Generalized Sparselet Models for Real-Time Multiclass Object Recognition
IEEE Trans. Pattern Anal. Mach. Intell.(2015)
摘要
The problem of real-time multiclass object recognition is of great practical importance in object recognition. In this paper, we describe a framework that simultaneously utilizes shared representation, reconstruction sparsity, and parallelism to enable real-time multiclass object detection with deformable part models at 5Hz on a laptop computer with almost no decrease in task performance. Our framework is trained in the standard structured output prediction formulation and is generically applicable for speeding up object recognition systems where the computational bottleneck is in multiclass, multi-convolutional inference. We experimentally demonstrate the efficiency and task performance of our method on PASCAL VOC, subset of ImageNet, Caltech101 and Caltech256 dataset.
更多查看译文
关键词
multiconvolutional inference,parallelism,parallel processing,image representation,real-time multiclass object detection,object detection,caltech101 dataset,standard structured output prediction formulation,laptop computer,reconstruction sparsity,real-time vision,sparse coding,imagenet dataset,image reconstruction,frequency 5 hz,deformable part models,object recognition,generalized sparselet model,laptop computers,multiclass inference,pascal voc,caltech256 dataset,real-time multiclass object recognition,real-time systems,shared representation,vectors,real time systems,dictionaries,sparse matrices,computational modeling
AI 理解论文
溯源树
样例
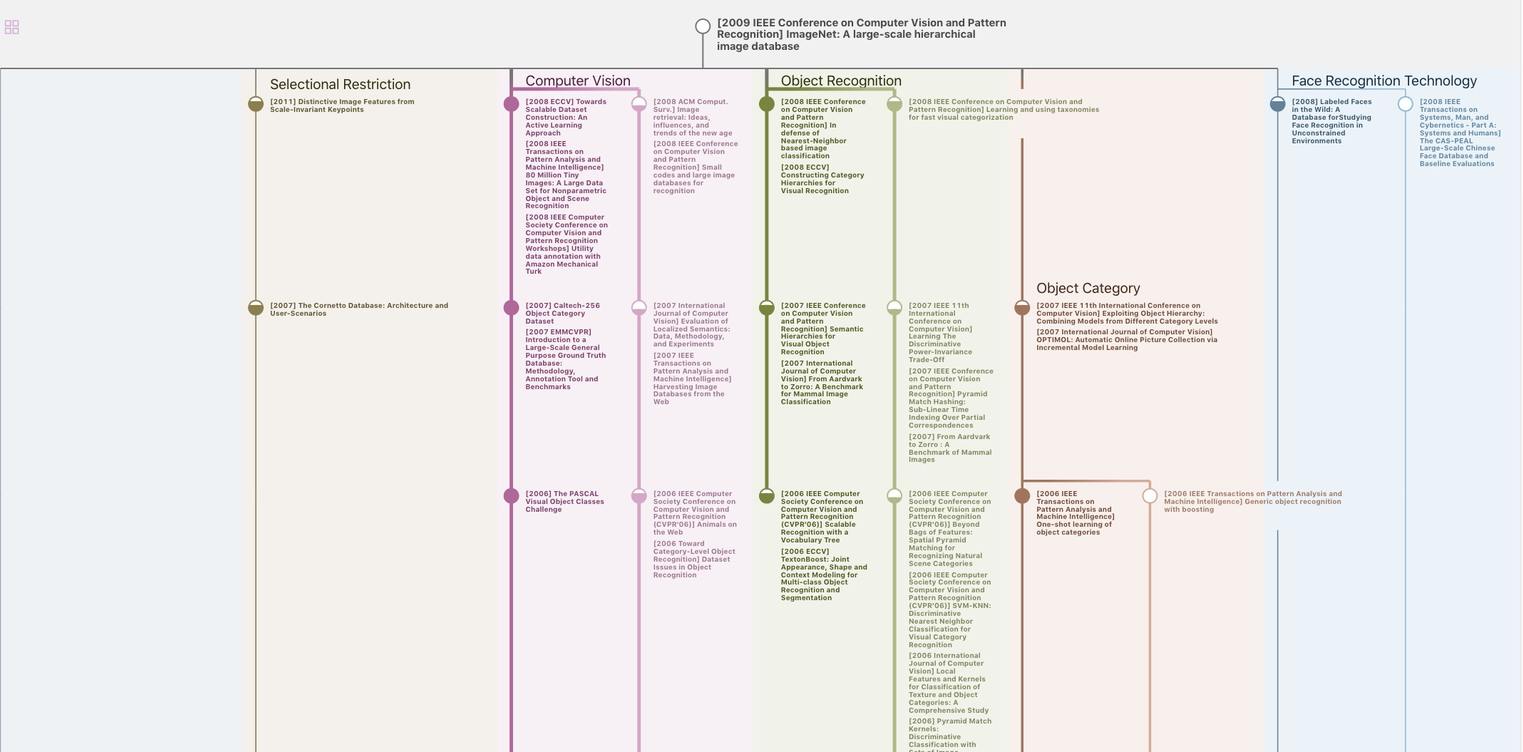
生成溯源树,研究论文发展脉络
Chat Paper
正在生成论文摘要