Randomized Dimensionality Reduction for $k$ -Means Clustering
IEEE Transactions on Information Theory(2015)
摘要
We study the topic of dimensionality reduction for k-means clustering. Dimensionality reduction encompasses the union of two approaches: 1) feature selection and 2) feature extraction. A feature selection-based algorithm for k-means clustering selects a small subset of the input features and then applies k-means clustering on the selected features. A feature extraction-based algorithm for k-means ...
更多查看译文
关键词
Feature extraction,Approximation methods,Approximation algorithms,Clustering algorithms,Optimized production technology,Vectors,Linear matrix inequalities
AI 理解论文
溯源树
样例
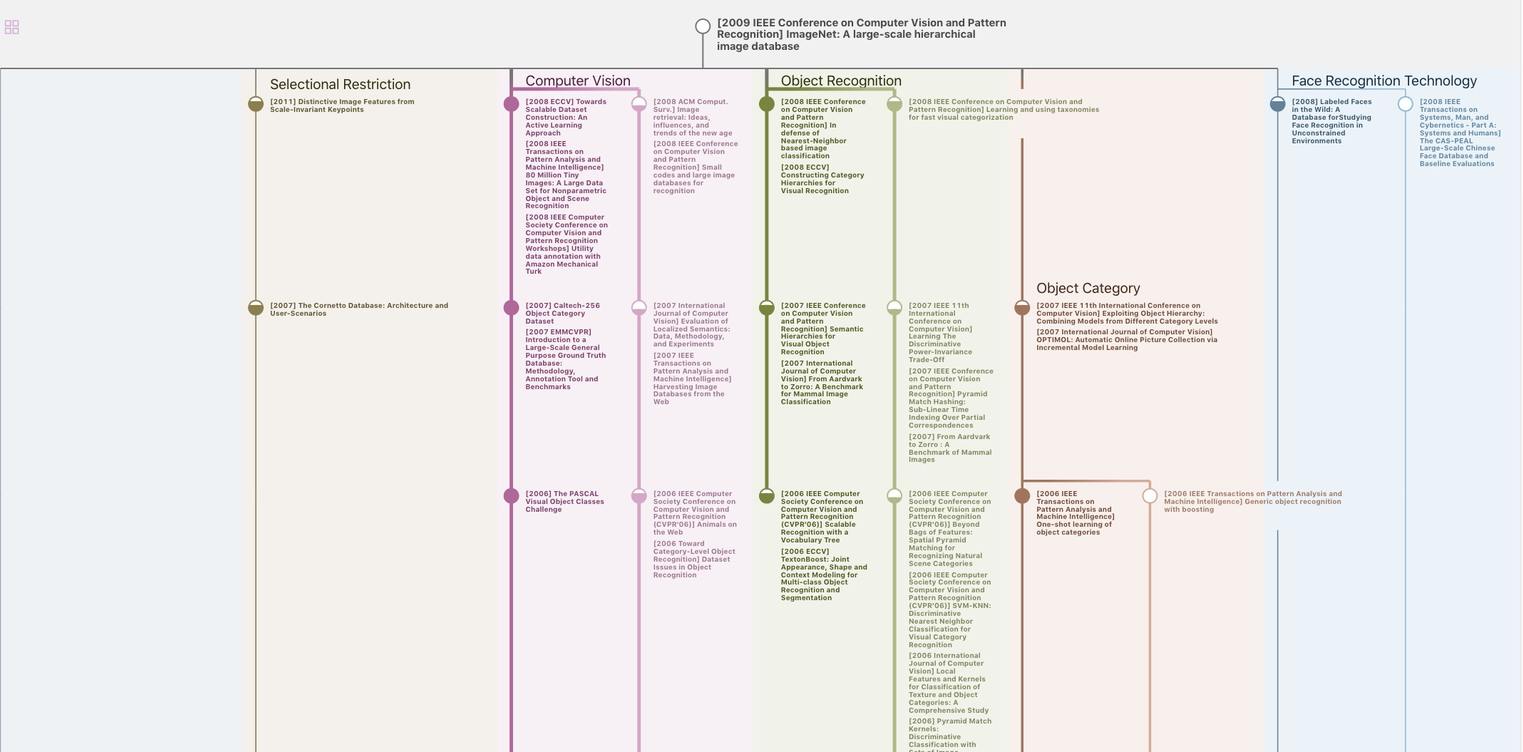
生成溯源树,研究论文发展脉络
Chat Paper
正在生成论文摘要