Question Recommendation For Collaborative Question Answering Systems With Rankslda
RECSYS(2014)
摘要
Collaborative question answering (CQA) communities rely on user participation for their success. This paper presents a supervised Bayesian approach to model expertise in online CQA communities with application to question recommendation, aimed at reducing waiting times for responses and avoiding question starvation. We propose a novel algorithm called RankSLDA which extends the supervised Latent Dirichlet Allocation model by considering a learning-to-rank paradigm. This allows us to exploit the inherent collaborative effects that are present in CQA communities where users tend to answer questions in their topics of expertise. Users can thus be modeled on the basis of the topics in which they demonstrate expertise. In the supervised stage of the method we model the pairwise order of expertise of users on a given question. We compare RankSLDA against several alternative methods on data from the Cross Validate community, part of the Stack Exchange network. RankSLDA outperforms all alternative methods by a significant margin.
更多查看译文
关键词
Learning to Rank,Supervised Latent Dirichlet Allocation,Latent Topics Model,Question Recommendation,Community Question Answering,Expert Modeling
AI 理解论文
溯源树
样例
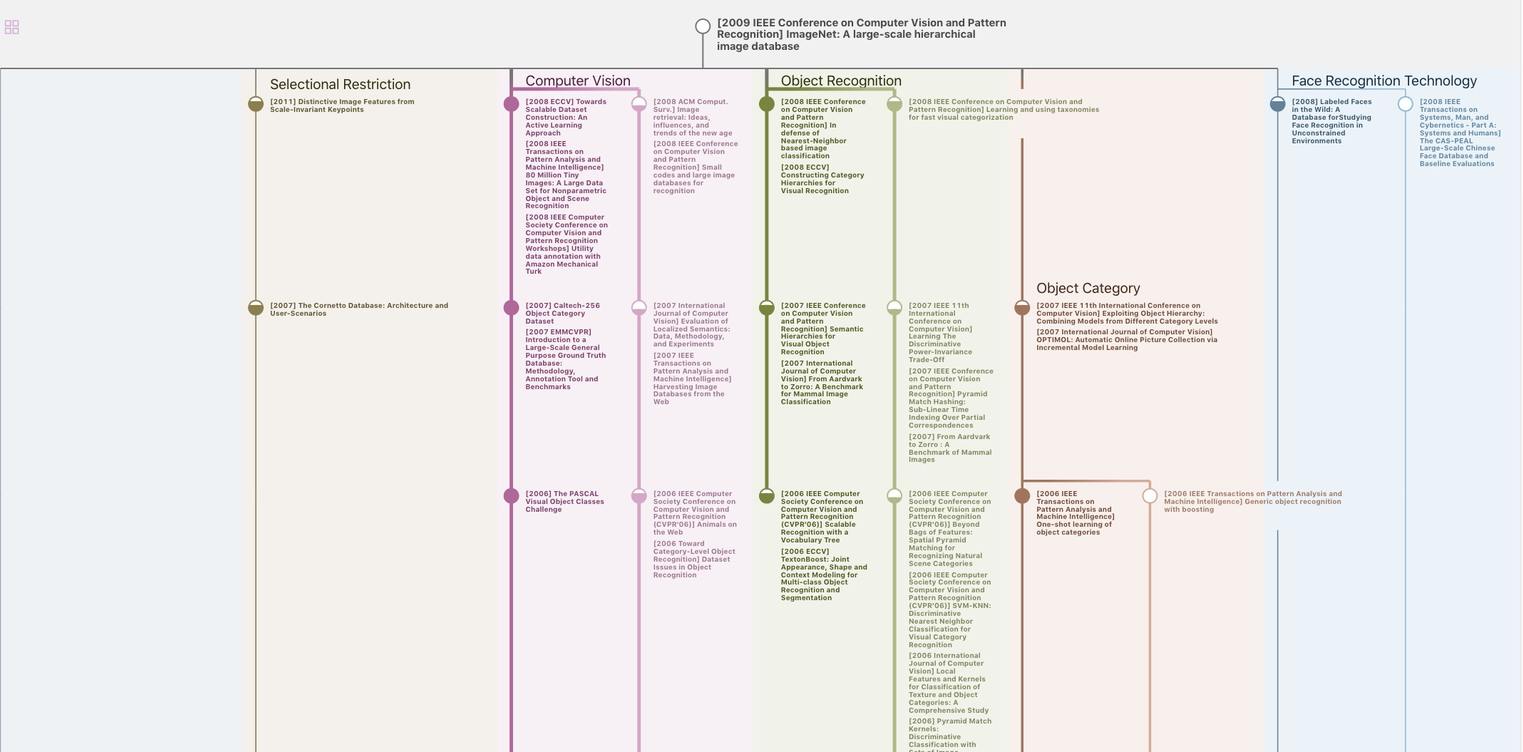
生成溯源树,研究论文发展脉络
Chat Paper
正在生成论文摘要