On Over-Specialization And Concentration Bias Of Recommendations: Probabilistic Neighborhood Selection In Collaborative Filtering Systems
RECSYS(2014)
摘要
Focusing on the problems of over-specialization and concentration bias, this paper presents a novel probabilistic method for recommending items in the neighborhood-based collaborative filtering framework. For the probabilistic neighborhood selection phase, we use an efficient method for weighted sampling of k neighbors that takes into consideration the similarity levels between the target user (or item) and the candidate neighbors. We conduct an empirical study showing that the proposed method increases the coverage, dispersion, and diversity reinforcement of recommendations by selecting diverse sets of representative neighbors. We also demonstrate that the proposed approach outperforms popular methods in terms of item prediction accuracy, utility-based ranking, and other popular measures, across various experimental settings. This performance improvement is in accordance with ensemble learning theory and the phenomenon of "hubness" in recommender systems.
更多查看译文
关键词
Collaborative Filtering,Probabilistic Neighborhood Selection,k-PN,Concentration Bias,Over-Specialization,Diversity,Mobility,Popularity Reinforcement,Long Tail
AI 理解论文
溯源树
样例
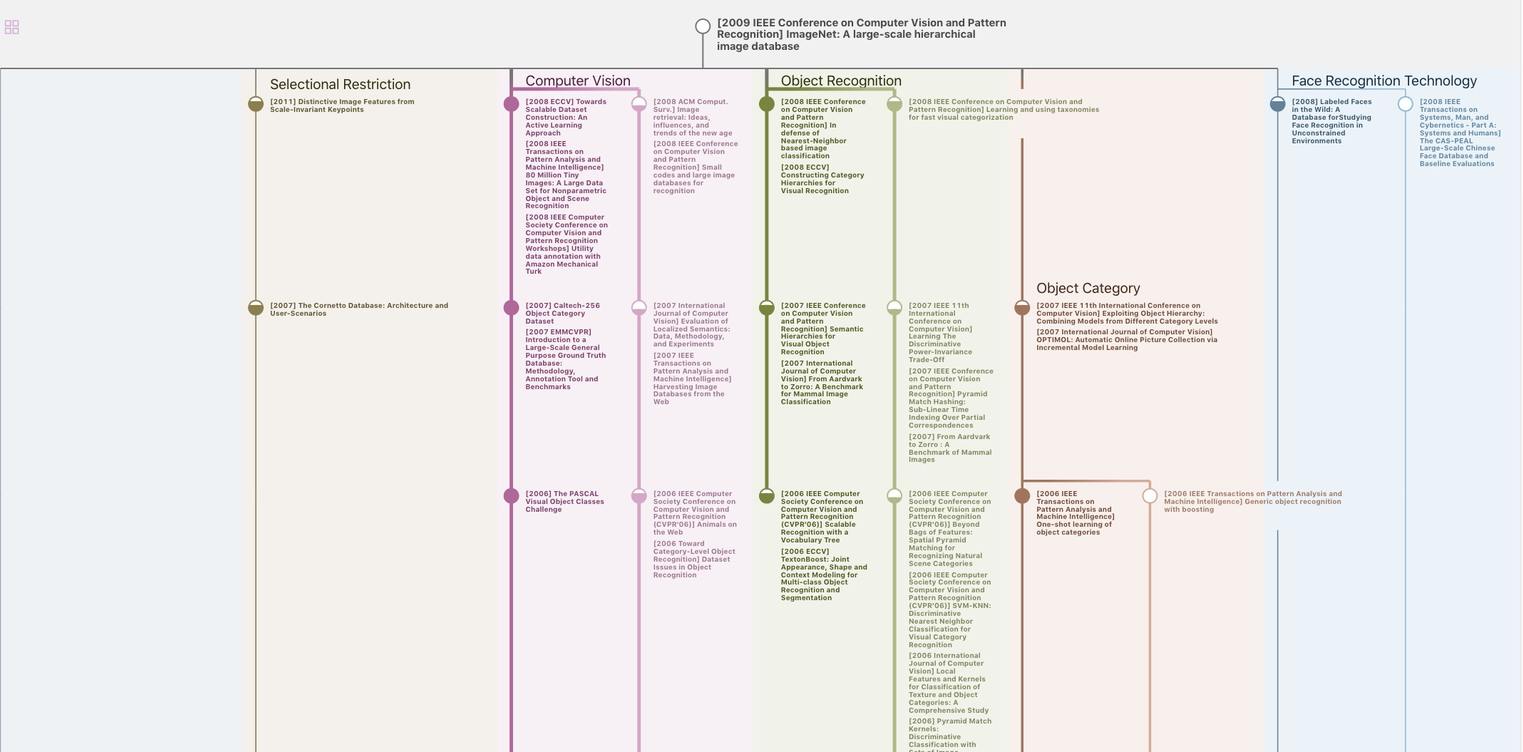
生成溯源树,研究论文发展脉络
Chat Paper
正在生成论文摘要