A knowledge-intensive approach to process similarity calculation.
Expert Syst. Appl.(2015)
摘要
Our framework support process mining and process comparison.A novel, knowledge-intensive metric for process comparison is proposed.The metric exploits domain knowledge and mined information.The metric takes into account complex control flow information.Experiments show that our metric outperforms previous literature contributions. Process model comparison and similar processes retrieval are key issues to be addressed in many real world situations, and particularly relevant ones in some applications (e.g., in medicine), where similarity quantification can be exploited in a quality assessment perspective.Most of the process comparison techniques described in the literature suffer from two main limitations: (1) they adopt a purely syntactic (vs. semantic) approach in process activity comparison, and/or (2) they ignore complex control flow information (i.e., other than sequence). These limitations oversimplify the problem, and make the results of similarity-based process retrieval less reliable, especially when domain knowledge is available, and can be adopted to quantify activity or control flow construct differences.In this paper, we aim at overcoming both limitations, by introducing a framework which allows to extract the actual process model from the available process execution traces, through process mining techniques, and then to compare (mined) process models, by relying on a novel distance measure.The novel distance measure, which represents the main contribution of this paper, is able to address issues (1) and (2) above, since: (1) it provides a semantic, knowledge-intensive approach to process activity comparison, by making use of domain knowledge; (2) it explicitly takes into account complex control flow constructs (such as AND and XOR splits/joins), thus fully considering the different semantic meaning of control flow connections in a reliable way.The positive impact of the framework in practice has been tested in stroke management, where our approach has outperformed a state-of-the art literature metric on a real world event log, providing results that were closer to those of a human expert. Experiments in other domains are foreseen in the future.
更多查看译文
关键词
process mining
AI 理解论文
溯源树
样例
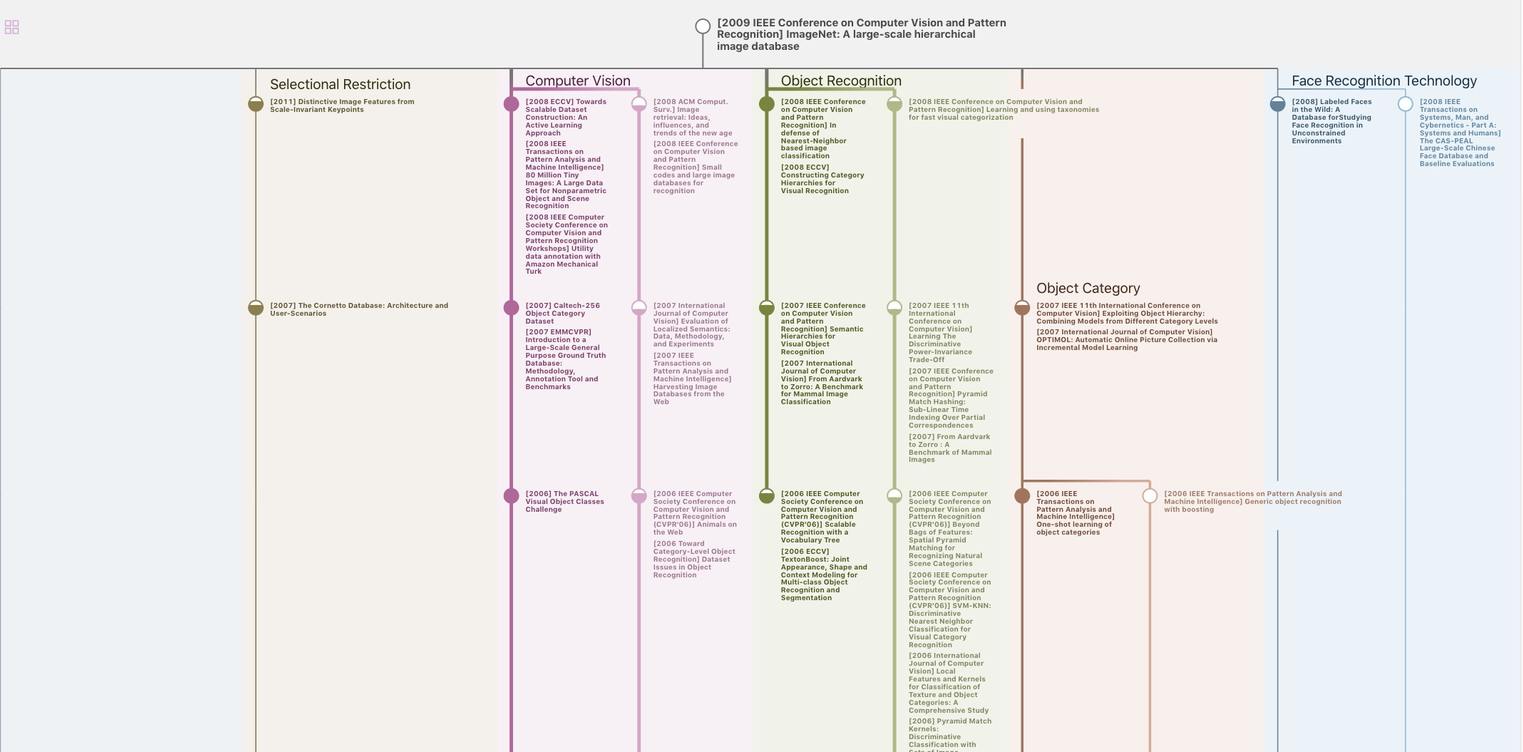
生成溯源树,研究论文发展脉络
Chat Paper
正在生成论文摘要