Automatic semantic modeling of indoor scenes from low-quality RGB-D data using contextual information
ACM Trans. Graph.(2014)
摘要
We present a novel solution to automatic semantic modeling of indoor scenes from a sparse set of low-quality RGB-D images. Such data presents challenges due to noise, low resolution, occlusion and missing depth information. We exploit the knowledge in a scene database containing 100s of indoor scenes with over 10,000 manually segmented and labeled mesh models of objects. In seconds, we output a visually plausible 3D scene, adapting these models and their parts to fit the input scans. Contextual relationships learned from the database are used to constrain reconstruction, ensuring semantic compatibility between both object models and parts. Small objects and objects with incomplete depth information which are difficult to recover reliably are processed with a two-stage approach. Major objects are recognized first, providing a known scene structure. 2D contour-based model retrieval is then used to recover smaller objects. Evaluations using our own data and two public datasets show that our approach can model typical real-world indoor scenes efficiently and robustly.
更多查看译文
关键词
model retrieval,semantic modeling,3d scenes,indoor scenes,three-dimensional graphics and realism,part assembly
AI 理解论文
溯源树
样例
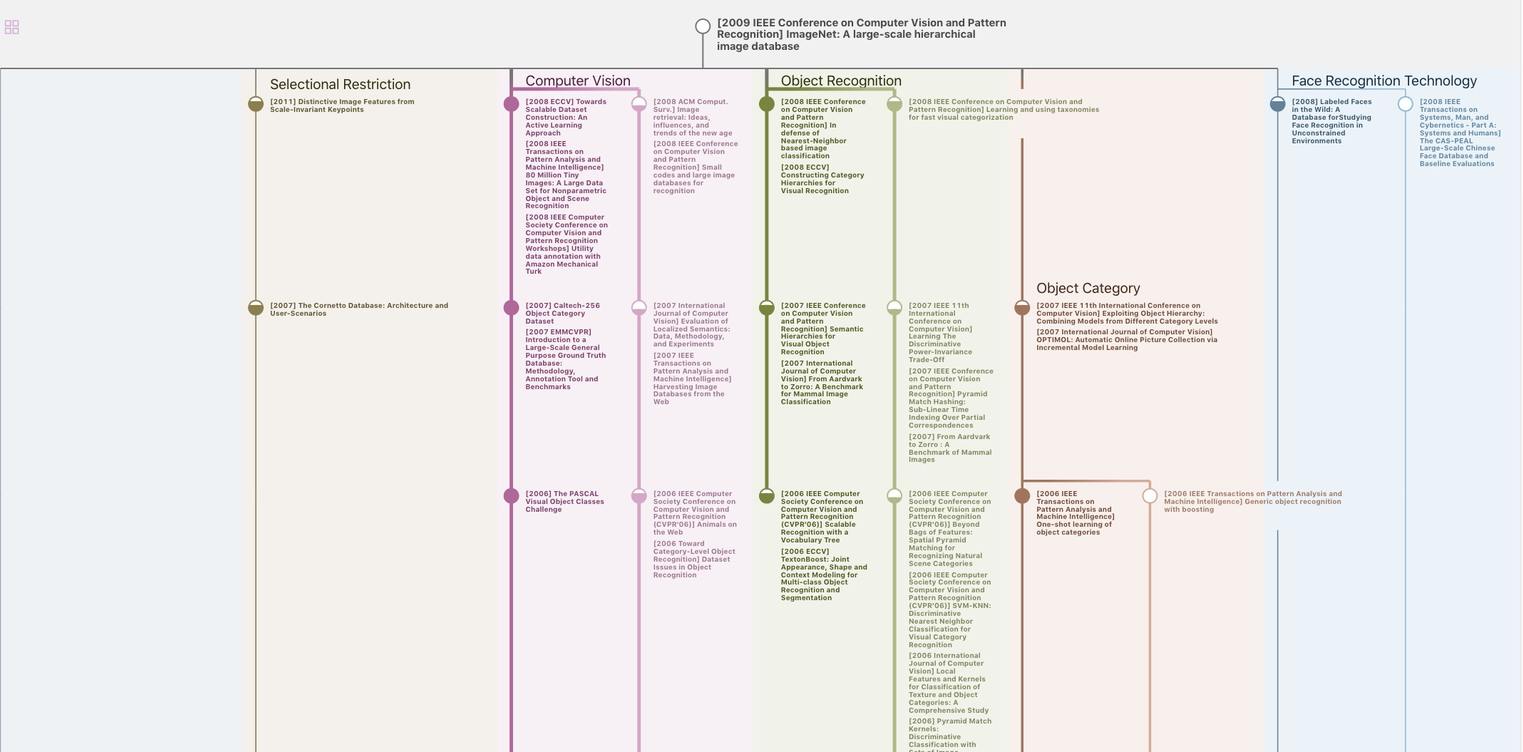
生成溯源树,研究论文发展脉络
Chat Paper
正在生成论文摘要