Ensemble learning methods for pay-per-click campaign management.
Expert Syst. Appl.(2015)
摘要
We propose using ensemble techniques to improve pay-per-click campaign management.Actual data containing pay-per-click campaign results is used for our analysis.Four base classifiers are analyzed as inputs to four ensemble modeling techniques.A MetaCost ensemble predicted the highest campaign portfolio profit. Sponsored search advertising has become a successful channel for advertisers as well as a profitable business model for the leading commercial search engines. There is an extensive sponsored search research stream regarding the classification and prediction of performance metrics such as clickthrough rate, impression rate, average results page position and conversion rate. However, there is limited research on the application of advanced data mining techniques, such as ensemble learning, to pay per click campaign classification. This research presents an in-depth analysis of sponsored search advertising campaigns by comparing the classification results from four base classification models (Naïve Bayes, logistic regression, decision trees, and Support Vector Machines) with four popular ensemble learning techniques (Voting, Boot Strap Aggregation, Stacked Generalization, and MetaCost). The goal of our research is to determine whether ensemble learning techniques can predict profitable pay-per-click campaigns and hence increase the profitability of the overall portfolio of campaigns when compared to standard classifiers. We found that the ensemble learning methods were superior classifiers based on a profit per campaign evaluation criterion. This paper extends the research on applied ensemble methods with respect to sponsored search advertising.
更多查看译文
关键词
classification,ensemble modeling
AI 理解论文
溯源树
样例
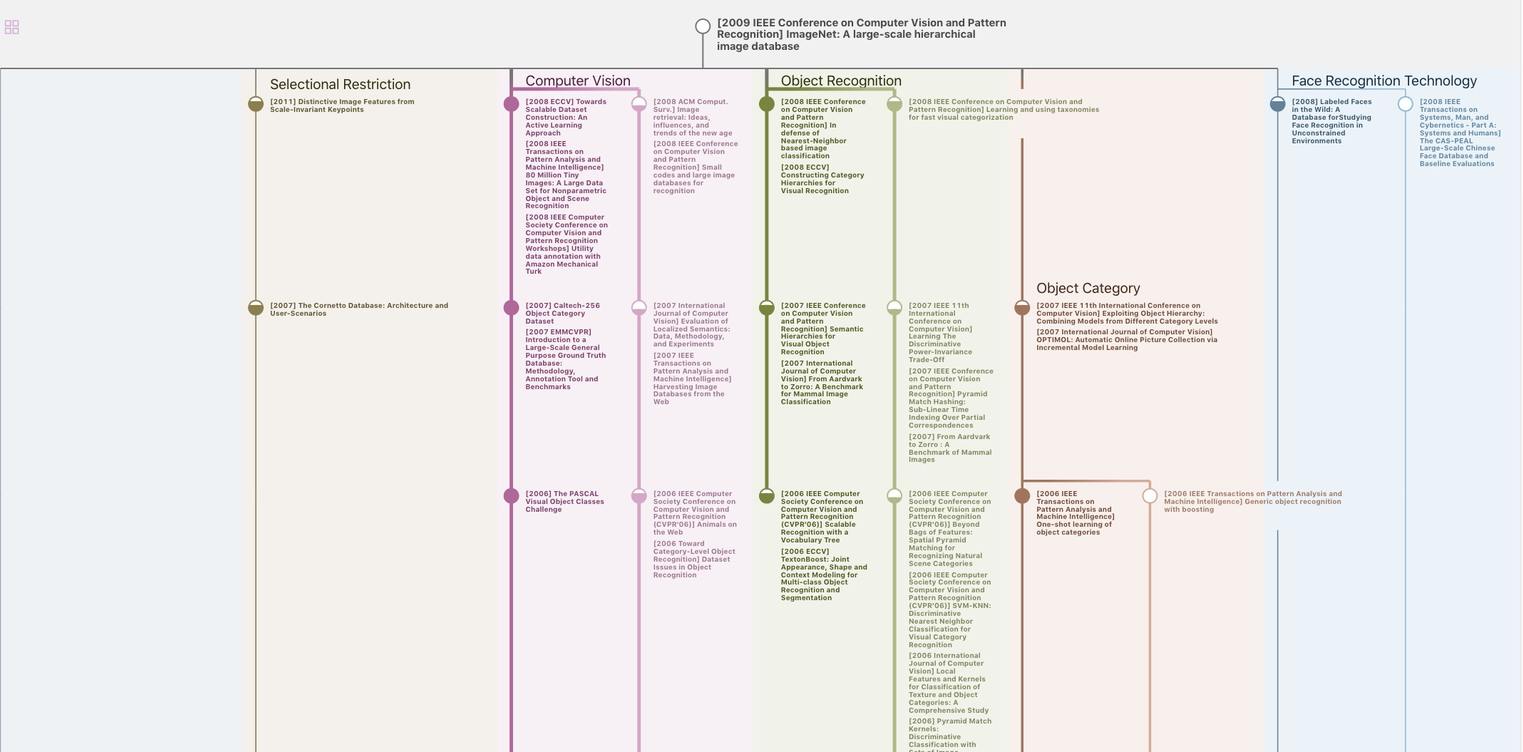
生成溯源树,研究论文发展脉络
Chat Paper
正在生成论文摘要