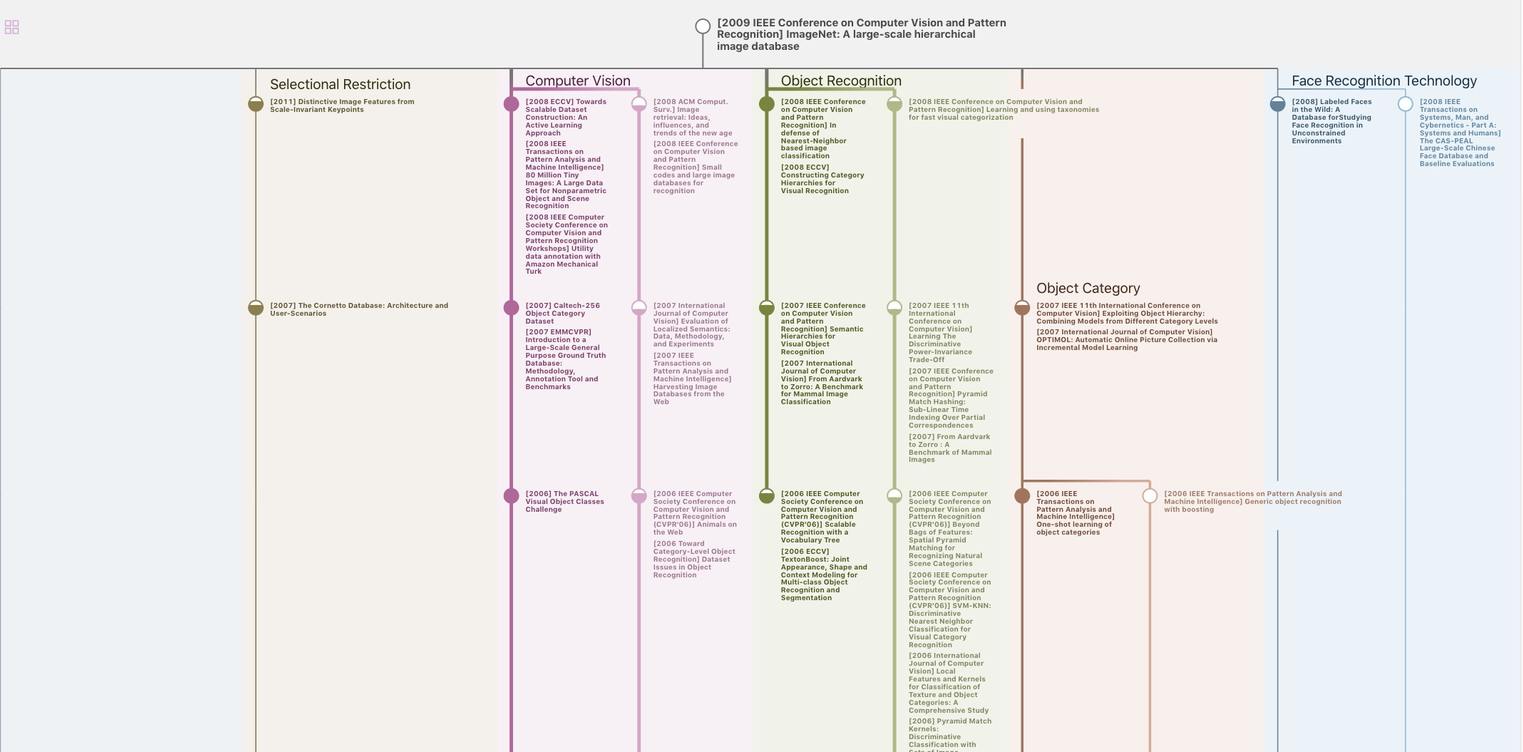
生成溯源树,研究论文发展脉络
Chat Paper
正在生成论文摘要