Empirical Mode Decomposition and Rough Set Attribute Reduction for Ultrasonic Flaw Signal Classification
INTERNATIONAL JOURNAL OF COMPUTATIONAL INTELLIGENCE SYSTEMS(2014)
摘要
Feature extraction and selection are the most important techniques for ultrasonic flaw signal classification. In this study, empirical mode decomposition (EMD) is used to obtain the intrinsic mode functions (IMFs) of original signal, and their corresponding traditional time and frequency domain based statistical parameters are extracted as the initial features. After that, spectral clustering method is used for feature value discretization so that rough set attribute reduction (RSAR) can be applied to implement feature selection. The final features are taken as input of artificial neural networks (ANNs) to train the decision classifier for flaw identification. Experimental results show that compared to conventional wavelet transform based schemes and principal components analysis, EMD combined with RSAR can improve the performance of feature extraction and selection. ANN by using such scheme can effectively classify different ultrasonic flaw signals with high accuracy and low training elapsed time.
更多查看译文
关键词
empirical mode decomposition, rough set attribute reduction, feature extraction and selection, ultrasonic flaw signal classification
AI 理解论文
溯源树
样例
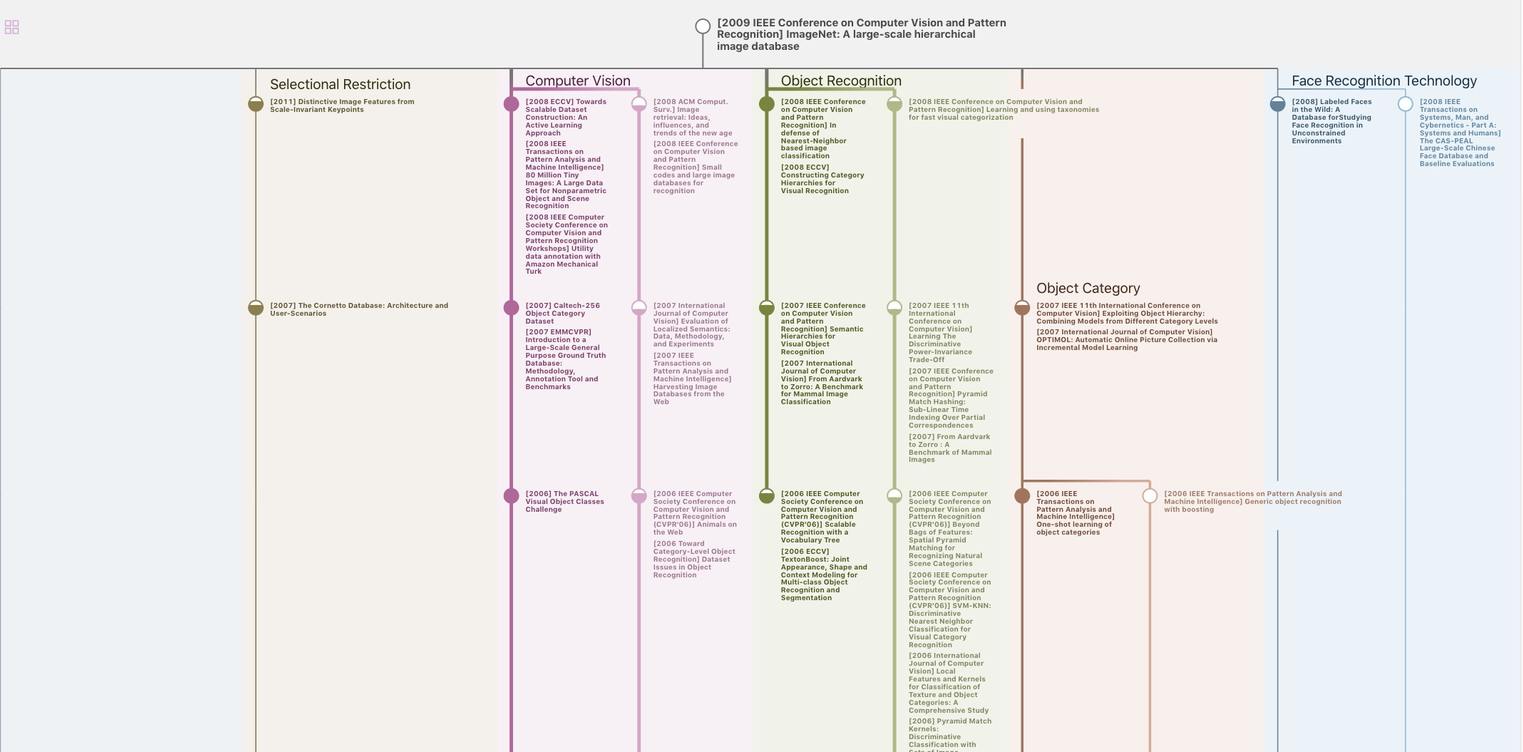
生成溯源树,研究论文发展脉络
Chat Paper
正在生成论文摘要