On-line learning of parametric mixture models for light transport simulation
ACM Trans. Graph.(2014)
摘要
Monte Carlo techniques for light transport simulation rely on importance sampling when constructing light transport paths. Previous work has shown that suitable sampling distributions can be recovered from particles distributed in the scene prior to rendering. We propose to represent the distributions by a parametric mixture model trained in an on-line (i.e. progressive) manner from a potentially infinite stream of particles. This enables recovering good sampling distributions in scenes with complex lighting, where the necessary number of particles may exceed available memory. Using these distributions for sampling scattering directions and light emission significantly improves the performance of state-of-the-art light transport simulation algorithms when dealing with complex lighting.
更多查看译文
关键词
light transport simulation,on-line expectation maximization,importance sampling,parametric density estimation,display algorithms
AI 理解论文
溯源树
样例
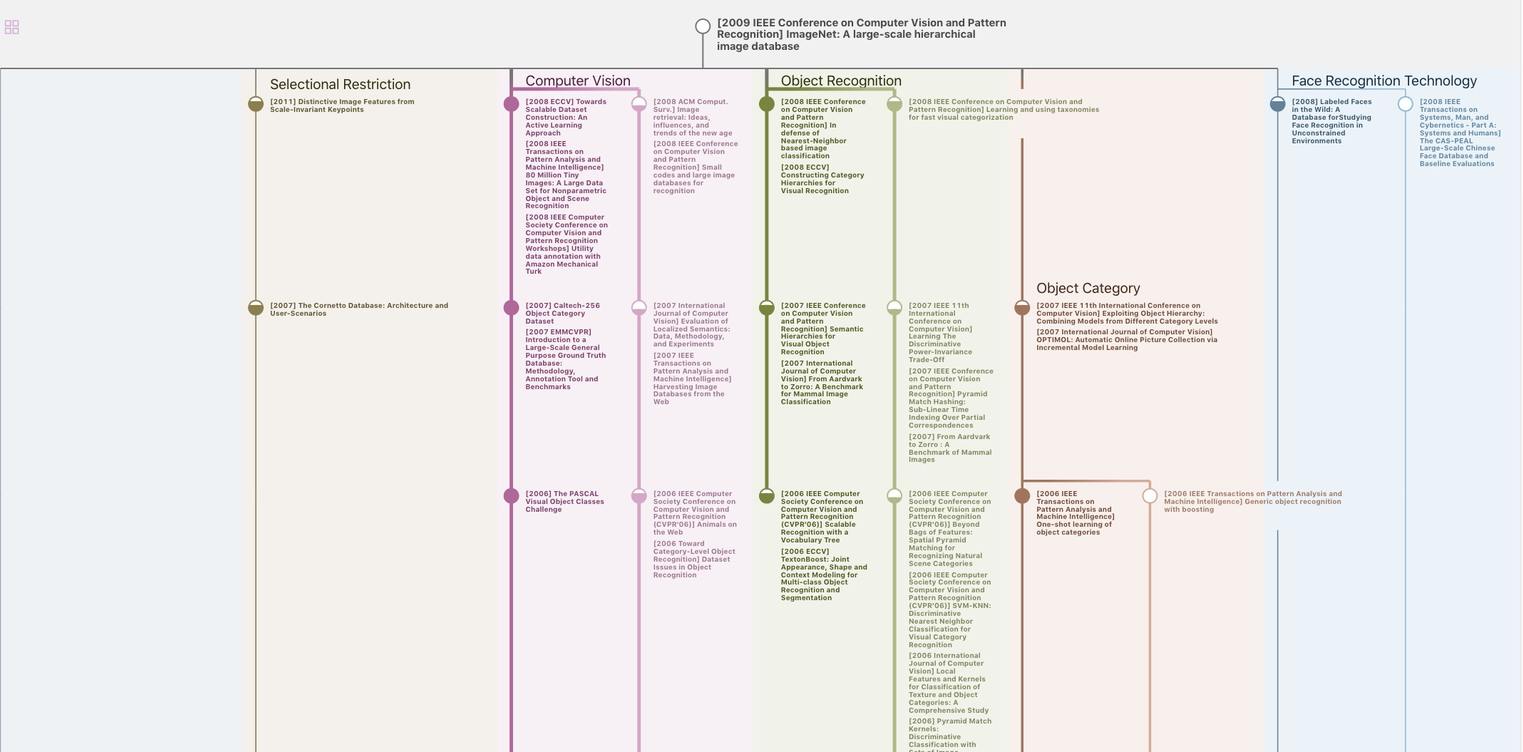
生成溯源树,研究论文发展脉络
Chat Paper
正在生成论文摘要