The Convergence Guarantees of a Non-Convex Approach for Sparse Recovery
IEEE Transactions on Signal Processing(2014)
摘要
In the area of sparse recovery, numerous researches hint that non-convex penalties might induce better sparsity than convex ones, but up until now those corresponding non-convex algorithms lack convergence guarantees from the initial solution to the global optimum. This paper aims to provide performance guarantees of a non-convex approach for sparse recovery. Specifically, the concept of weak convexity is incorporated into a class of sparsity-inducing penalties to characterize the non-convexity. Borrowing the idea of the projected subgradient method, an algorithm is proposed to solve the non-convex optimization problem. In addition, a uniform approximate projection is adopted in the projection step to make this algorithm computationally tractable for large scale problems. The convergence analysis is provided in the noisy scenario. It is shown that if the non-convexity of the penalty is below a threshold (which is in inverse proportion to the distance between the initial solution and the sparse signal), the recovered solution has recovery error linear in both the step size and the noise term. Numerical simulations are implemented to test the performance of the proposed approach and verify the theoretical analysis.
更多查看译文
关键词
sparseness measure,non-convex optimization,sparse signal,numerical analysis,theoretical analysis,convergence analysis,sparse recovery,nonconvex approach,compressed sensing,concave programming,weak convexity,nonconvex optimization problem,gradient methods,nonconvex algorithms,subgradient method,approximate projection,inverse proportion,numerical simulations,projected generalized gradient method,sparsity-inducing penalties
AI 理解论文
溯源树
样例
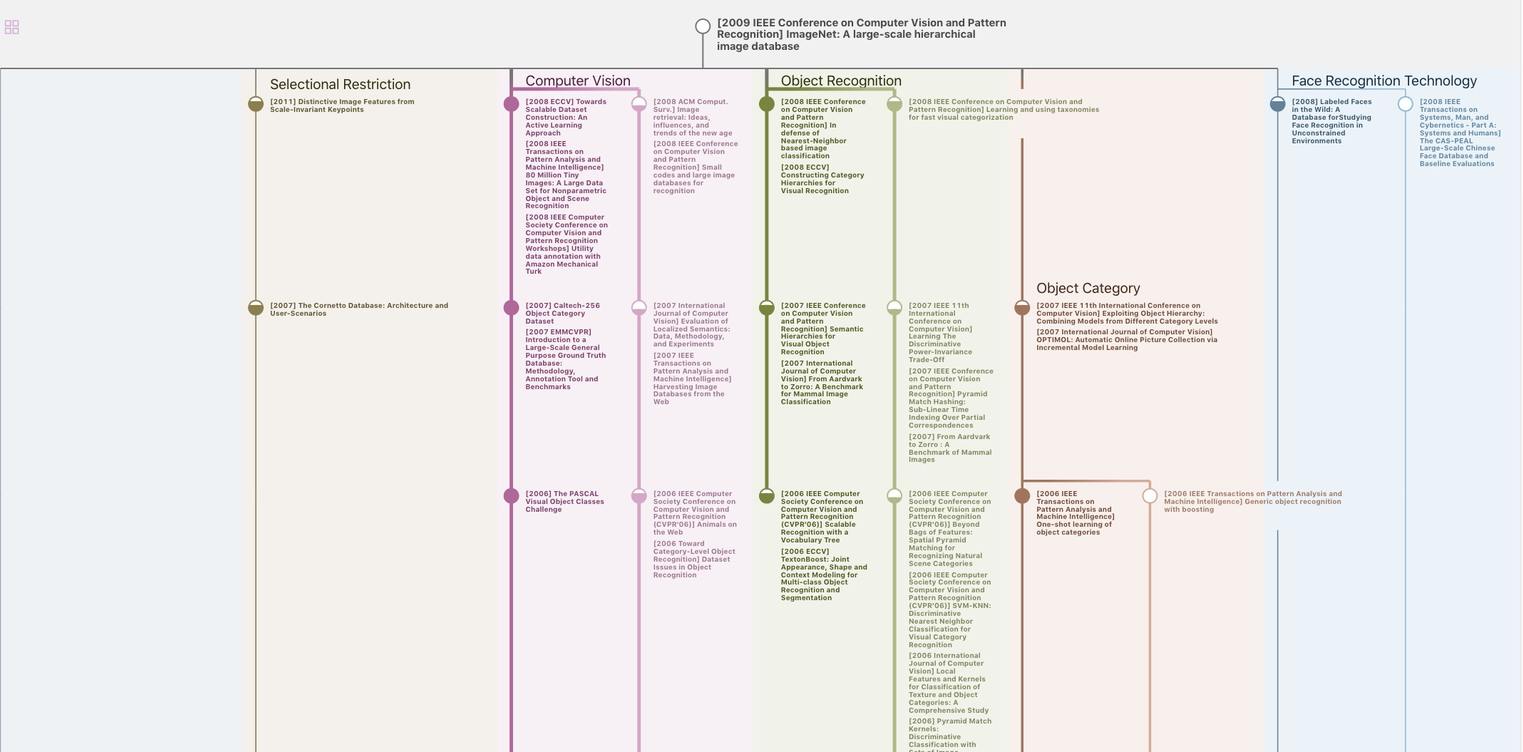
生成溯源树,研究论文发展脉络
Chat Paper
正在生成论文摘要