Multiple Instance Reinforcement Learning for Efficient Weakly-Supervised Detection in Images.
CoRR(2014)
摘要
State-of-the-art visual recognition and detection systems increasingly rely on large amounts of training data and complex classifiers. Therefore it becomes increasingly expensive both to manually annotate datasets and to keep running times at levels acceptable for practical applications. In this paper, we propose two solutions to address these issues. First, we introduce a weakly supervised, segmentation-based approach to learn accurate detectors and image classifiers from weak supervisory signals that provide only approximate constraints on target localization. We illustrate our system on the problem of action detection in static images (Pascal VOC Actions 2012), using human visual search patterns as our training signal. Second, inspired from the saccade-and-fixate operating principle of the human visual system, we use reinforcement learning techniques to train efficient search models for detection. Our sequential method is weakly supervised and general (it does not require eye movements), finds optimal search strategies for any given detection confidence function and achieves performance similar to exhaustive sliding window search at a fraction of its computational cost.
更多查看译文
AI 理解论文
溯源树
样例
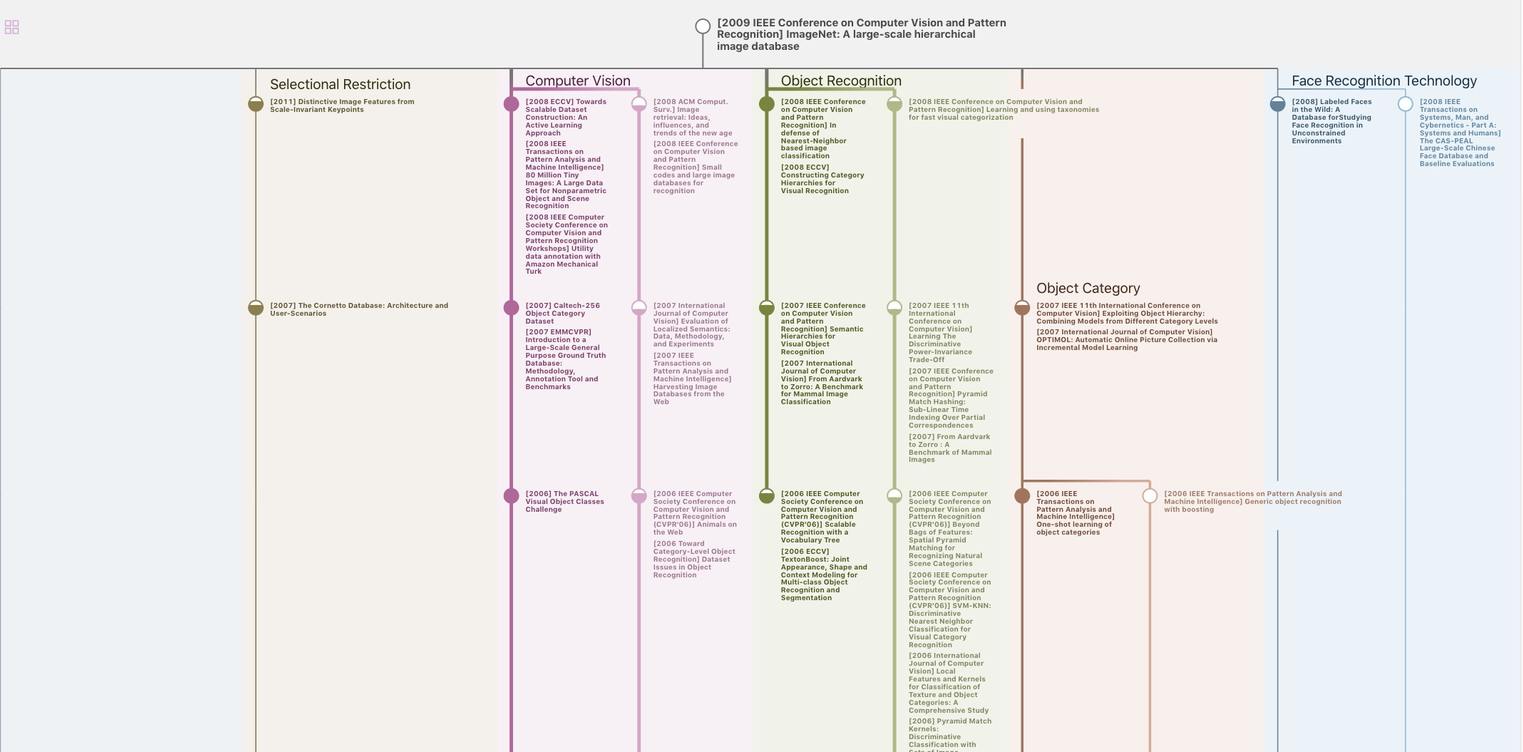
生成溯源树,研究论文发展脉络
Chat Paper
正在生成论文摘要