Sparse Representation Based Fisher Discrimination Dictionary Learning for Image Classification
International Journal of Computer Vision(2014)
摘要
The employed dictionary plays an important role in sparse representation or sparse coding based image reconstruction and classification, while learning dictionaries from the training data has led to state-of-the-art results in image classification tasks. However, many dictionary learning models exploit only the discriminative information in either the representation coefficients or the representation residual, which limits their performance. In this paper we present a novel dictionary learning method based on the Fisher discrimination criterion. A structured dictionary, whose atoms have correspondences to the subject class labels, is learned, with which not only the representation residual can be used to distinguish different classes, but also the representation coefficients have small within-class scatter and big between-class scatter. The classification scheme associated with the proposed Fisher discrimination dictionary learning (FDDL) model is consequently presented by exploiting the discriminative information in both the representation residual and the representation coefficients. The proposed FDDL model is extensively evaluated on various image datasets, and it shows superior performance to many state-of-the-art dictionary learning methods in a variety of classification tasks.
更多查看译文
关键词
Dictionary learning,Sparse representation,Fisher criterion,Image classification
AI 理解论文
溯源树
样例
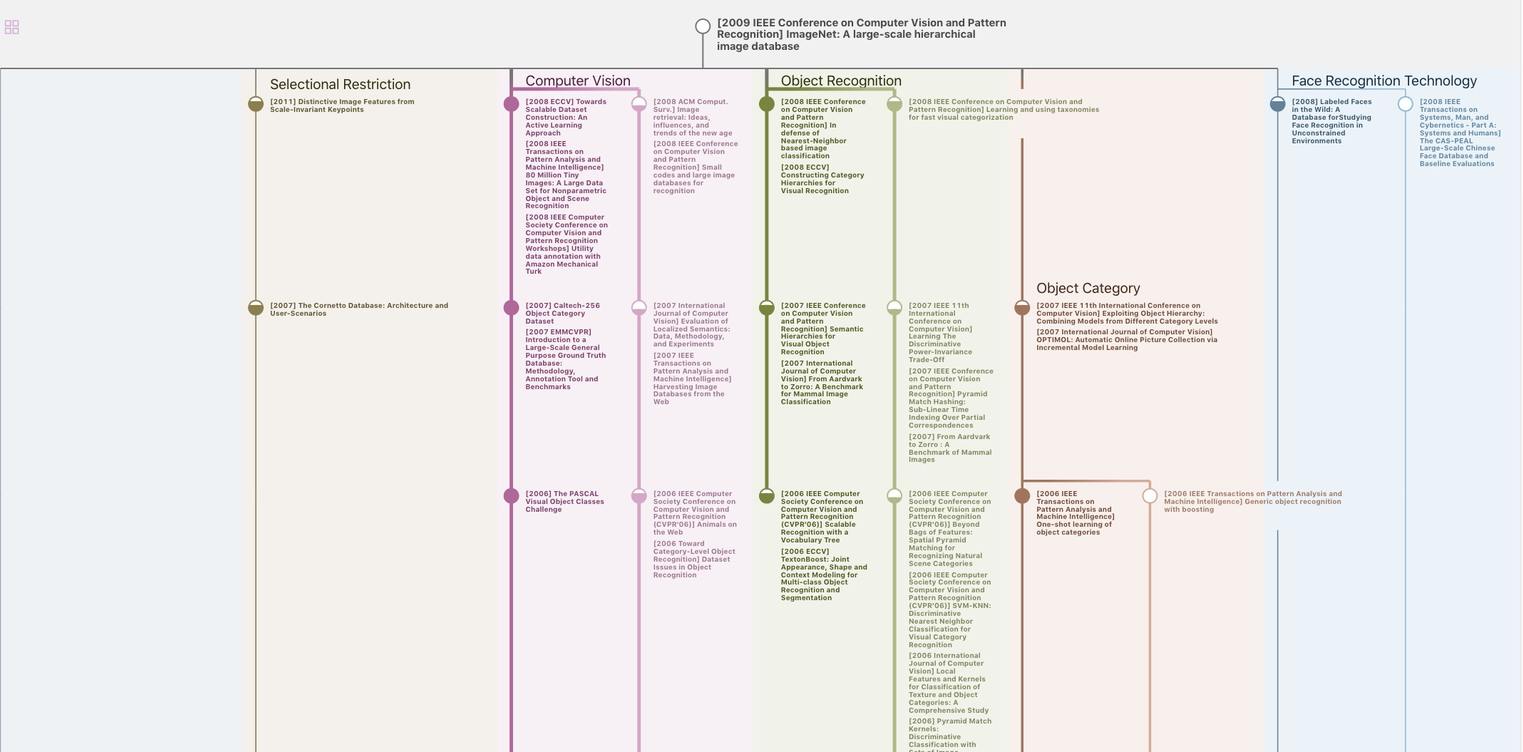
生成溯源树,研究论文发展脉络
Chat Paper
正在生成论文摘要