Max-Margin-Based Discriminative Feature Learning.
IEEE Transactions on Neural Networks and Learning Systems(2016)
摘要
In this brief, we propose a new max-margin-based discriminative feature learning method. In particular, we aim at learning a low-dimensional feature representation, so as to maximize the global margin of the data and make the samples from the same class as close as possible. In order to enhance the robustness to noise, we leverage a regularization term to make the transformation matrix sparse in rows. In addition, we further learn and leverage the correlations among multiple categories for assisting in learning discriminative features. The experimental results demonstrate the power of the proposed method against the related state-of-the-art methods.
更多查看译文
关键词
Correlation,Support vector machines,Linear programming,Learning systems,Covariance matrices,Data visualization,Manifolds
AI 理解论文
溯源树
样例
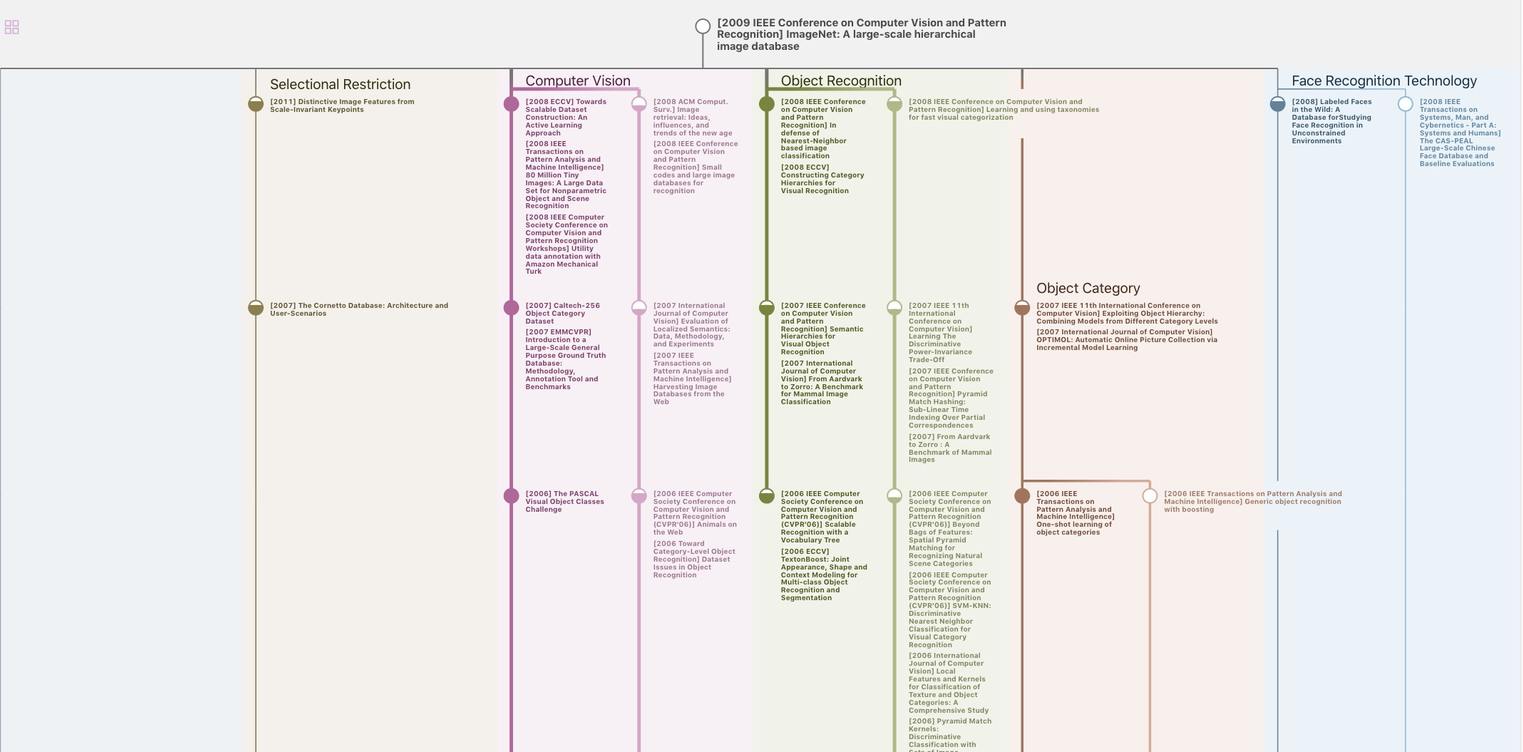
生成溯源树,研究论文发展脉络
Chat Paper
正在生成论文摘要