Unsupervised Learning of Spatiotemporally Coherent Metrics
2015 IEEE International Conference on Computer Vision (ICCV)(2015)
摘要
Current state-of-the-art classification and detection algorithms rely on supervised training. In this work we study unsupervised feature learning in the context of temporally coherent video data. We focus on feature learning from unlabeled video data, using the assumption that adjacent video frames contain semantically similar information. This assumption is exploited to train a convolutional pooling auto-encoder regularized by slowness and sparsity. We establish a connection between slow feature learning to metric learning and show that the trained encoder can be used to define a more temporally and semantically coherent metric.
更多查看译文
关键词
spatiotemporally coherent metrics,detection algorithms,classification algorithms,deep convolutional networks,labeled data,unsupervised feature learning,video data,video frames,convolutional pooling auto-encoder,metric learning,hyperparameters,transfer learning
AI 理解论文
溯源树
样例
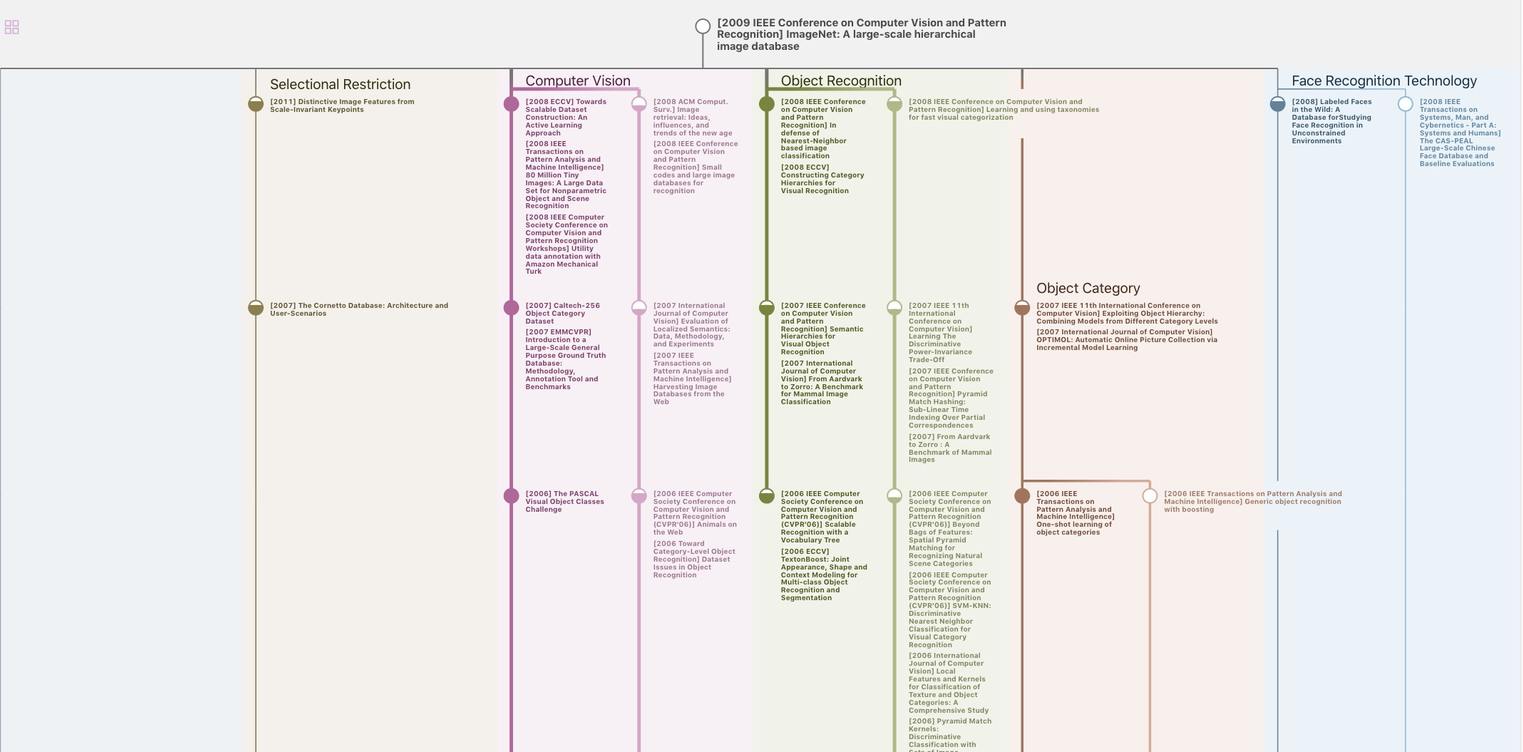
生成溯源树,研究论文发展脉络
Chat Paper
正在生成论文摘要