Feature-based automatic identification of interesting data segments in group movement data
Periodicals(2014)
摘要
AbstractThe study of movement data is an important task in a variety of domains such as transportation, biology, or finance. Often, the data objects are grouped [e.g. countries by continents]. We distinguish three main categories of movement data analysis, based on the focus of the analysis: [a] movement characteristics of an individual in the context of its group, [b] the dynamics of a given group, and [c] the comparison of the behavior of multiple groups. Examination of group movement data can be effectively supported by data analysis and visualization. In this respect, approaches based on analysis of derived movement characteristics [called features in this article] can be useful. However. current approaches are limited as they do not cover a broad range of situations and typically require manual feature monitoring. We present an enhanced set of movement analysis features and add automatic analysis of the features for filtering the interesting parts in large movement data sets. Using this approach, users can easily detect new interesting characteristics such as outliers, trends, and task-dependent data patterns even in large sets of data points over long time horizons. We demonstrate the usefulness with two real-world data sets from the socioeconomic and the financial domains.
更多查看译文
关键词
Spatiotemporal data, visual analytics, time-dependent data, movement data, group movements
AI 理解论文
溯源树
样例
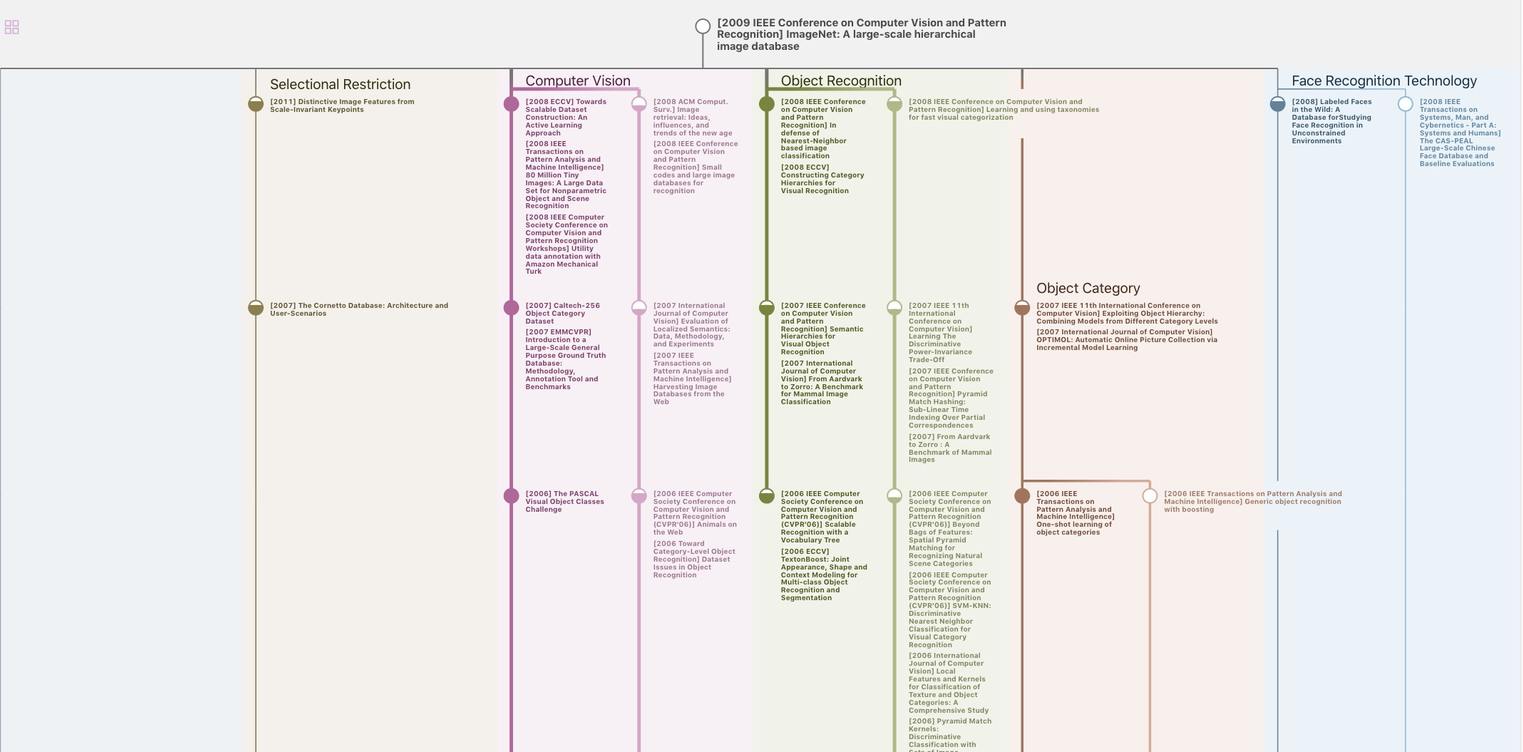
生成溯源树,研究论文发展脉络
Chat Paper
正在生成论文摘要