A Two-Stage Framework for 3D FaceReconstruction from RGBD Images
IEEE Trans. Pattern Anal. Mach. Intell.(2014)
摘要
This paper proposes a new approach for 3D face reconstruction with RGBD images from an inexpensive commodity sensor. The challenges we face are: 1) substantial random noise and corruption are present in low-resolution depth maps; and 2) there is high degree of variability in pose and face expression. We develop a novel two-stage algorithm that effectively maps low-quality depth maps to realistic face models. Each stage is targeted toward a certain type of noise. The first stage extracts sparse errors from depth patches through the data-driven local sparse coding, while the second stage smooths noise on the boundaries between patches and reconstructs the global shape by combining local shapes using our template-based surface refinement. Our approach does not require any markers or user interaction. We perform quantitative and qualitative evaluations on both synthetic and real test sets. Experimental results show that the proposed approach is able to produce high-resolution 3D face models with high accuracy, even if inputs are of low quality, and have large variations in viewpoint and face expression.
更多查看译文
关键词
non-rigid registration,two-stage algorithm,image coding,red-green-blue-depth images,face reconstruction,rigid registration,pose expression,template-based surface refinement,face expression,low-resolution depth maps,variability degree,sparse coding,rgbd images,image reconstruction,high-resolution 3d face models,data-driven local sparse coding,surface modeling,deformation transfer,3d face reconstruction,feature extraction,surface tracking,two-stage framework,sparse error extraction,commodity sensor,statistical learning,image colour analysis,face,solid modeling,databases,noise
AI 理解论文
溯源树
样例
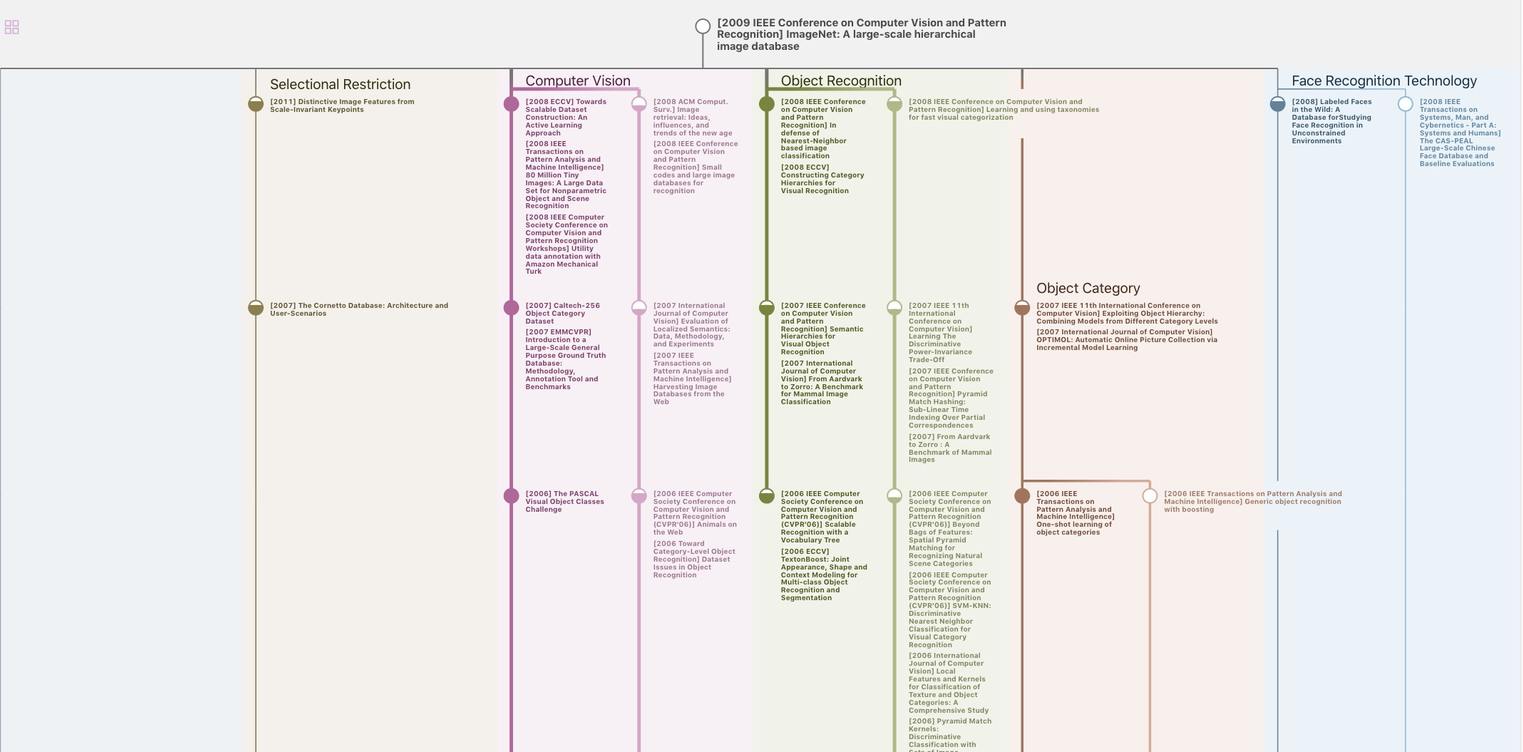
生成溯源树,研究论文发展脉络
Chat Paper
正在生成论文摘要