Real-time Crowd Tracking using Parameter Optimized Mixture of Motion Models.
CoRR(2014)
摘要
We present a novel, real-time algorithm to track the trajectory of each pedestrian in moderately dense crowded scenes. Our formulation is based on an adaptive particle-filtering scheme that uses a combination of various multi-agent heterogeneous pedestrian simulation models. We automatically compute the optimal parameters for each of these different models based on prior tracked data and use the best model as motion prior for our particle-filter based tracking algorithm. We also use our "mixture of motion models" for adaptive particle selection and accelerate the performance of the online tracking algorithm. The motion model parameter estimation is formulated as an optimization problem, and we use an approach that solves this combinatorial optimization problem in a model independent manner and hence scalable to any multi-agent pedestrian motion model. We evaluate the performance of our approach on different crowd video datasets and highlight the improvement in accuracy over homogeneous motion models and a baseline mean-shift based tracker. In practice, our formulation can compute trajectories of tens of pedestrians on a multi-core desktop CPU in in real time and offer higher accuracy as compared to prior real time pedestrian tracking algorithms.
更多查看译文
AI 理解论文
溯源树
样例
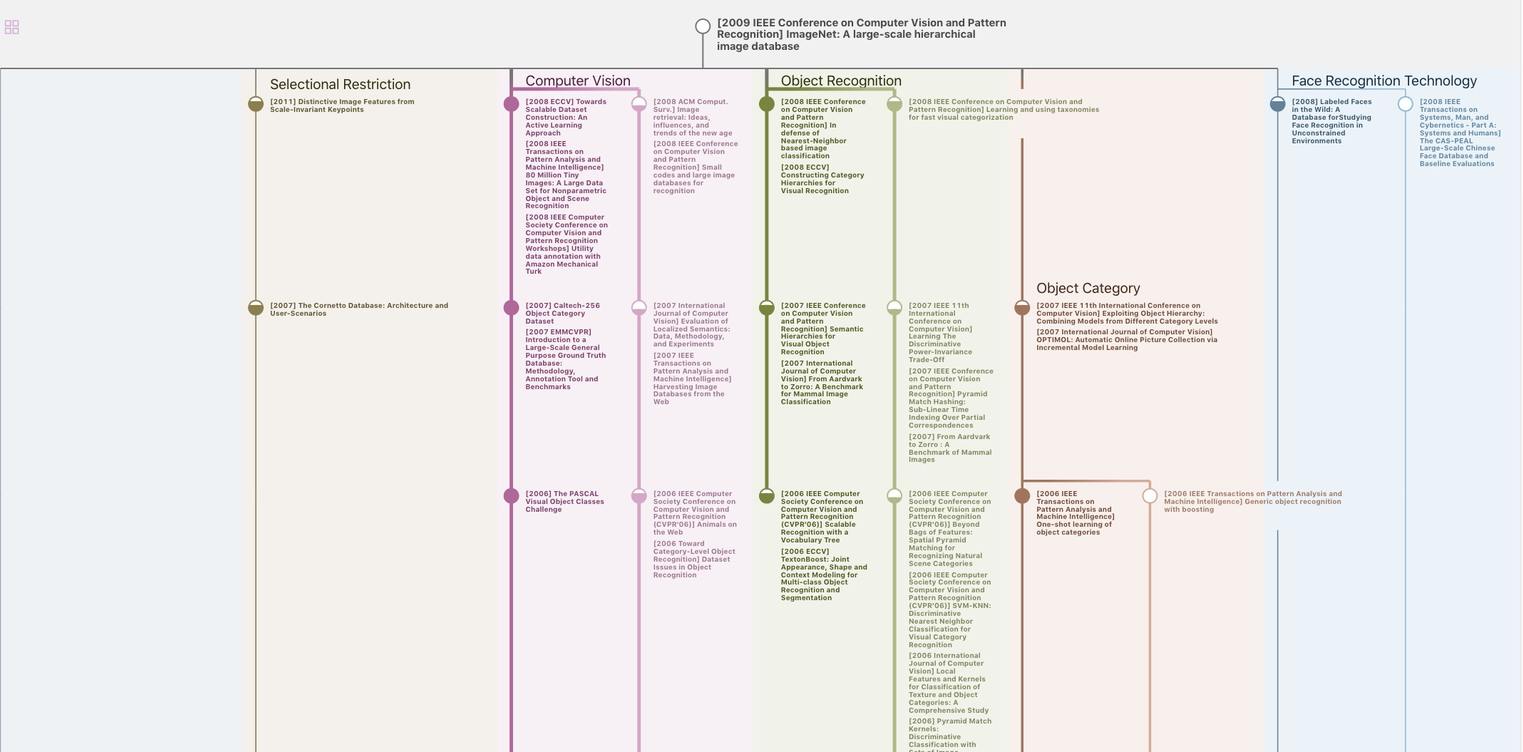
生成溯源树,研究论文发展脉络
Chat Paper
正在生成论文摘要