Nonnegative Matrix and Tensor Factorizations : An algorithmic perspective
IEEE Signal Process. Mag.(2014)
摘要
A common thread in various approaches for model reduction, clustering, feature extraction, classification, and blind source separation (BSS) is to represent the original data by a lower-dimensional approximation obtained via matrix or tensor (multiway array) factorizations or decompositions. The notion of matrix/tensor factorizations arises in a wide range of important applications and each matrix/tensor factorization makes different assumptions regarding component (factor) matrices and their underlying structures. So choosing the appropriate one is critical in each application domain. Approximate low-rank matrix and tensor factorizations play fundamental roles in enhancing the data and extracting latent (hidden) components.
更多查看译文
关键词
tensor factorization,pattern clustering,approximation theory,statistical analysis,pattern classification,feature extraction,blind source separation,matrix decomposition,rank matrix approximation,data enhancement,tensors,model reduction,nonnegative matrix,latent component extraction,sparse matrices,tensile stress,clustering
AI 理解论文
溯源树
样例
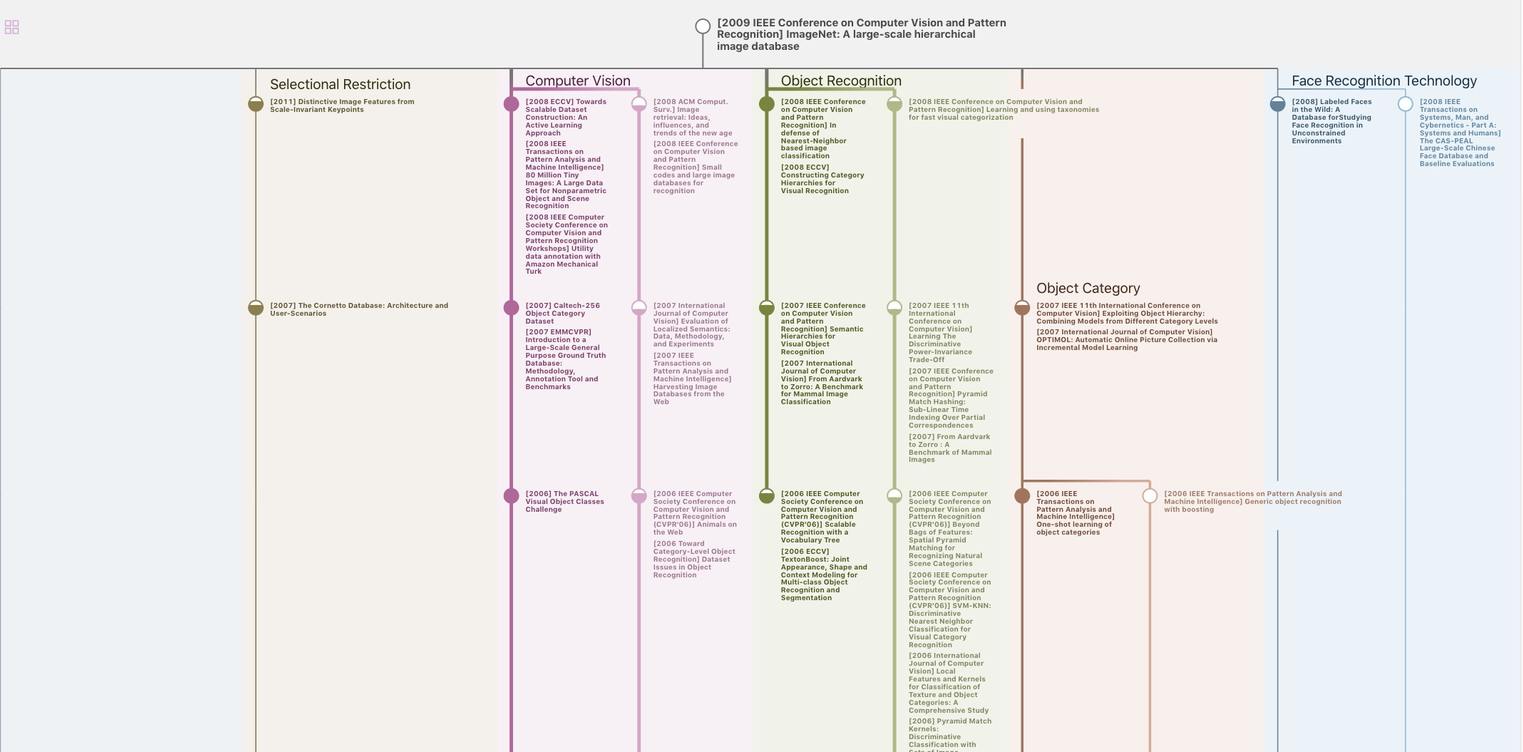
生成溯源树,研究论文发展脉络
Chat Paper
正在生成论文摘要