Clothing Attributes Assisted Person Reidentification
IEEE Trans. Circuits Syst. Video Techn.(2015)
摘要
Person reidentification across nonoverlapping camera views is a rather challenging task. Due to the difficulties in obtaining identifiable faces, clothing appearance becomes the main cue for identification purposes. In this paper, we present a comprehensive study on clothing attributes assisted person reidentification. First, the body parts and their local features are extracted for alleviating the pose-misalignment issue. A latent support vector machine (LSVM)-based person reidentification approach is proposed to describe the relations among the low-level part features, middle-level clothing attributes, and high-level reidentification labels of person pairs. Motivated by the uncertainties of clothing attributes, we treat them as real-value variables instead of using them as discrete variables. Moreover, a large-scale real-world dataset with 10 camera views and about 200 subjects is collected and thoroughly annotated for this paper. The extensive experiments on this dataset show: 1) part features are more effective than features extracted from the holistic human bounding boxes; 2) the clothing attributes embedded in the LSVM model may further boost reidentification performance compared with support vector machine without clothing attributes; and 3) treating clothing attributes as real-value variables is more effective than using them as discrete variables in person reidentification.
更多查看译文
关键词
computational modeling,support vector machines,clothing,feature extraction,object recognition,semantics,body parts,vectors
AI 理解论文
溯源树
样例
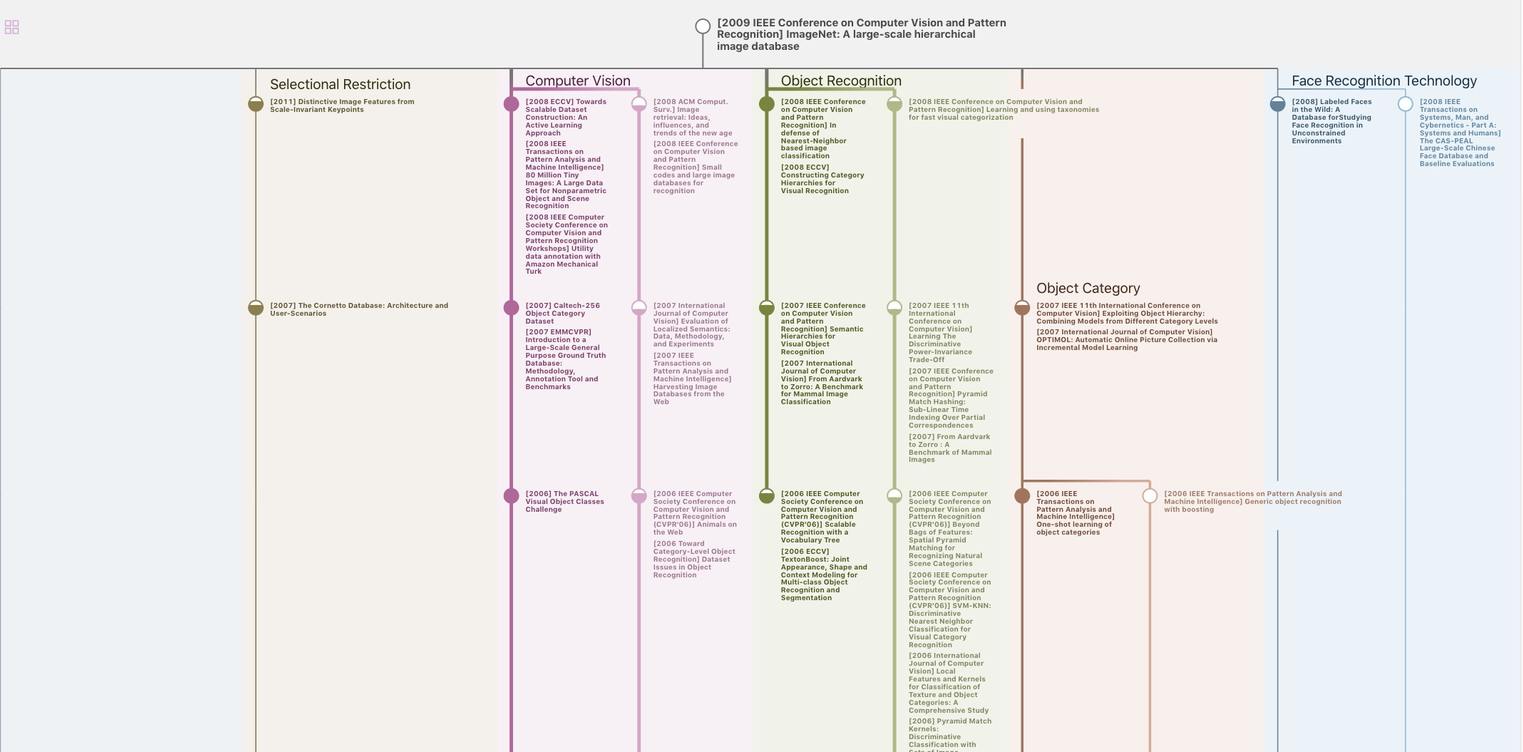
生成溯源树,研究论文发展脉络
Chat Paper
正在生成论文摘要