Towards a Better Understanding of the Local Attractor in Particle Swarm Optimization: Speed and Solution Quality.
ADAPTIVE AND INTELLIGENT SYSTEMS, ICAIS 2014(2014)
摘要
Particle Swarm Optimization (PSO) is a popular nature-inspired meta-heuristic for solving continuous optimization problems. Although this technique is widely used, the understanding of the mechanisms that make swarms so successful is still limited. We present the first substantial experimental investigation of the influence of the local attractor on the quality of exploration and exploitation. We compare in detail classical PSO with the social-only variant where local attractors are ignored. To measure the exploration capabilities, we determine how frequently both variants return results in the neighborhood of the global optimum. We measure the quality of exploitation by considering only function values from runs that reached a search point sufficiently close to the global optimum and then comparing in how many digits such values still deviate from the global minimum value. It turns out that the local attractor significantly improves the exploration, but sometimes reduces the quality of the exploitation. The effects mentioned can also be observed by measuring the potential of the swarm.
更多查看译文
关键词
Particle Swarm Optimiza, Global Optimum, Particle Swarm, Local Optimum, Global Attractor
AI 理解论文
溯源树
样例
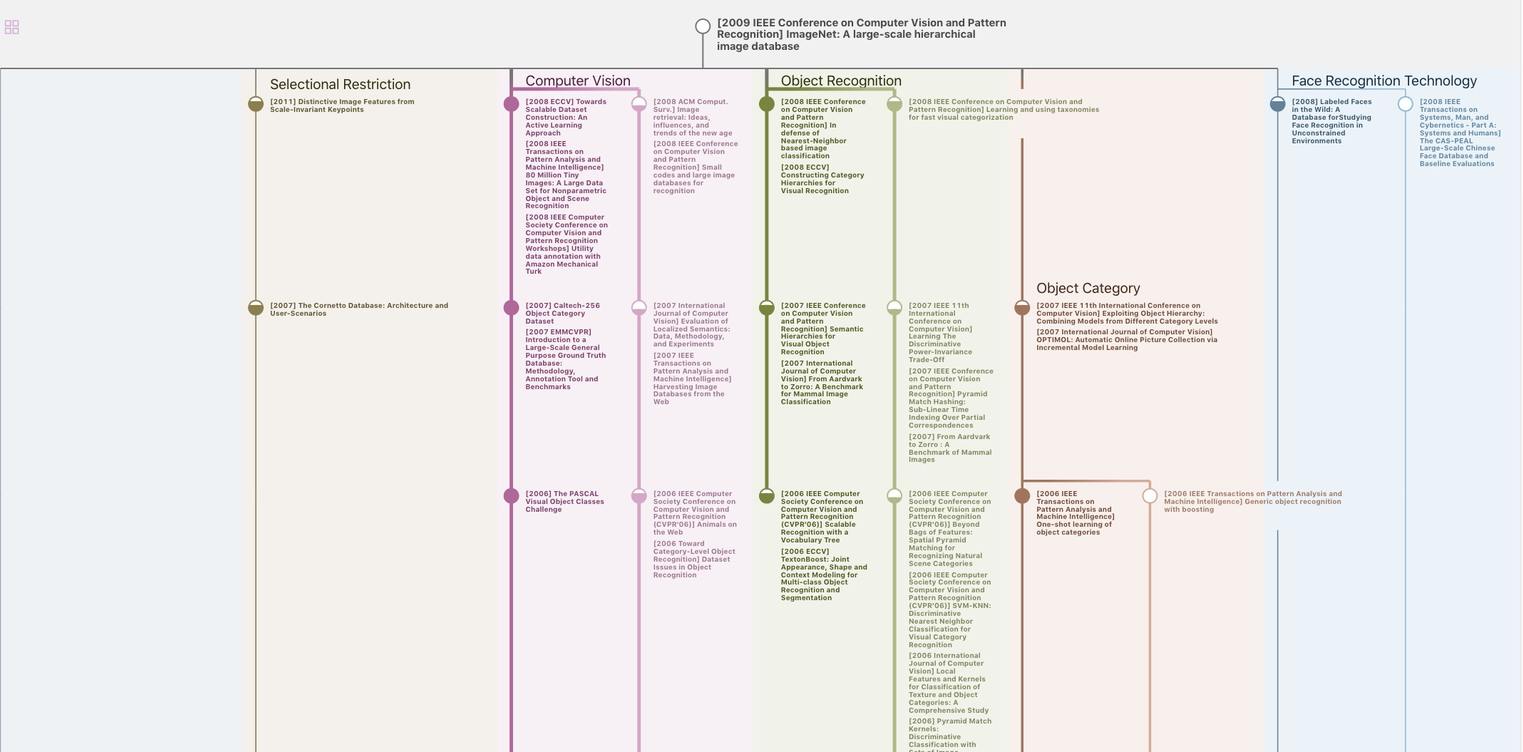
生成溯源树,研究论文发展脉络
Chat Paper
正在生成论文摘要