Likelihood Estimators for Dependent Samples and Their Application to Order Detection
IEEE Transactions on Signal Processing(2014)
摘要
Estimation of the dimension of the signal subspace, or order detection, is one of the key issues in many signal processing problems. Information theoretic criteria are widely used to estimate the order under the independently and identically distributed (i.i.d.) sampling assumption. However, in many applications, the i.i.d. sampling assumption does not hold. Previous approaches address the dependent sample issue by downsampling the data set so that existing order detection methods can be used. By discarding data, the sample size is decreased causing degradation in the accuracy of the order estimation. In this paper, we introduce two likelihood estimators for dependent samples based on two signal models. The likelihood for each signal model is developed based on the entire data set and used in an information theoretic framework to achieve reliable order estimation performance for dependent samples. Experimental results show the desirable performance of the new method.
更多查看译文
关键词
entropy rate,reliable order estimation performance,distributed sampling assumption,information theoretic framework,signal processing,signal processing problems,maximum likelihood estimation,likelihood estimators,minimum description length,signal subspace,order detection,signal models,information theoretic criteria
AI 理解论文
溯源树
样例
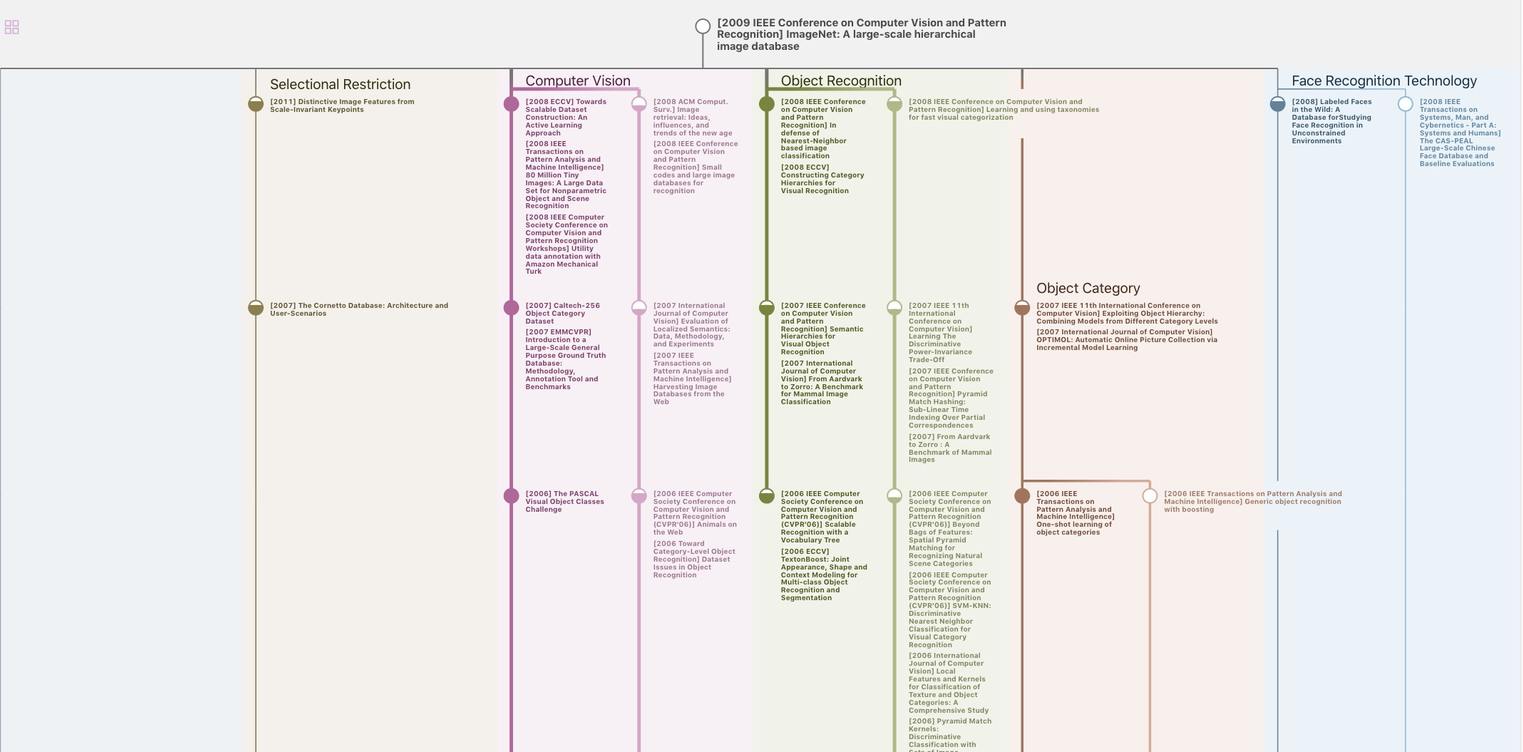
生成溯源树,研究论文发展脉络
Chat Paper
正在生成论文摘要